As computing power continues to advance, the amount of digital data being generated has seen a massive increase. This data holds great potential for both computer scientists and business leaders, as it can provide deep insights that can help improve products and drive decision-making. Machine learning (ML), a subset of artificial intelligence (AI), is a crucial tool in unlocking the value of this data. ML engineers use statistical methods to analyze and find patterns in massive datasets, enabling businesses to make informed decisions and improve application design and testing.
According to the IBM Global AI Adoption Index 2022, 35% of companies are currently using AI in their operations, including ML, and an additional 42% are exploring AI. As ML becomes more integrated into daily business operations, there is a growing need for faster and more efficient ways of managing ML initiatives, increasing model accuracy, and gaining deeper insights. This is where MLOps comes into play.
What is MLOps?
MLOps, short for machine learning operations, is the next stage in the evolution of data analysis and deep learning. It combines automation, continuous integration and continuous delivery/deployment (CI/CD), and machine learning models to streamline the deployment, monitoring, and maintenance of the overall machine learning system. MLOps aims to make machine learning more efficient by using machine learning itself.
Origins of the MLOps Process
MLOps emerged as a response to the slow and difficult scaling of ML lifecycle management for business applications. The term was coined in a 2015 research paper titled “Hidden Technical Debts in the Machine Learning System,” which highlighted common challenges faced when using machine learning in real-world applications. The paper proposed the creation of a systematic MLOps process that borrowed concepts from the CI/CD methodology used in DevOps, creating an assembly line-like approach for each step of the machine learning workflow.
How MLOps Works
The MLOps process involves several steps that work in an iterative and collaborative manner to optimize machine learning models:
- Data preparation and sharing: ML teams prepare and refine datasets, ensuring data availability across teams.
- Model building and training: ML teams use automation and existing ML training models to create and train new models for business applications.
- Model deployment: The trained ML models are made available for deployment and monitoring.
- Model improvement: ML teams use established training data to automate improvements in the model’s performance.
- Automation of the ML lifecycle: ML pipelines are set up to create repeatable workflows, enabling a more efficient process.
The Advantages of MLOps
Implementing MLOps brings several benefits to organizations:
- Increased productivity: The iterative nature of MLOps practices frees up time for IT, engineering, and data science teams to focus on core work.
- Accountability: MLOps processes provide oversight and data validation, ensuring responsible and trustworthy AI implementations.
- Efficiency and cost savings: By streamlining the data science models and enabling simultaneous improvements, MLOps saves time and cost.
- Reduced risk: MLOps enables transparency, faster response to review requests, and compliance with regulations, reducing the risk of delays and wasted efforts.
MLOps Use Cases
MLOps can be applied to various industries and functions:
- IT: MLOps provides greater visibility into operations and centralized deployment and monitoring hubs for AI and machine learning models.
- Data Science: Data scientists can use MLOps for efficiency, oversight, and governance to facilitate regulatory compliance.
- DevOps: Operations teams and data engineers can better manage ML processes by deploying models using familiar programming languages onto modern runtime environments.
MLOps vs. DevOps
MLOps and DevOps share similarities, but MLOps is specifically tailored for machine learning projects. While DevOps focuses on combining and automating software development and IT operations, MLOps applies similar principles to ML workflows. The goal of both is to enable more efficient and high-quality production processes.
MLOps vs. AIOps
AIOps (Artificial Intelligence for IT Operations) utilizes AI capabilities to automate and streamline operational workflows. It focuses on optimizing IT operations, while MLOps is centered around building and training ML models for various applications.
MLOps and IBM
IBM’s Watsonx.ai offers a platform that empowers data scientists, developers, and analysts to build, run, and manage AI models. With MLOps, AI builders can simplify model production, deploy models from any tool, and enable automatic model retraining.
If you’re looking to scale the impact of AI across your business, explore watsonx.ai, IBM’s next-generation enterprise studio for AI builders.
Summary
MLOps (Machine Learning Operations) is the evolution of data analysis and deep learning, aiming to make machine learning more efficient by using automation, continuous integration and continuous delivery/deployment (CI/CD), and machine learning models. It streamlines the deployment, monitoring, and maintenance of machine learning systems. MLOps emerged from the realization that managing the machine learning lifecycle was slow and challenging, and it builds upon DevOps principles to create a more scalable and streamlined process. By implementing MLOps, organizations can increase productivity, accountability, efficiency, cost savings, and reduce risks associated with machine learning models. MLOps is applicable to various industries and functions, and it differs from DevOps and AIOps in its specific focus on machine learning projects. IBM’s Watsonx.ai offers a platform to simplify and automate MLOps processes, enabling AI builders to deploy and manage AI models effectively.
FAQs
What is MLOps?
MLOps stands for Machine Learning Operations. It is a process that uses automation, continuous integration and continuous delivery/deployment (CI/CD), and machine learning models to streamline the deployment, monitoring, and maintenance of machine learning systems.
Why is MLOps important?
MLOps is crucial because it makes machine learning more efficient and scalable. It enables organizations to manage ML initiatives, increase model accuracy, and gain deeper insights. By implementing MLOps, businesses can improve productivity, accountability, efficiency, and cost savings.
What are the benefits of MLOps?
The benefits of MLOps include increased productivity, accountability, efficiency, cost savings, and reduced risk. MLOps enables organizations to streamline their machine learning processes, ensure responsible and trustworthy AI implementations, and save time and costs associated with data science models.
How does MLOps differ from DevOps?
MLOps and DevOps share similarities in their approach to process automation and collaboration. However, MLOps is specifically tailored for machine learning projects, while DevOps focuses on combining and automating software development and IT operations in general.
What is AIOps?
AIOps stands for Artificial Intelligence for IT Operations. AIOps uses AI capabilities, such as natural language processing and machine learning models, to automate and streamline operational workflows. It is primarily focused on optimizing IT operations.
How does MLOps help in data science?
MLOps helps data scientists by providing them with a more efficient and standardized process for building machine learning models. It offers oversight, governance, and compliance facilitation, allowing data scientists to focus on their core work and achieve better outcomes.
More in this category ...
Ripple companions with SBI Group and HashKey DX for XRPL answers in Japan

April sees $25M in exploits and scams, marking historic low ― Certik

MSTR, COIN, RIOT and different crypto shares down as Bitcoin dips

EigenLayer publicizes token release and airdrop for the group

VeloxCon 2024: Innovation in knowledge control
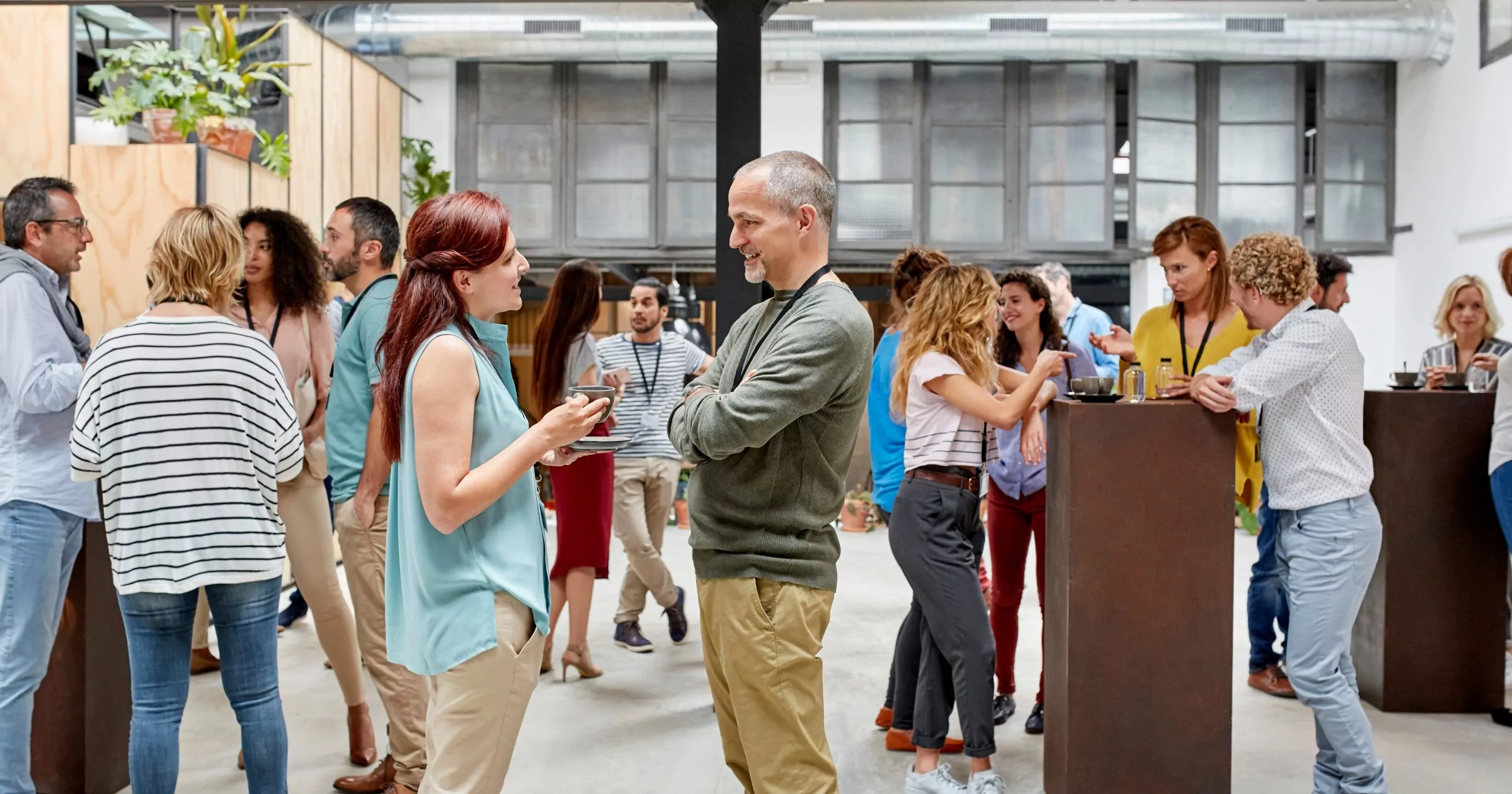
Successful Beta Service release of SOMESING, ‘My Hand-Carry Studio Karaoke App’

Dogwifhat (WIF) large pump on Bybit after record reasons marketplace frenzy

How fintech innovation is riding virtual transformation for communities around the globe

Wasabi Wallet developer bars U.S. customers amidst regulatory considerations

Analyst Foresees Peak In Late 2025
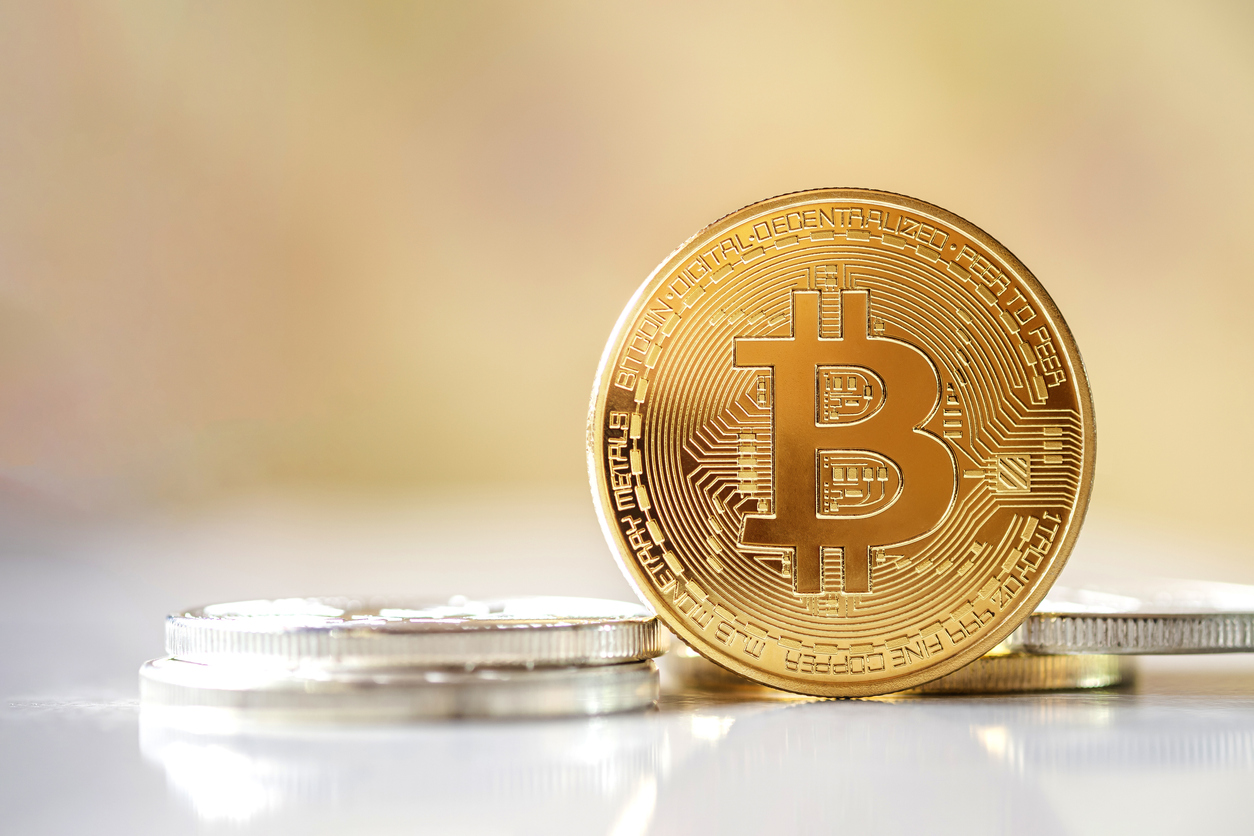
Solo Bitcoin miner wins the three.125 BTC lottery, fixing legitimate block
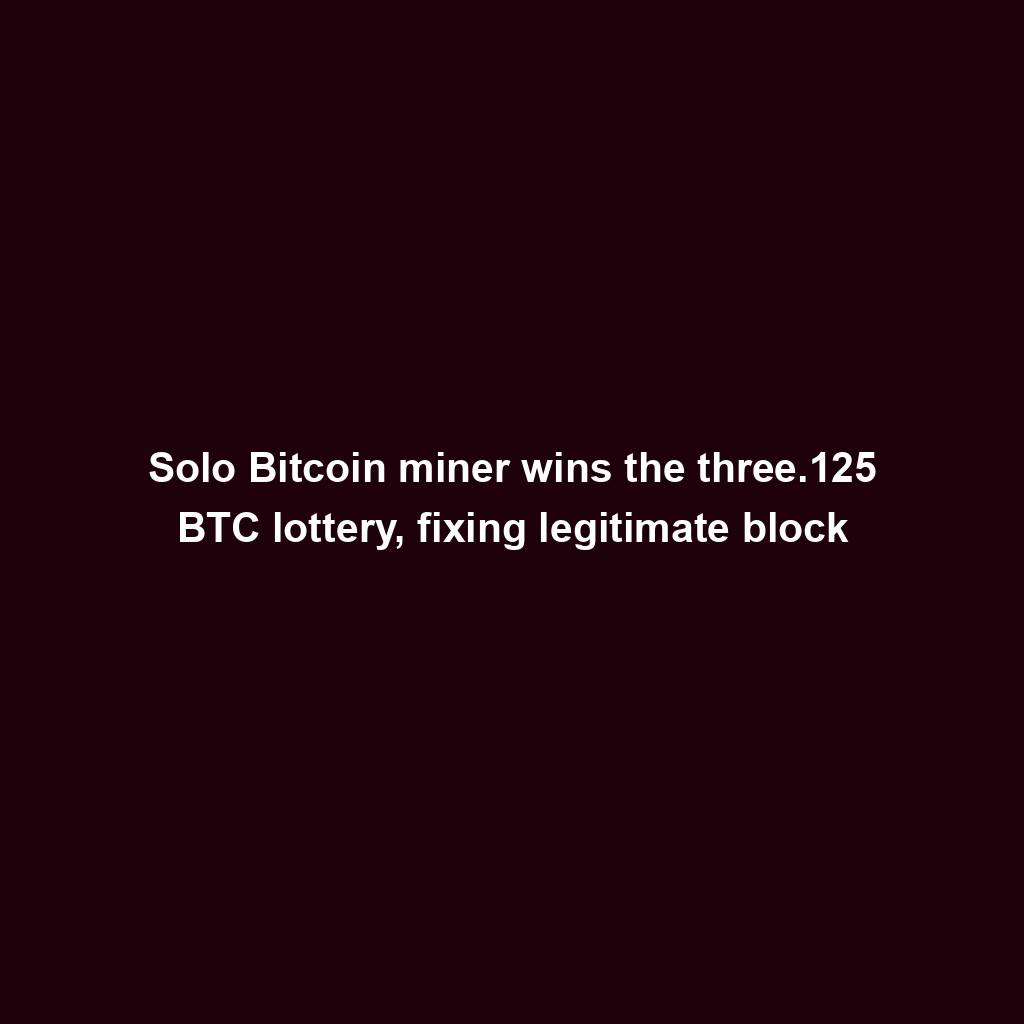
Ace Exchange Suspects Should Get 20-Year Prison Sentences: Prosecutors
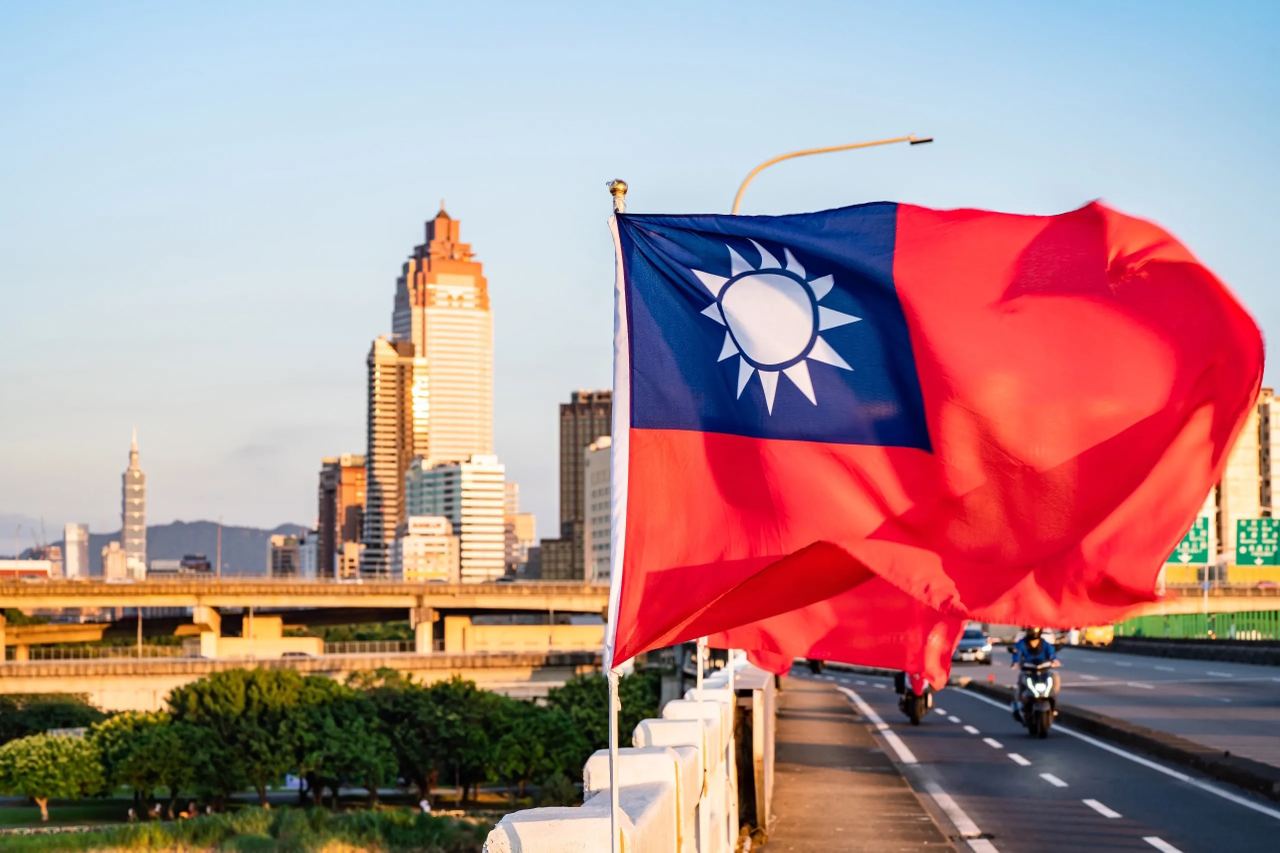
Google Cloud's Web3 portal release sparks debate in crypto trade
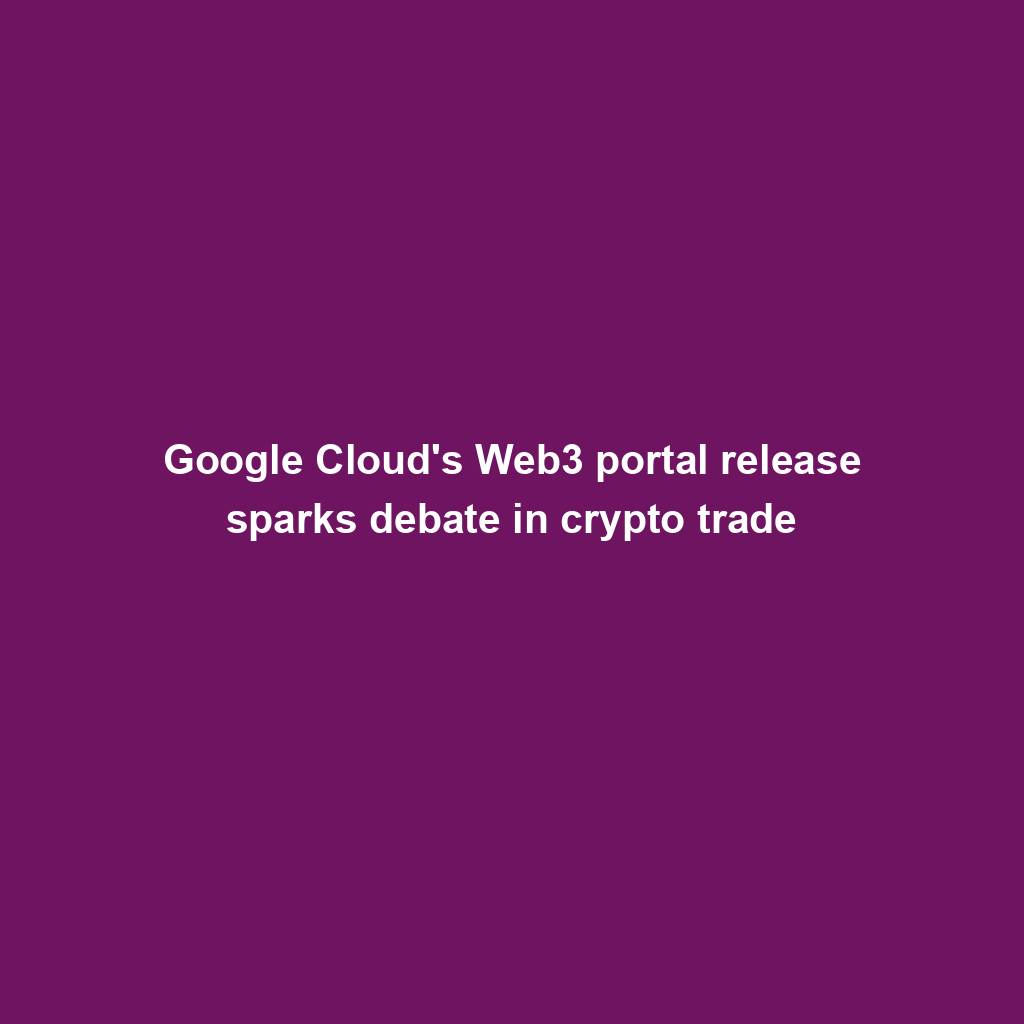
Bitcoin Primed For $77,000 Surge
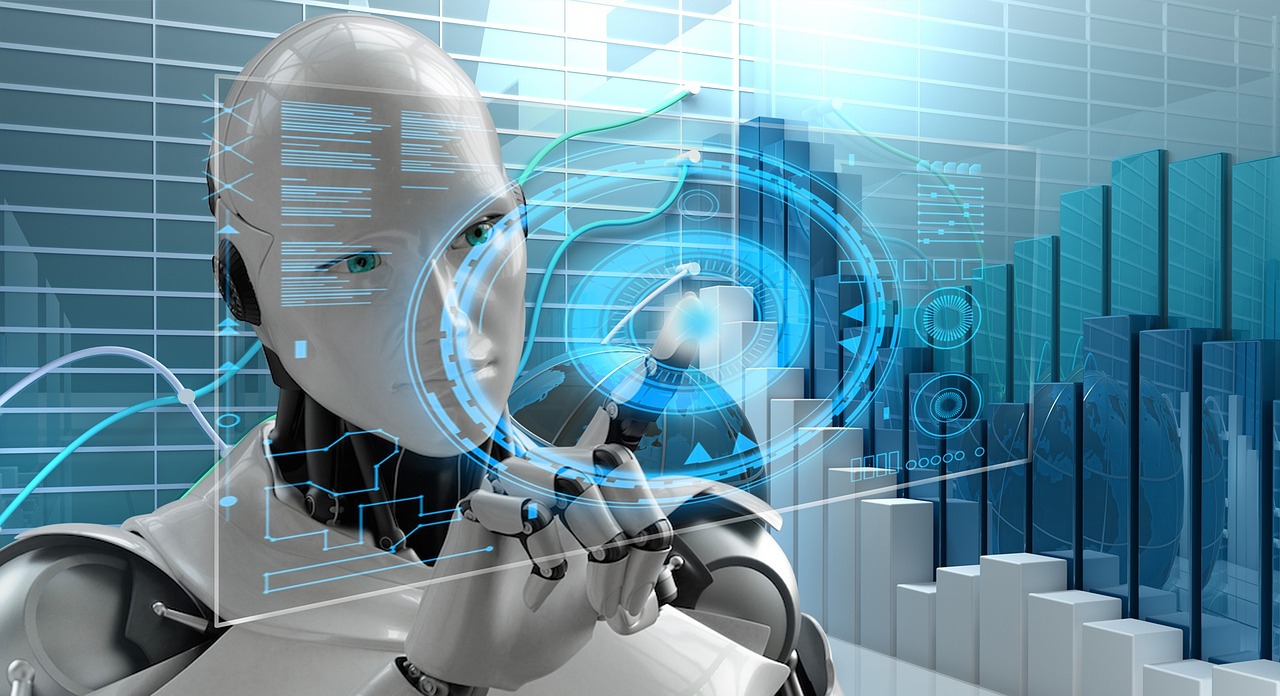
Bitbot’s twelfth presale level nears its finish after elevating $2.87 million
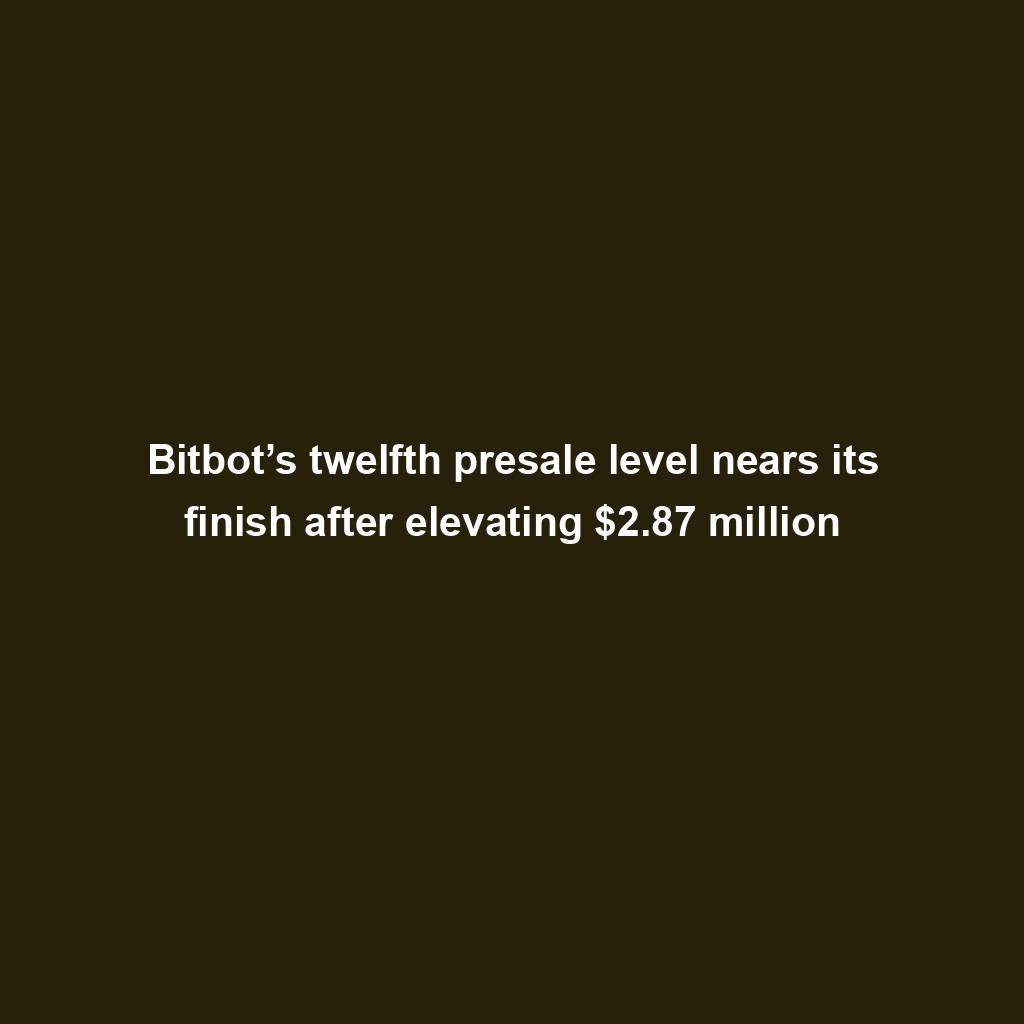
PANDA and MEW bullish momentum cool off: traders shift to new altcoin
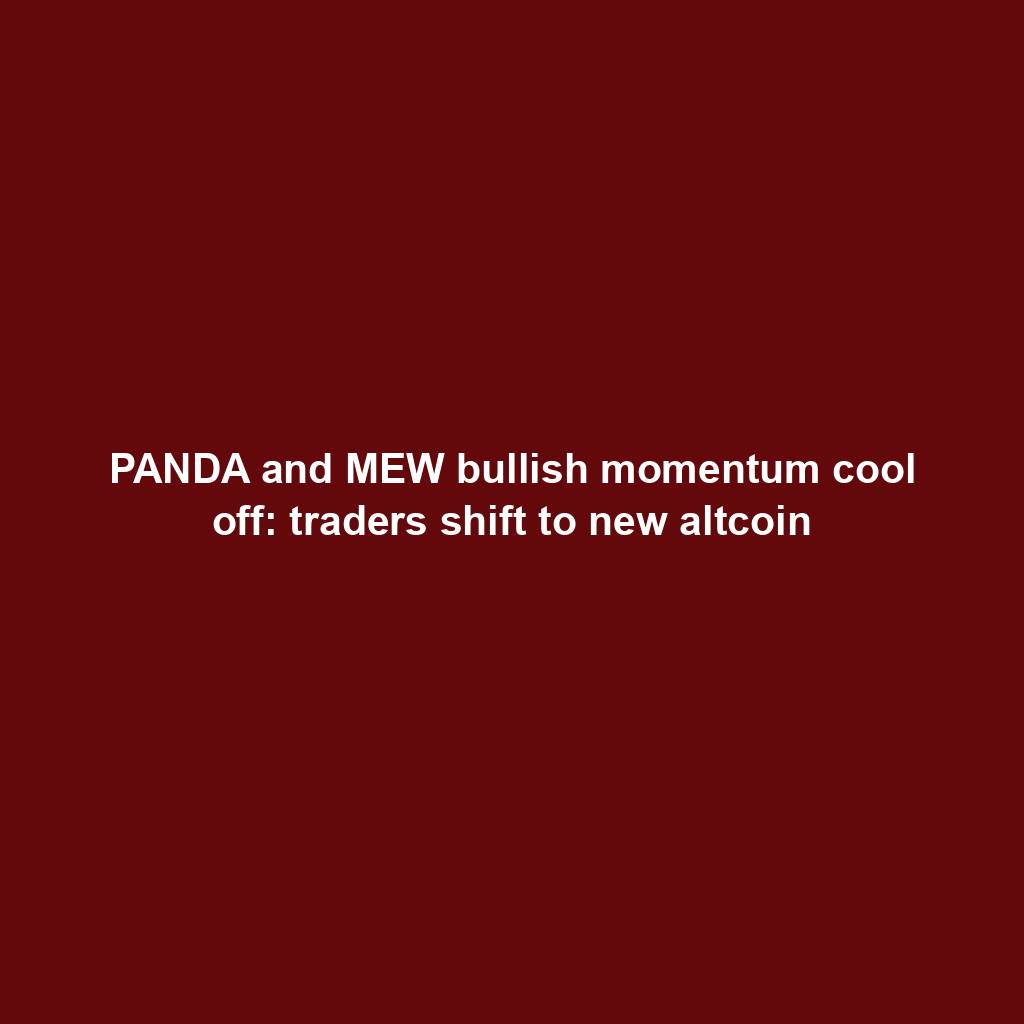
Commerce technique: Ecommerce is useless, lengthy are living ecommerce
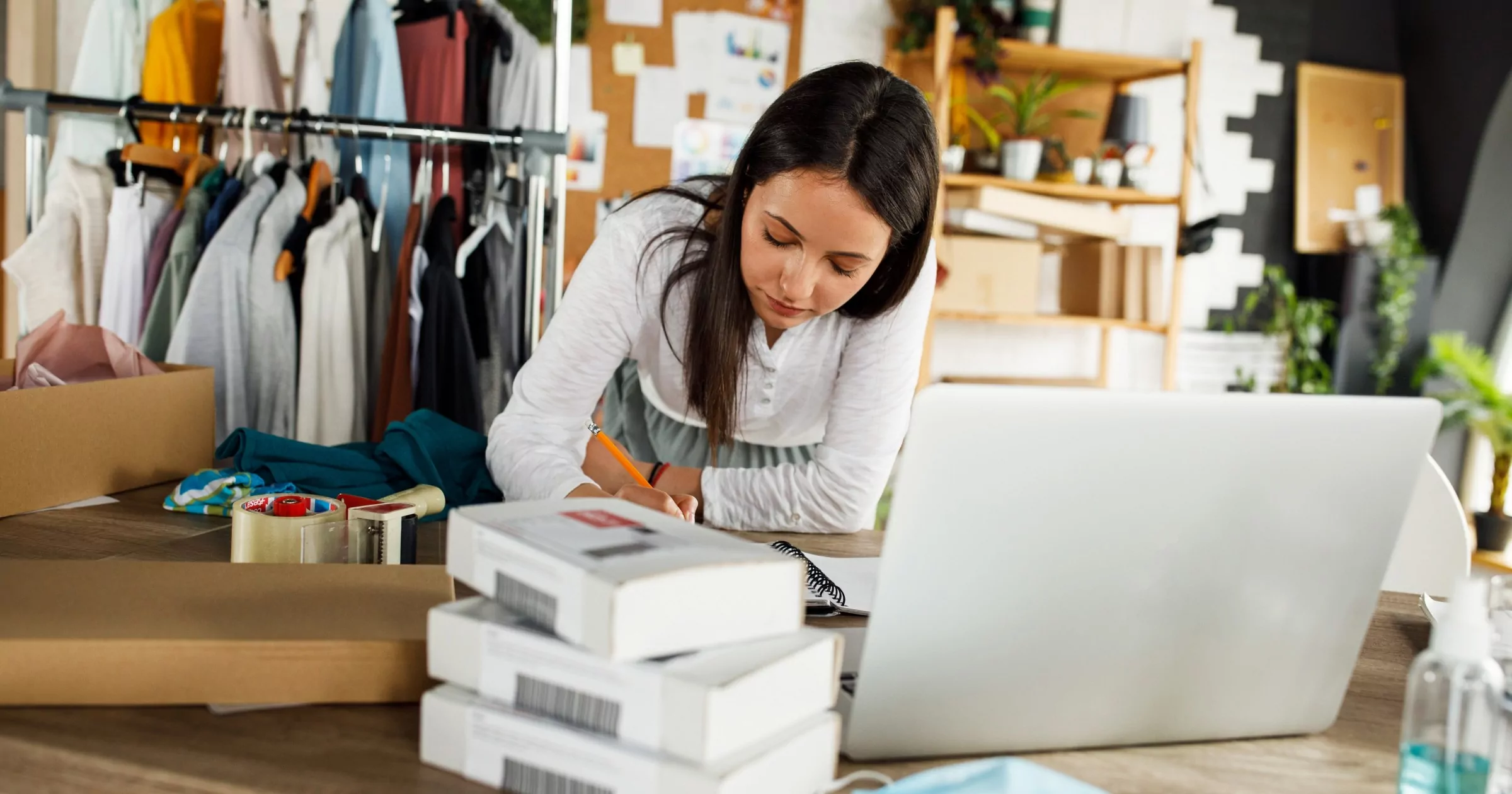
Republic First Bank closed by way of US regulators — crypto neighborhood reacts
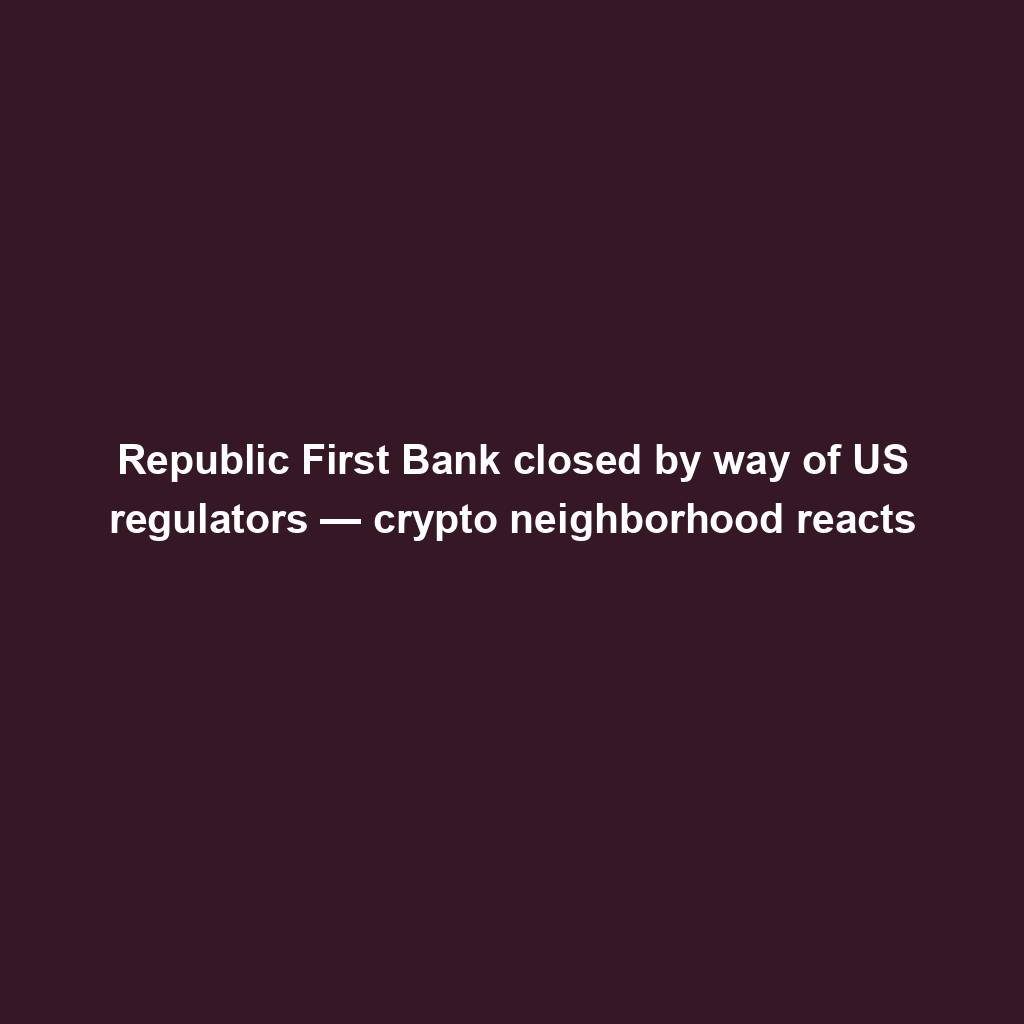
China’s former CBDC leader is beneath executive investigation
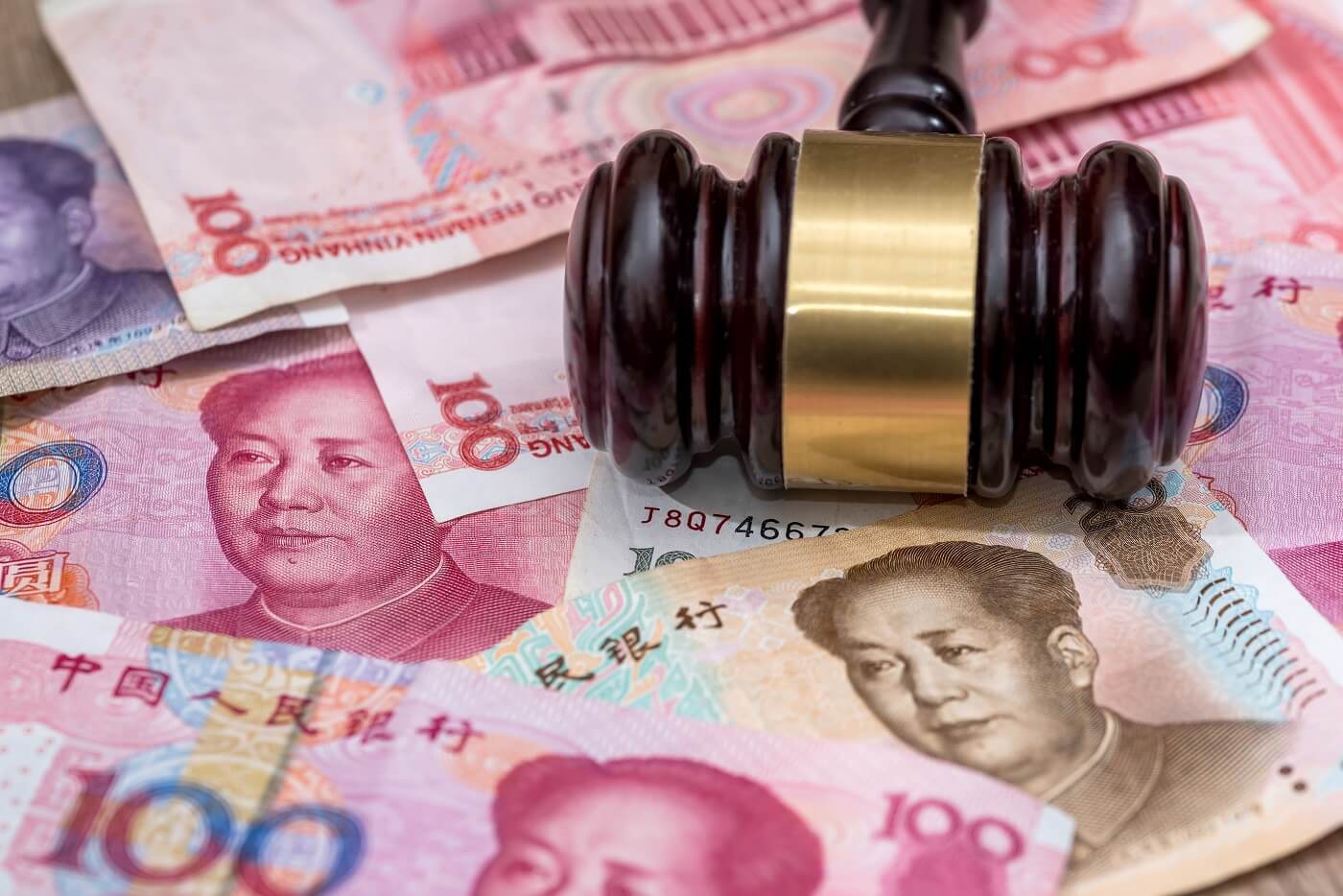
Bigger isn’t all the time higher: How hybrid Computational Intelligence development permits smaller language fashions
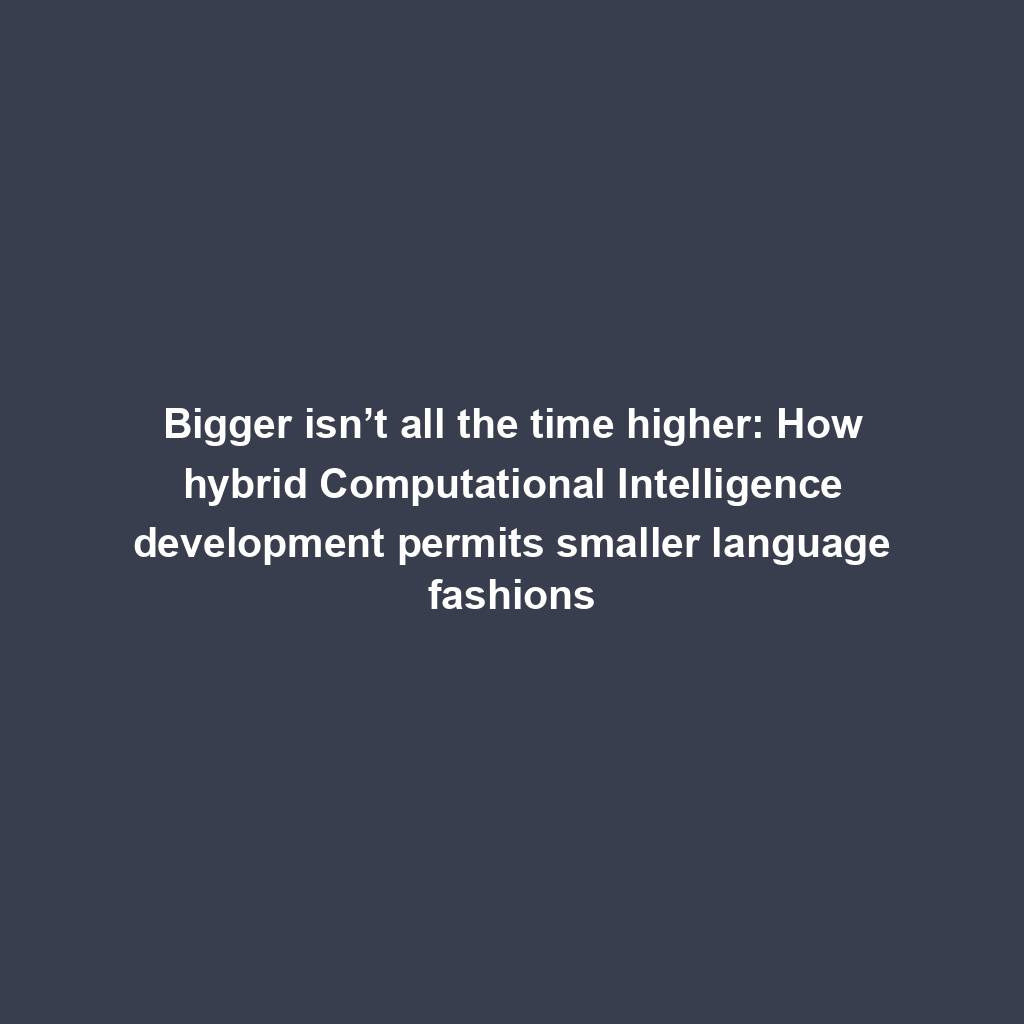
Pantera Capital buys extra Solana (SOL) from FTX
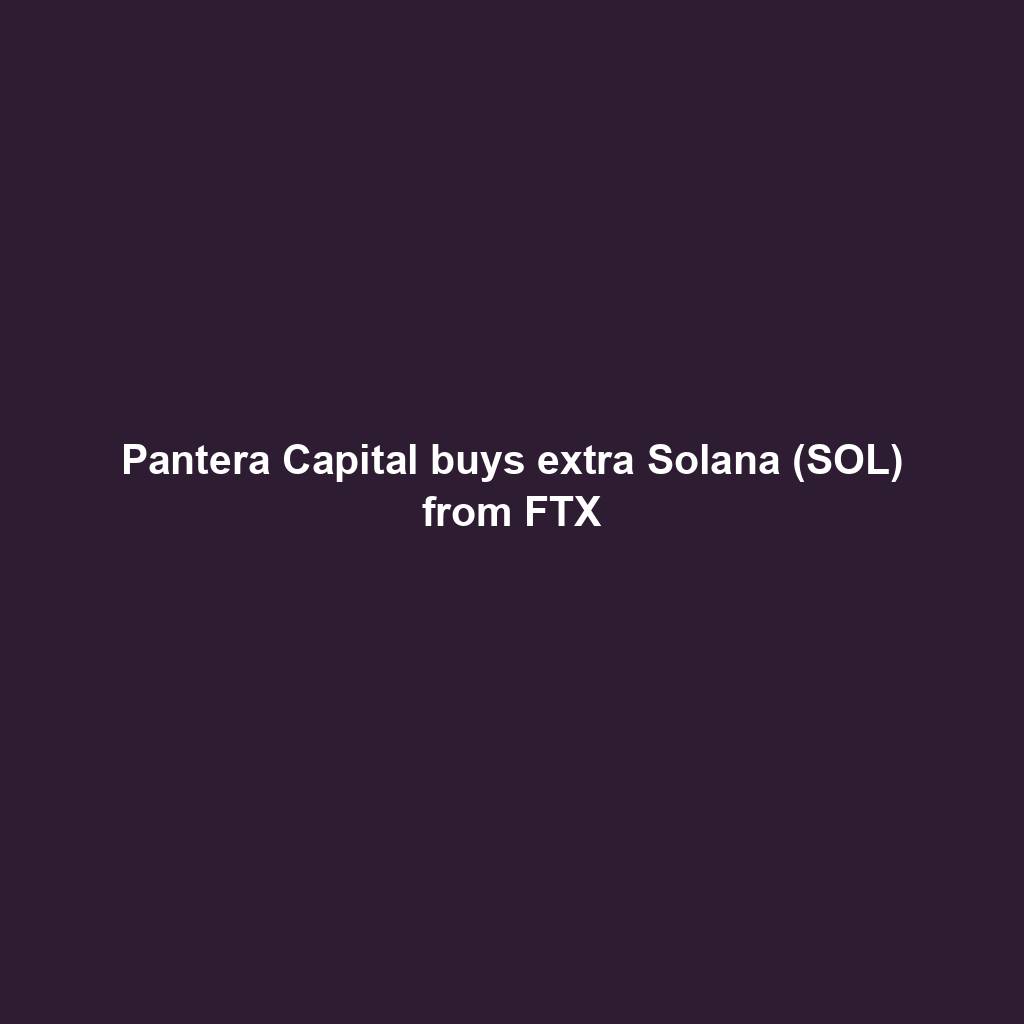
Successful Beta Service release of SOMESING, ‘My Hand-Carry Studio Karaoke App’

SEC sues Bitcoin miner Geosyn Mining for fraud; Bitbot presale nears $3M
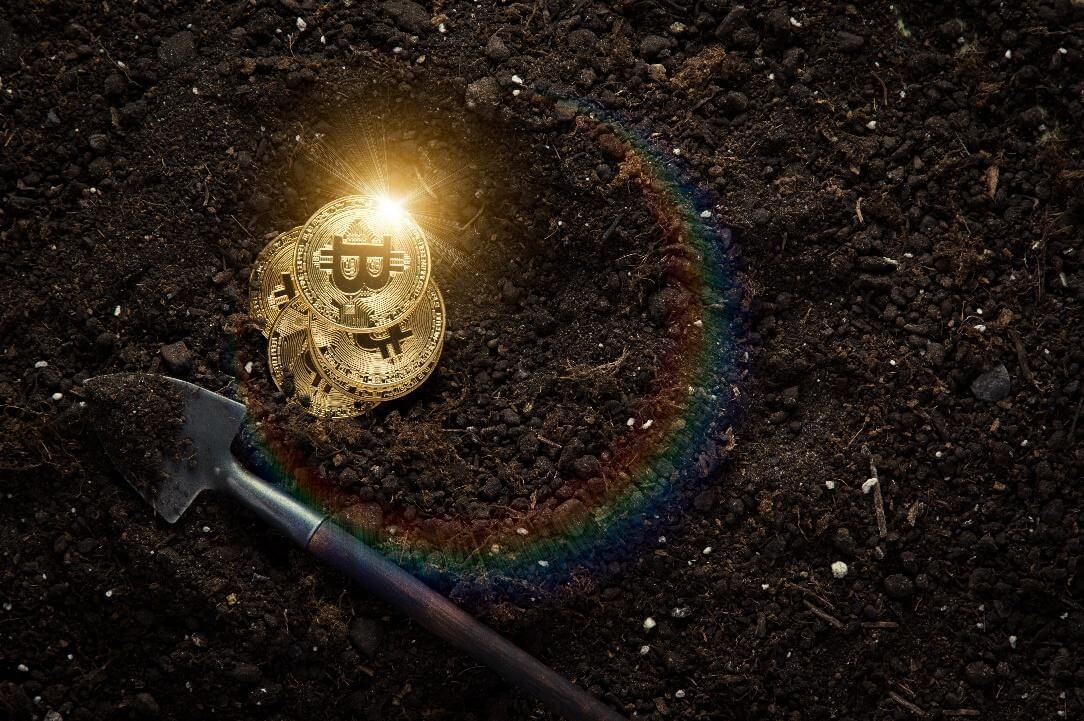
Business procedure reengineering (BPR) examples
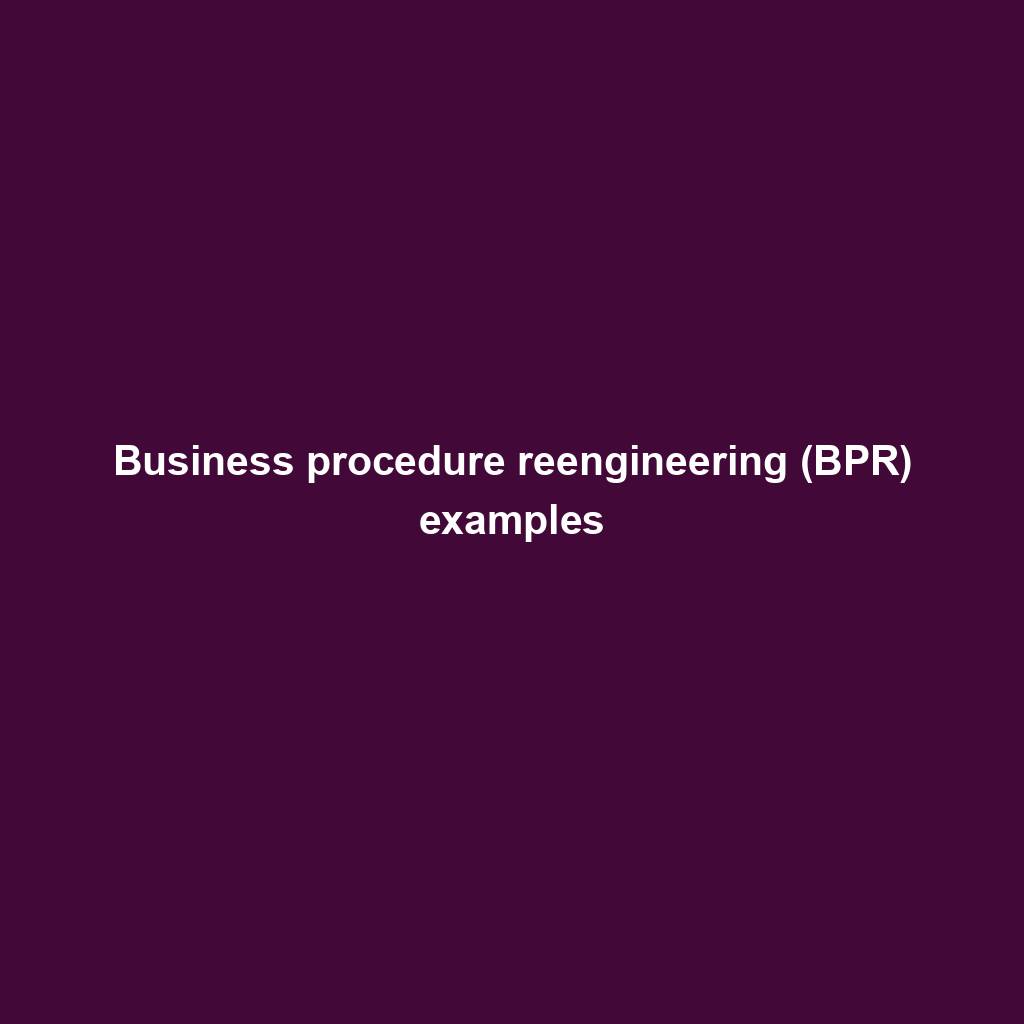
85% Of Altcoins In “Opportunity Zone,” Santiment Reveals
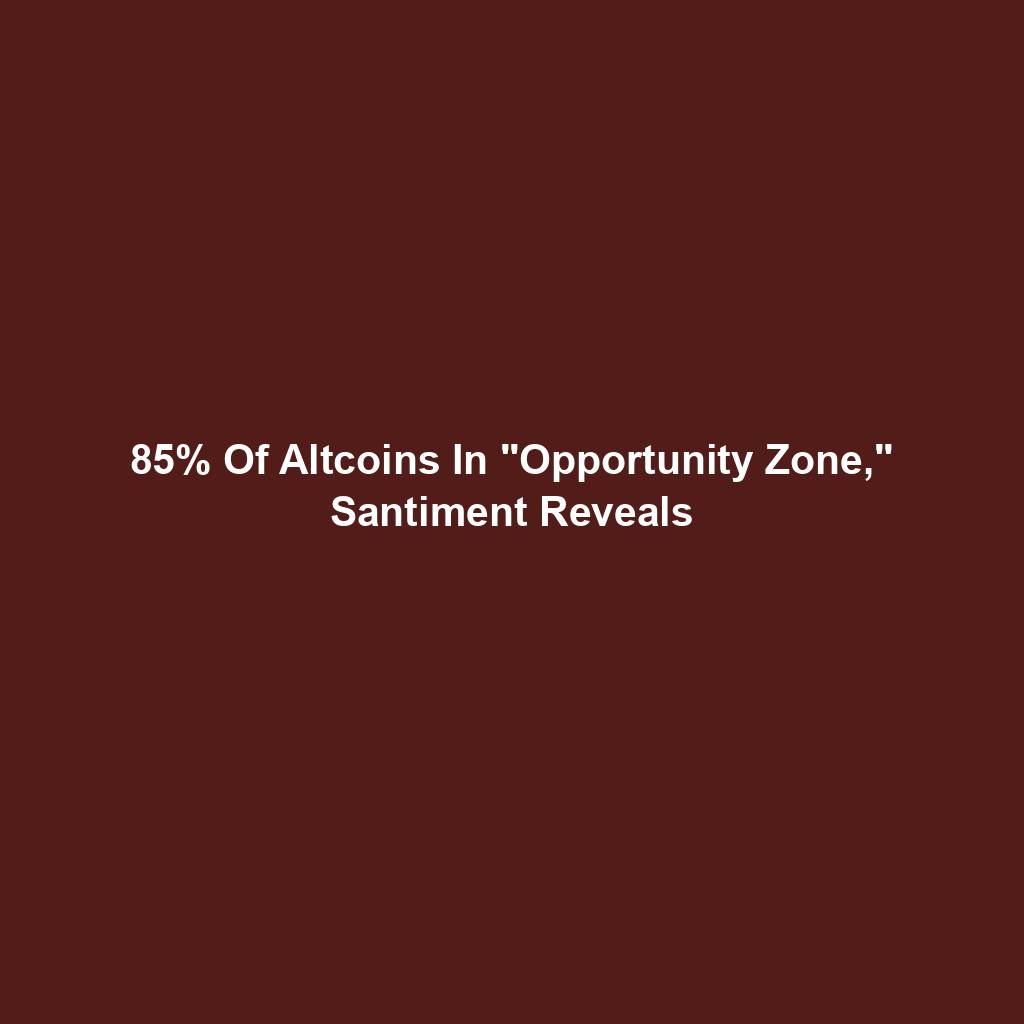
Sam Altman’s Worldcoin eyeing PayPal and OpenAI partnerships
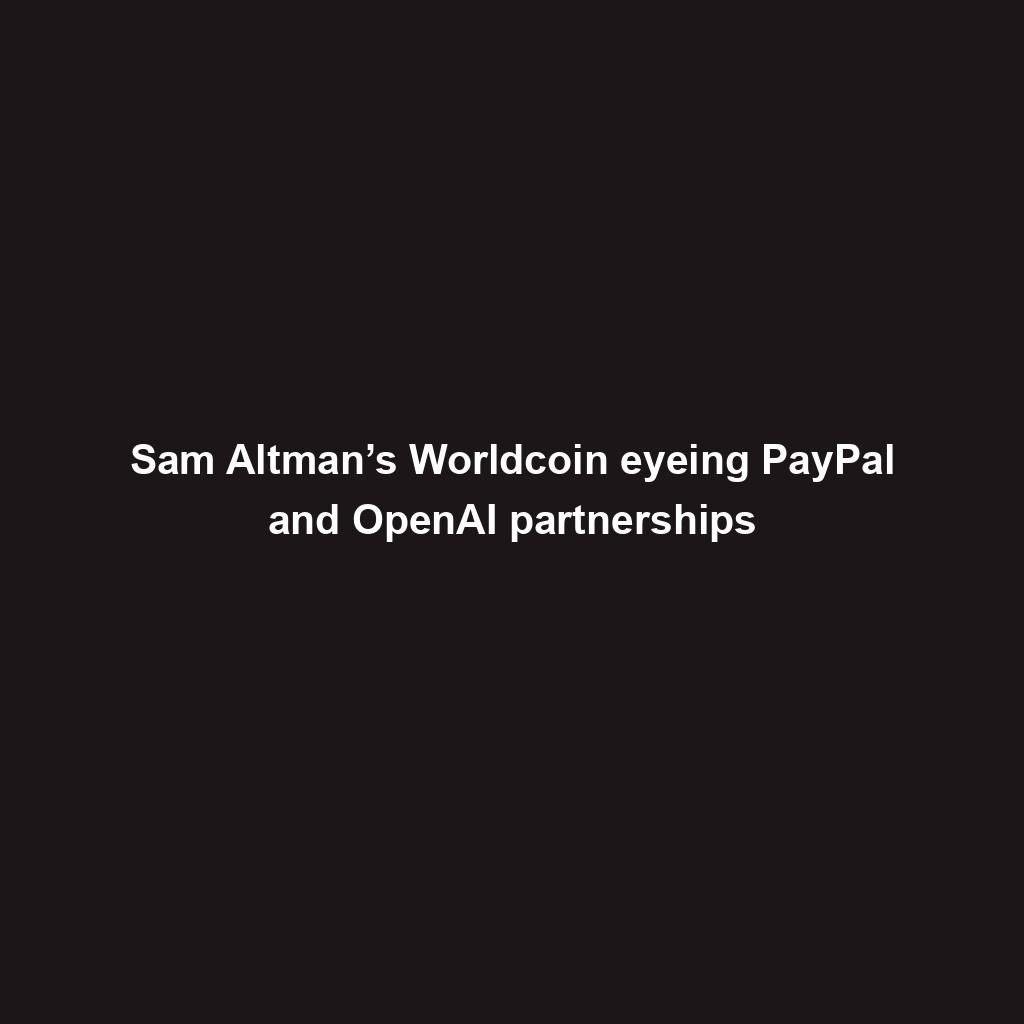
Artificial Intelligence transforms the IT strengthen enjoy
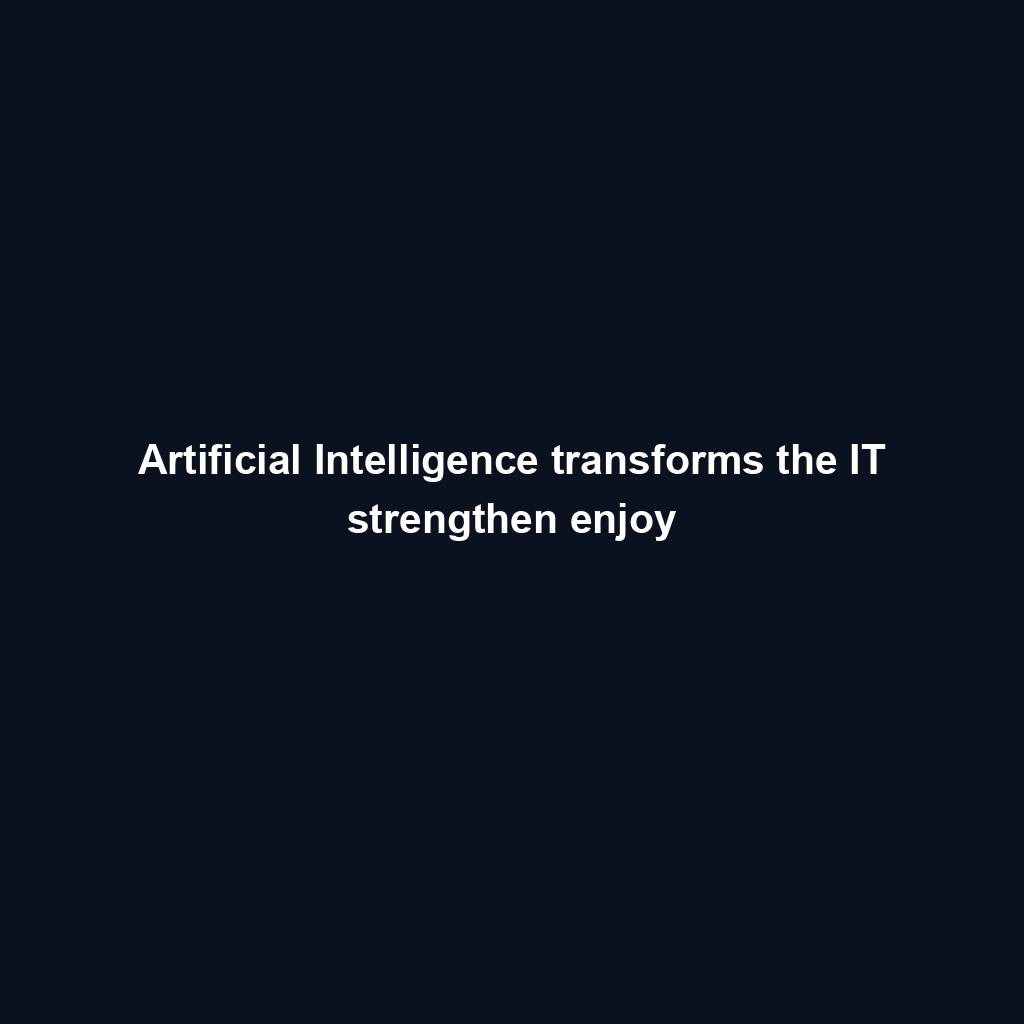
Franklin Templeton tokenizes $380M fund on Polygon and Stellar for P2P transfers
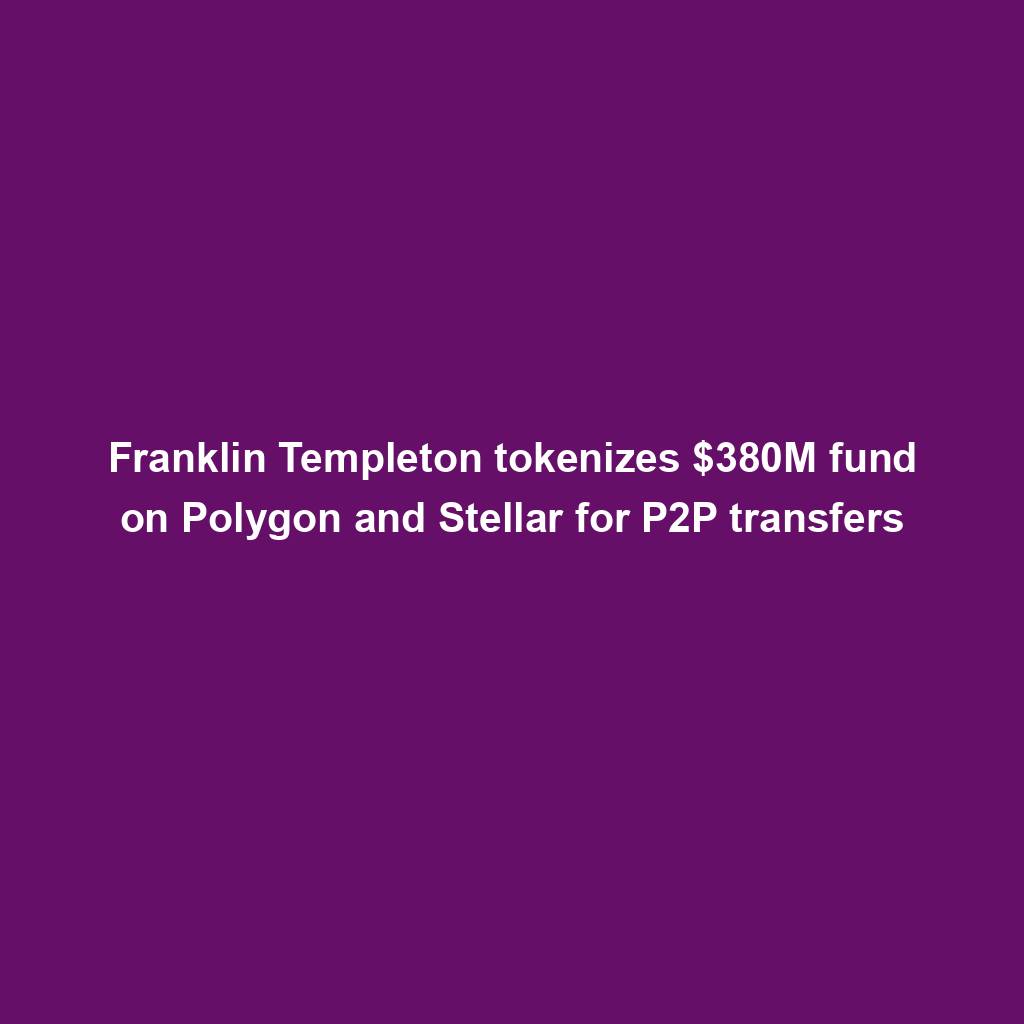
Meta’s letting Xbox, Lenovo, and Asus construct new Quest metaverse {hardware}
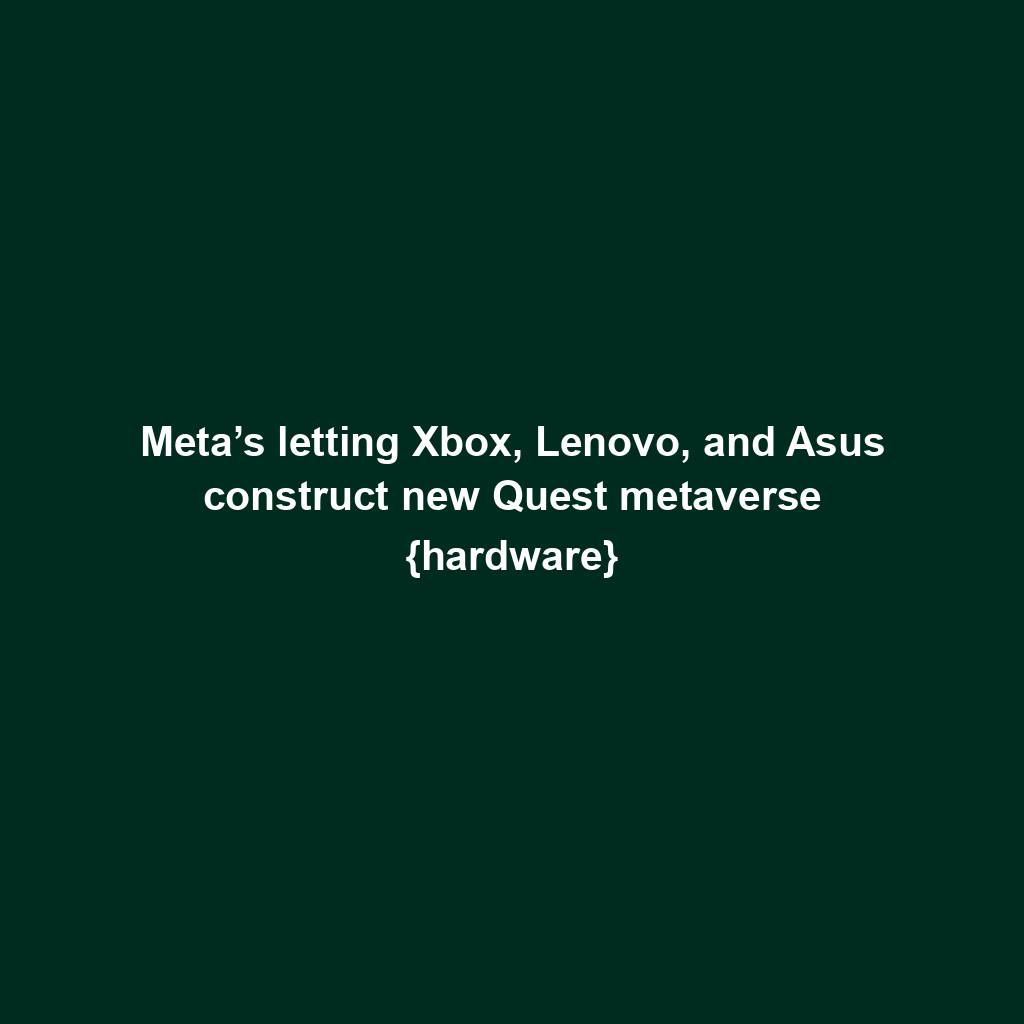
Shiba Inu (SHIB) unveils bold Shibarium plans as Kangamoon steals the display
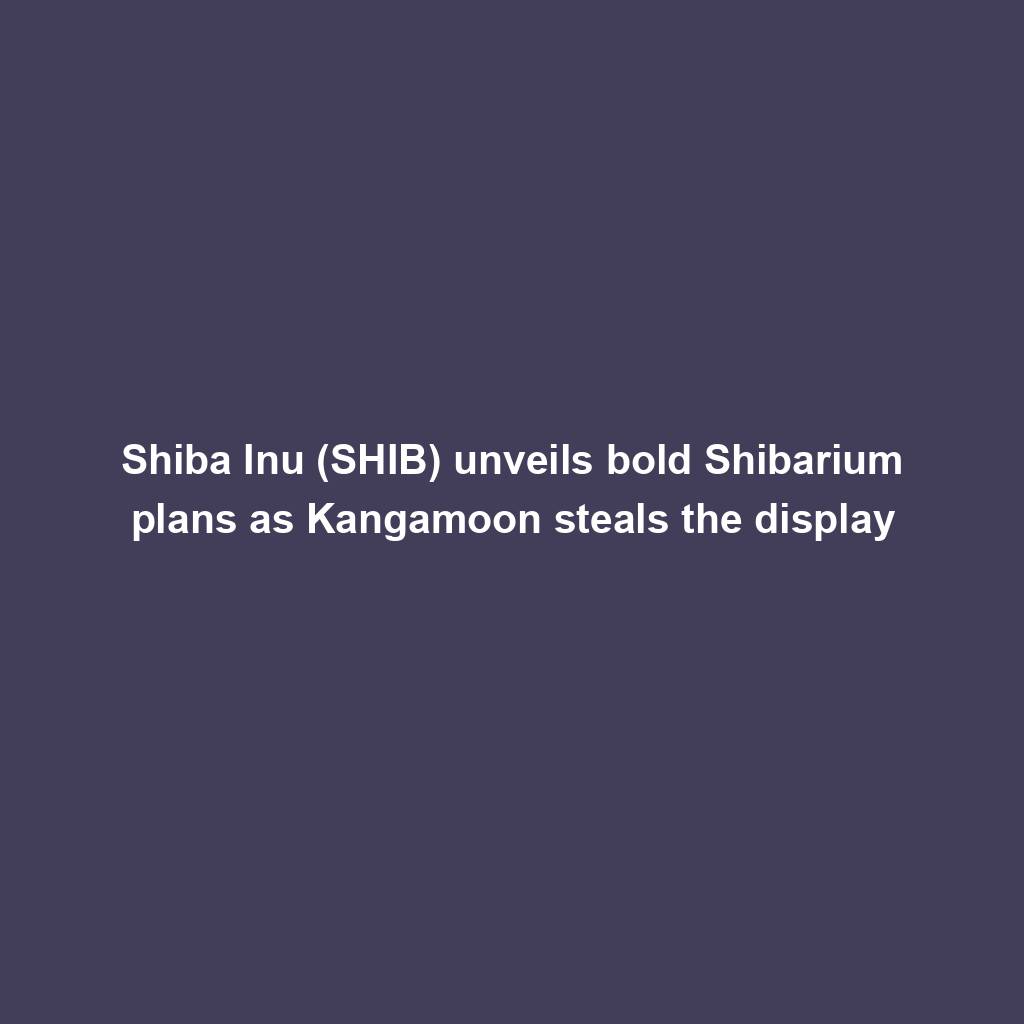