In the world of machine learning (ML) and artificial intelligence (AI), foundational models (FMs) are revolutionizing the way AI is developed and applied. These models, trained on a broad set of unlabeled data, can be adapted to a wide range of tasks and applications. By combining the power of FMs with edge computing, enterprises can run AI workloads for FM fine-tuning and inferencing at the operational edge, enabling faster deployment, near-real-time predictions, and reduced costs.
What are Foundational Models (FMs)?
FMs are AI models trained on large amounts of unlabeled data, enabling them to learn more generally and work across domains and problems. Unlike traditional AI models that specialize in specific tasks within a single domain, FMs serve as a foundation for a multitude of applications. They address the challenges of scaling AI adoption by ingesting vast amounts of unlabeled data and using self-supervised techniques for training, eliminating the need for extensive human labeling and annotation.
How Do Large Language Models (LLMs) Fit into Foundational Models?
Large language models (LLMs) are a class of FMs that consist of neural networks trained on massive amounts of unlabeled data. These models can perform various natural language processing (NLP) tasks and have become integral to AI applications. LLMs learn to understand language in a way similar to humans, making them invaluable in a wide range of industries.
Scaling AI at the Edge
Deploying AI models at the edge, where data is generated and work is performed, allows for near-real-time predictions while maintaining data sovereignty and privacy. However, scaling AI deployments at the edge comes with challenges such as deployment time and cost, as well as day-to-day management of numerous edge locations.
IBM has developed an edge architecture that addresses these challenges by introducing an integrated hardware/software (HW/SW) appliance model. This model enables zero-touch provisioning of software, continuous monitoring of edge system health, and centralized management of software, security, and configuration updates. The architecture follows a hub-and-spoke deployment configuration, with a central cloud acting as the hub and edge-in-a-box appliances serving as spokes at each edge location.
The Value of FM Fine-Tuning and Inference at the Edge
By pre-training FMs in the cloud and fine-tuning them for specific downstream tasks at the edge, enterprises can optimize the use of compute resources and reduce operational costs. Fine-tuning requires fewer labeled data samples and can be performed using a few GPUs at the enterprise edge, allowing sensitive data to stay within the operational environment. Serving the fine-tuned AI model at the edge also reduces latency and data transfer costs.
To demonstrate the value of FM fine-tuning and inference at the edge, IBM deployed a vision-transformer-based FM for civil infrastructure on a three-node edge cluster. This deployment showcased the reduction in time-to-insight and cost associated with defect detection using drone imagery inputs.
Summary
Combining IBM watsonx data and AI platform capabilities with edge-in-a-box appliances empowers enterprises to run AI workloads at the edge, reducing deployment time, enabling near-real-time predictions, and optimizing resource utilization. FM fine-tuning and inference at the edge provide significant advantages in terms of reduced latency, data transfer costs, and improved security. With FMs and edge computing, the possibilities for AI deployments are expanding, opening doors to faster and more efficient AI adoption across industries.
FAQs
What are foundational models (FMs)?
Foundational models (FMs) are AI models that are trained on a broad set of unlabeled data, allowing them to be adapted to various downstream tasks and applications. Unlike traditional AI models, FMs learn more generally and work across domains and problems.
What are large language models (LLMs)?
Large language models (LLMs) are a class of foundational models that consist of neural networks trained on massive amounts of unlabeled data. These models excel in natural language processing (NLP) tasks and have become integral to AI applications.
What is the advantage of running AI workloads at the edge?
Running AI workloads at the edge enables near-real-time predictions while abiding by data sovereignty and privacy requirements. It reduces latency and data transfer costs, allowing for faster and more efficient data analysis and insights.
How does edge-in-a-box architecture facilitate AI deployments at the edge?
The edge-in-a-box architecture provides an integrated hardware/software (HW/SW) appliance model for AI deployments at the edge. It enables zero-touch provisioning, continuous monitoring of edge system health, and centralized management of software, security, and configuration updates, making AI deployments at the edge more scalable and efficient.
How does fine-tuning AI models at the edge reduce operational costs?
Fine-tuning AI models at the edge reduces the time required and data transfer costs associated with inferencing tasks. It allows sensitive data to stay within the operational environment and optimizes resource utilization by utilizing a few GPUs at the enterprise edge instead of extensive cloud compute resources.
More in this category ...
Ripple companions with SBI Group and HashKey DX for XRPL answers in Japan

April sees $25M in exploits and scams, marking historic low ― Certik

MSTR, COIN, RIOT and different crypto shares down as Bitcoin dips

EigenLayer publicizes token release and airdrop for the group

VeloxCon 2024: Innovation in knowledge control
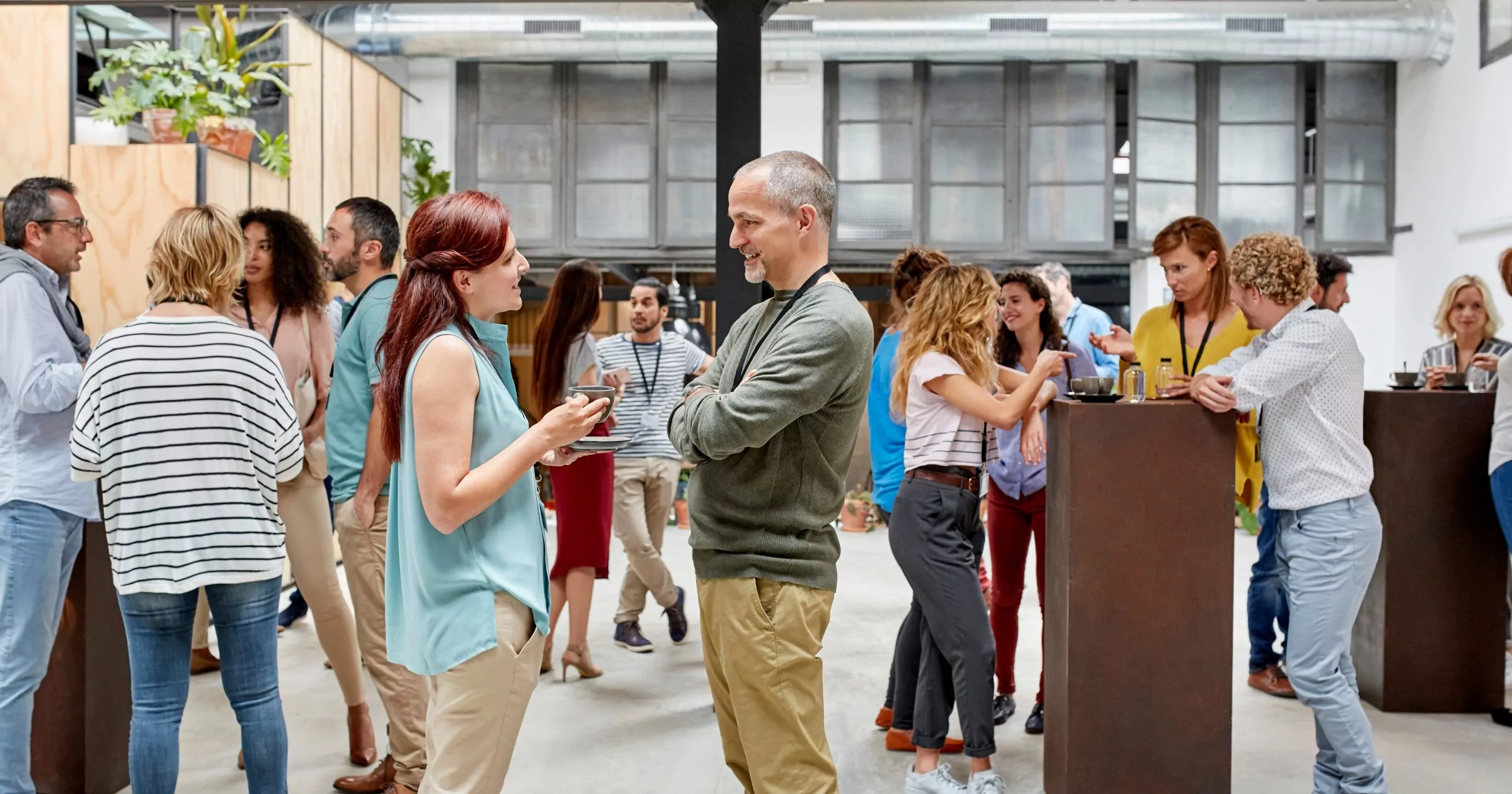
Successful Beta Service release of SOMESING, ‘My Hand-Carry Studio Karaoke App’

Dogwifhat (WIF) large pump on Bybit after record reasons marketplace frenzy

How fintech innovation is riding virtual transformation for communities around the globe

Wasabi Wallet developer bars U.S. customers amidst regulatory considerations

Analyst Foresees Peak In Late 2025
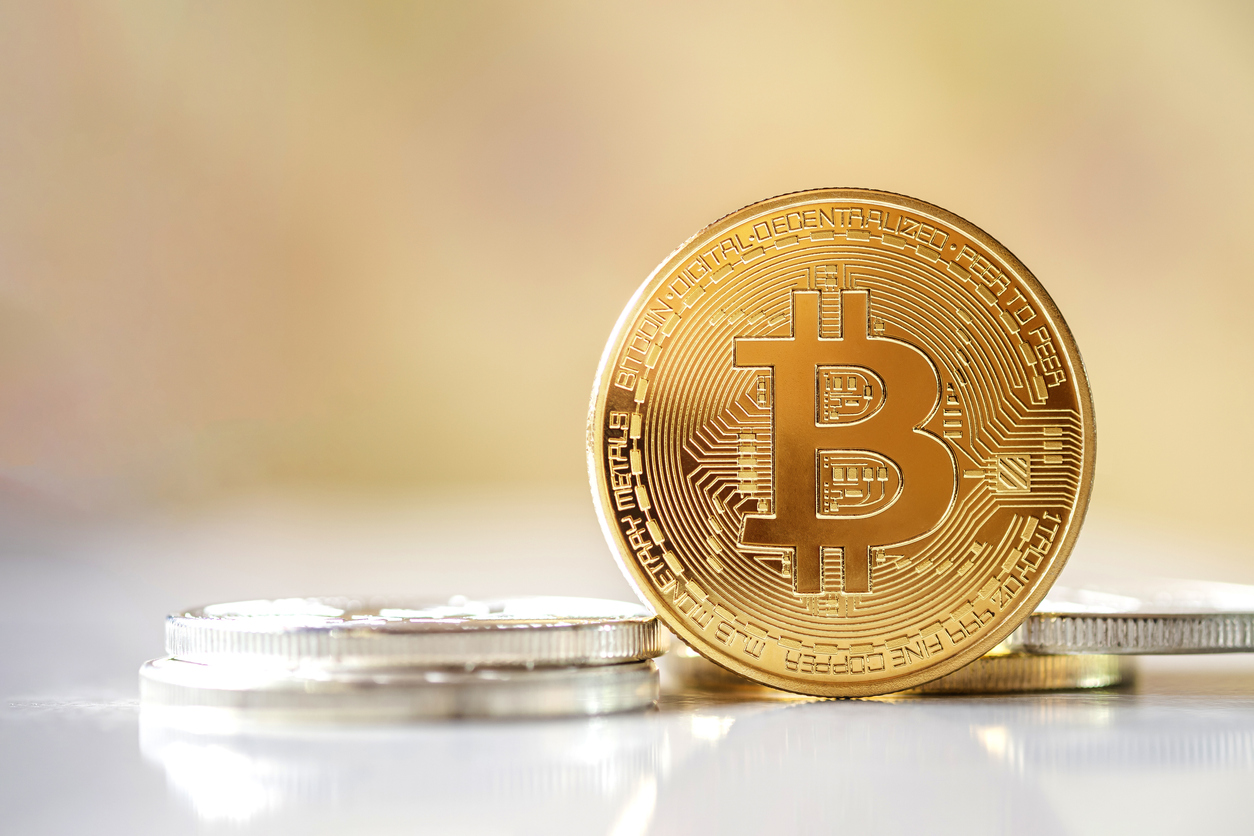
Solo Bitcoin miner wins the three.125 BTC lottery, fixing legitimate block
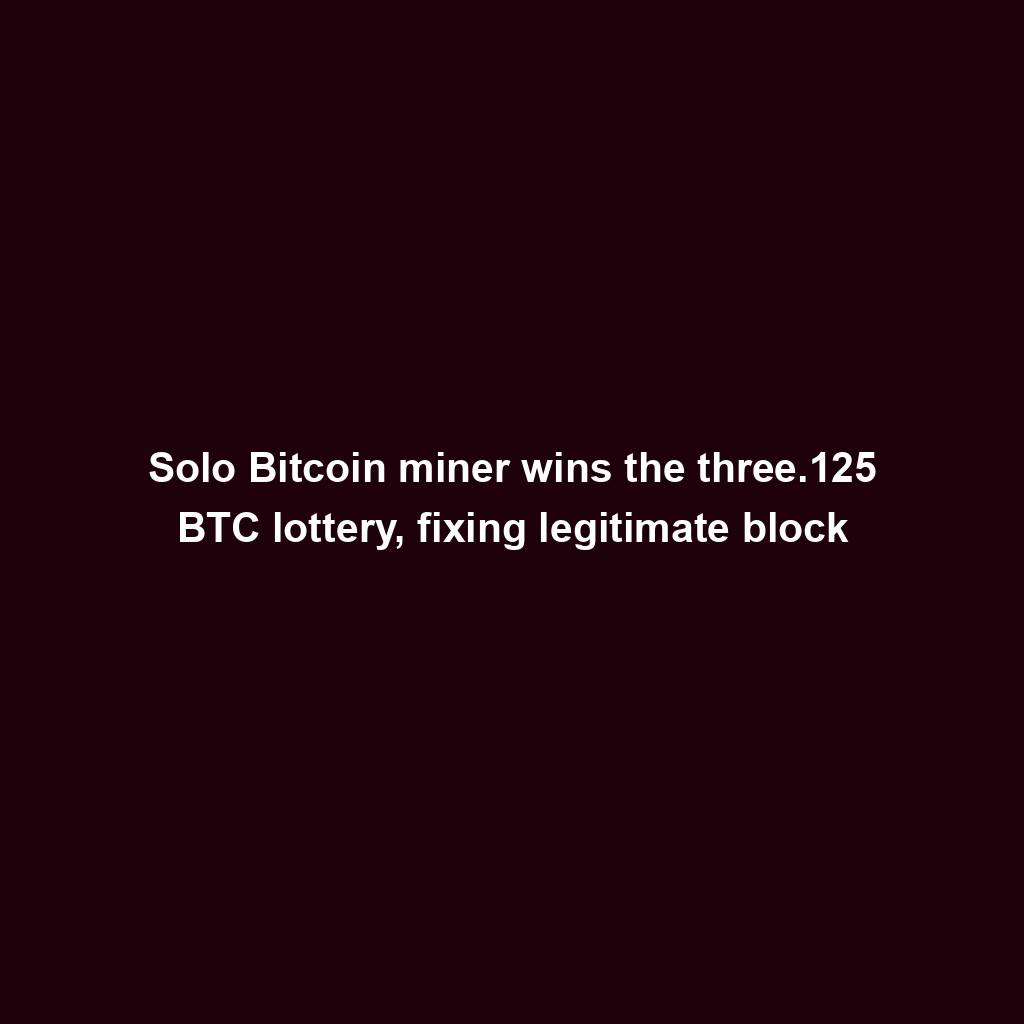
Ace Exchange Suspects Should Get 20-Year Prison Sentences: Prosecutors
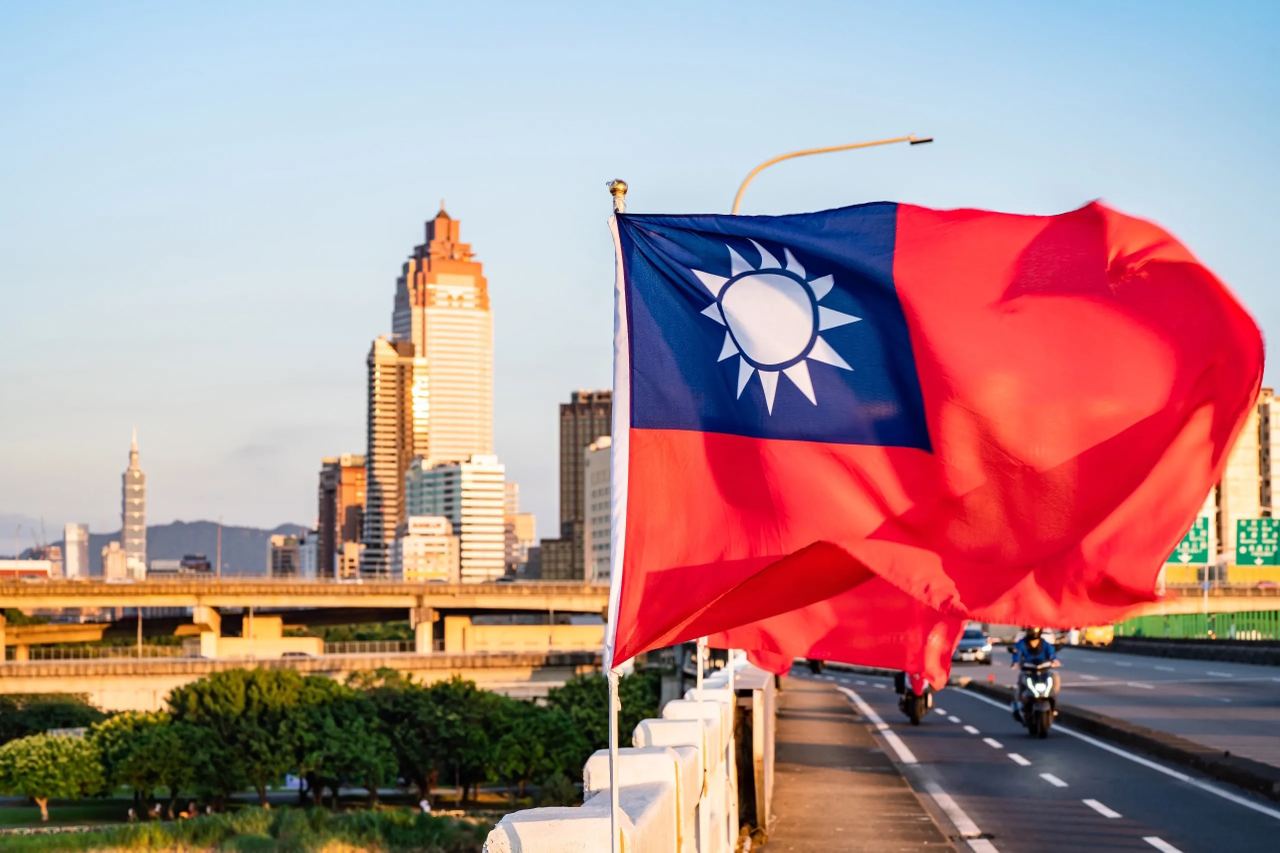
Google Cloud's Web3 portal release sparks debate in crypto trade
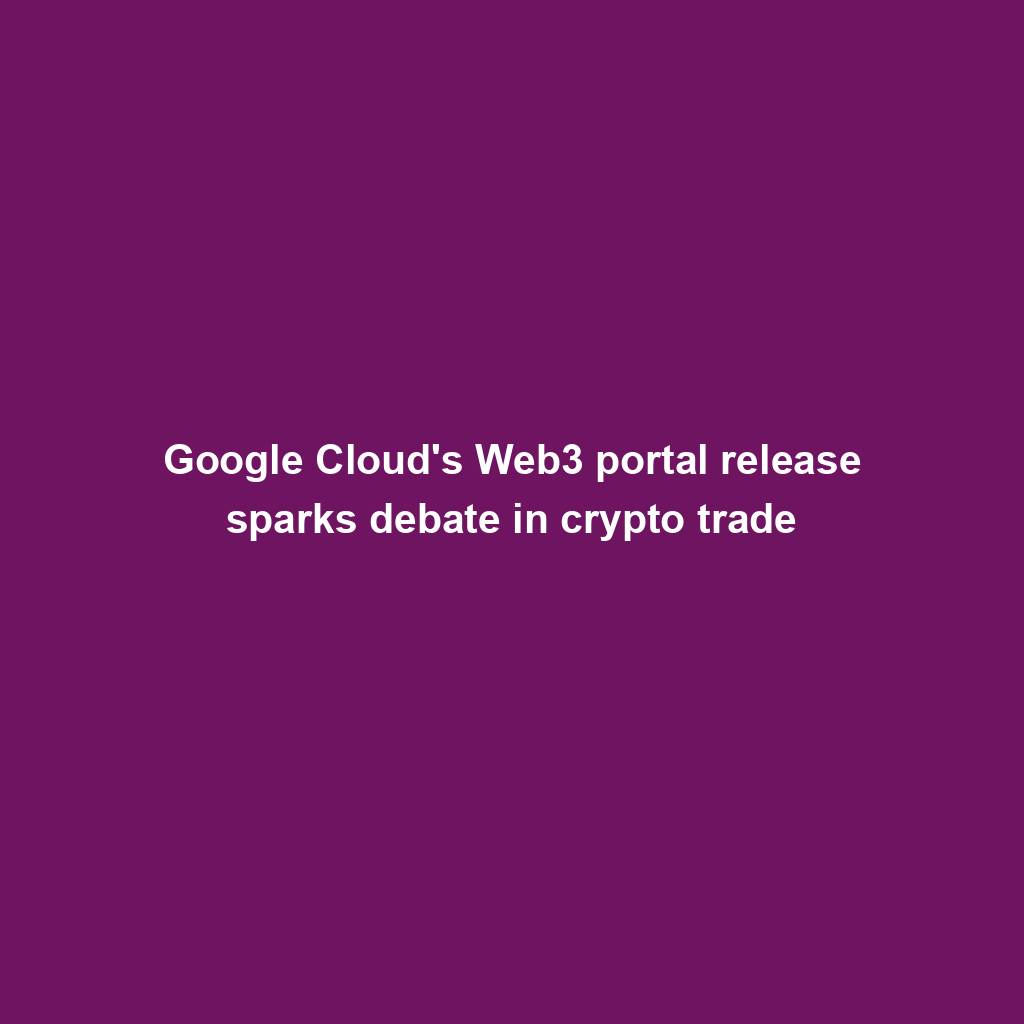
Bitcoin Primed For $77,000 Surge
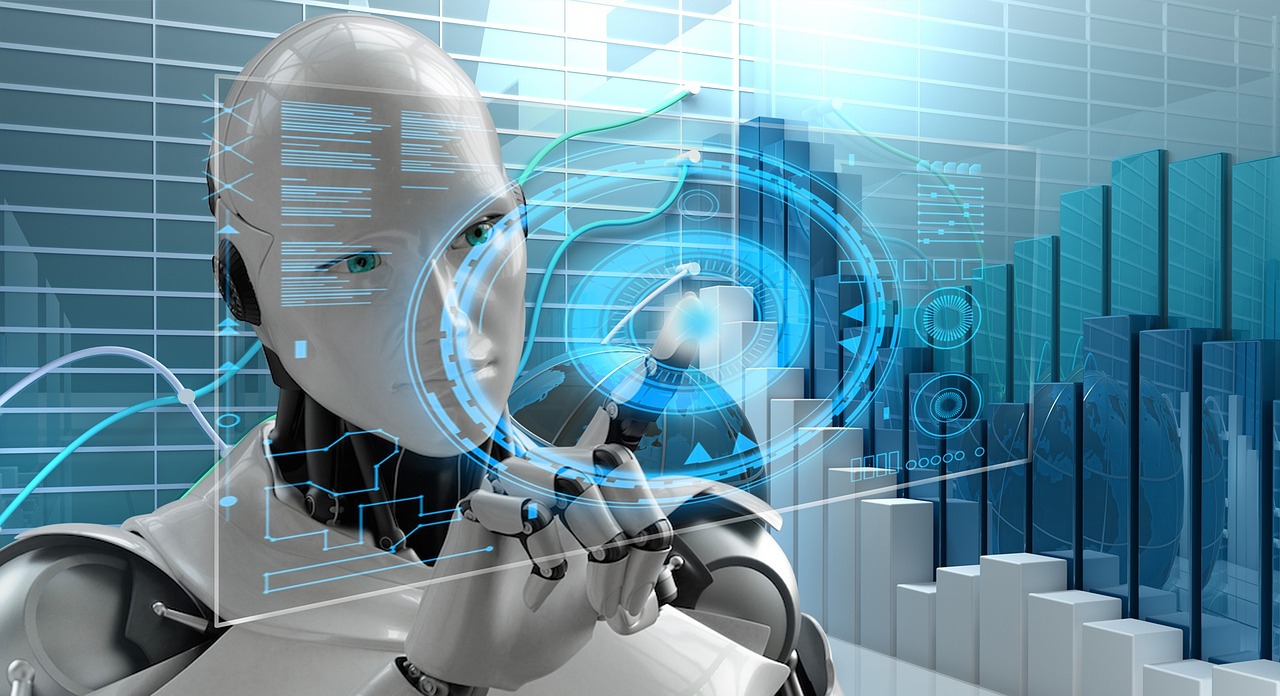
Bitbot’s twelfth presale level nears its finish after elevating $2.87 million
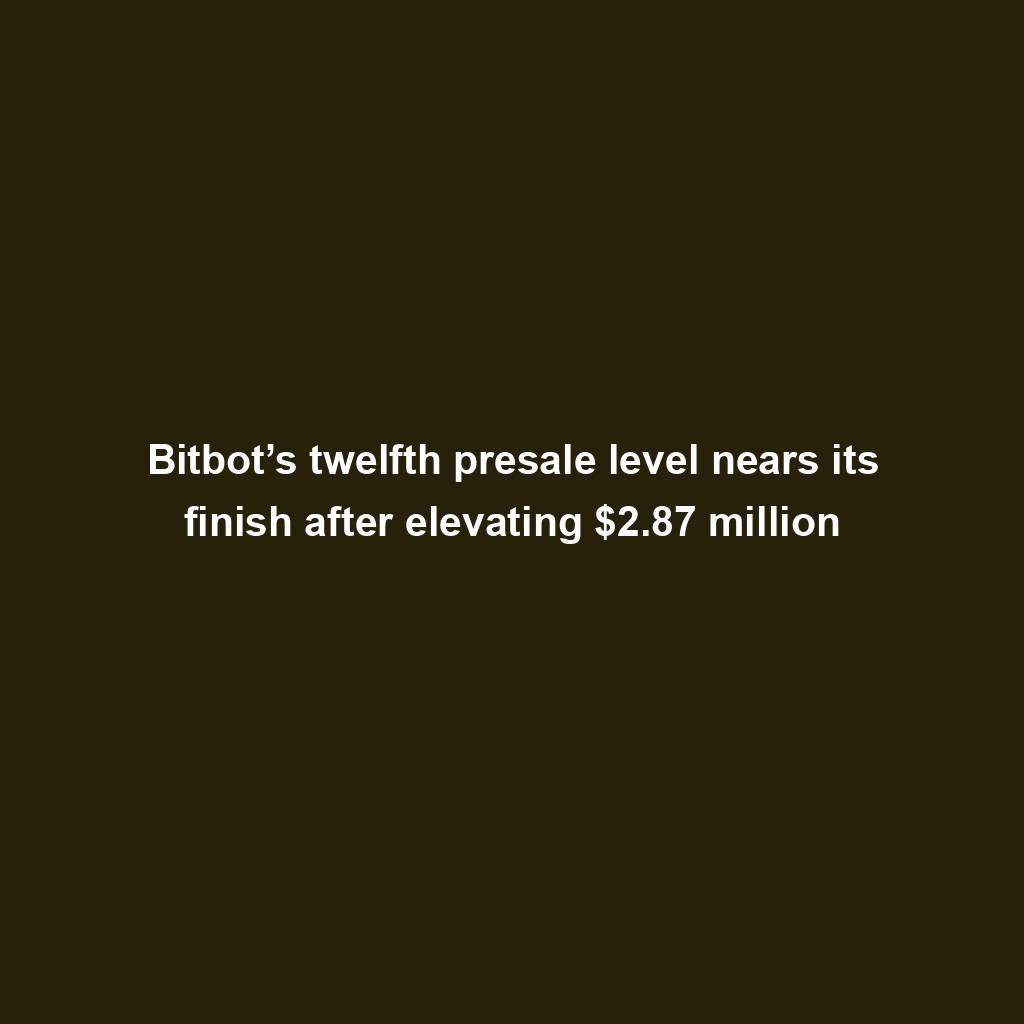
PANDA and MEW bullish momentum cool off: traders shift to new altcoin
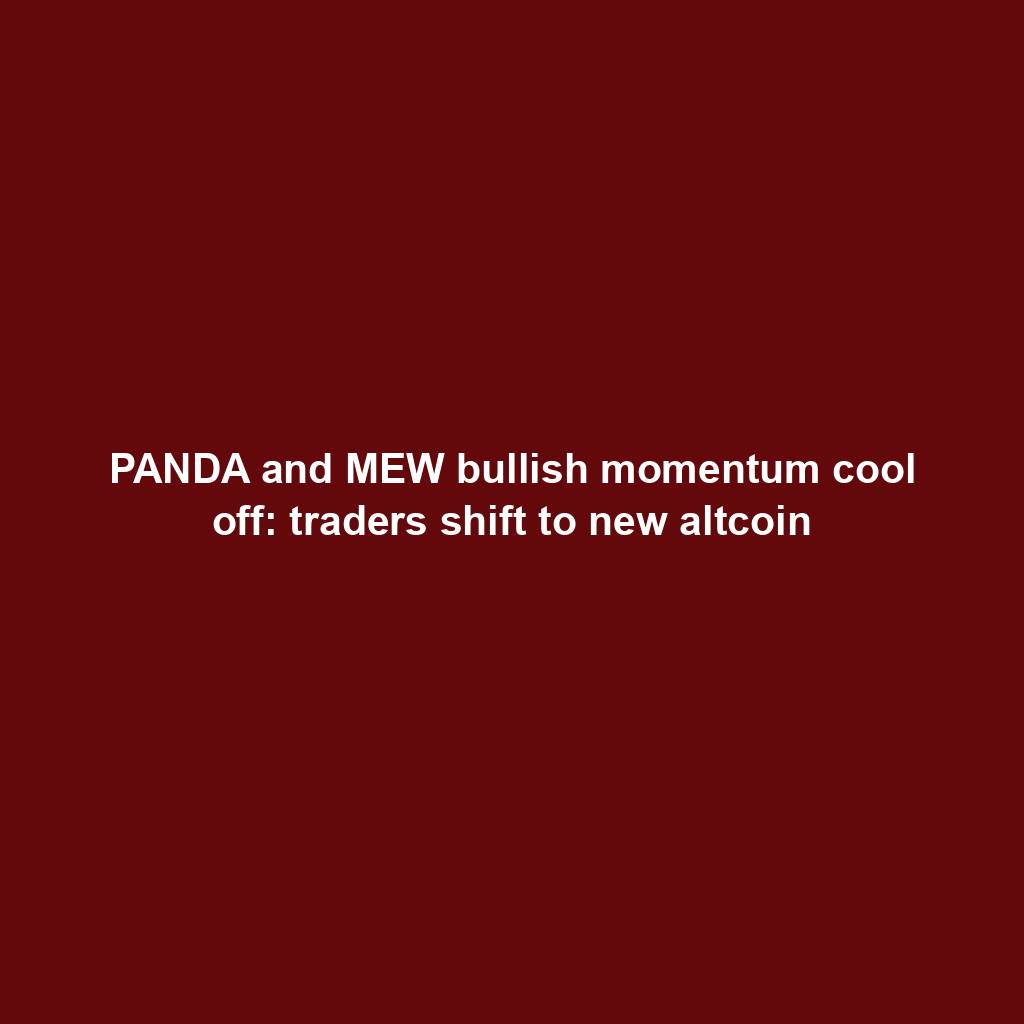
Commerce technique: Ecommerce is useless, lengthy are living ecommerce
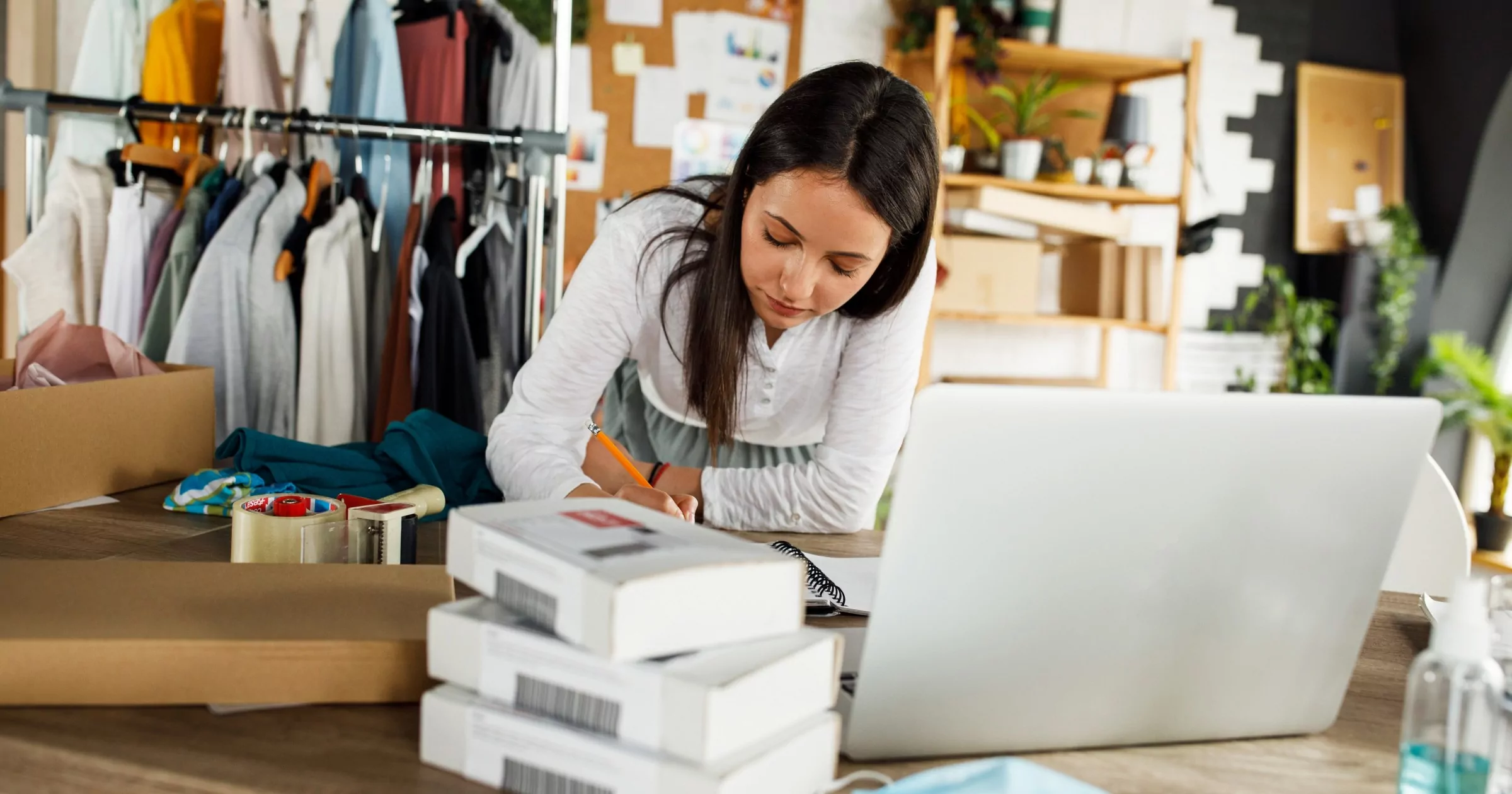
Republic First Bank closed by way of US regulators — crypto neighborhood reacts
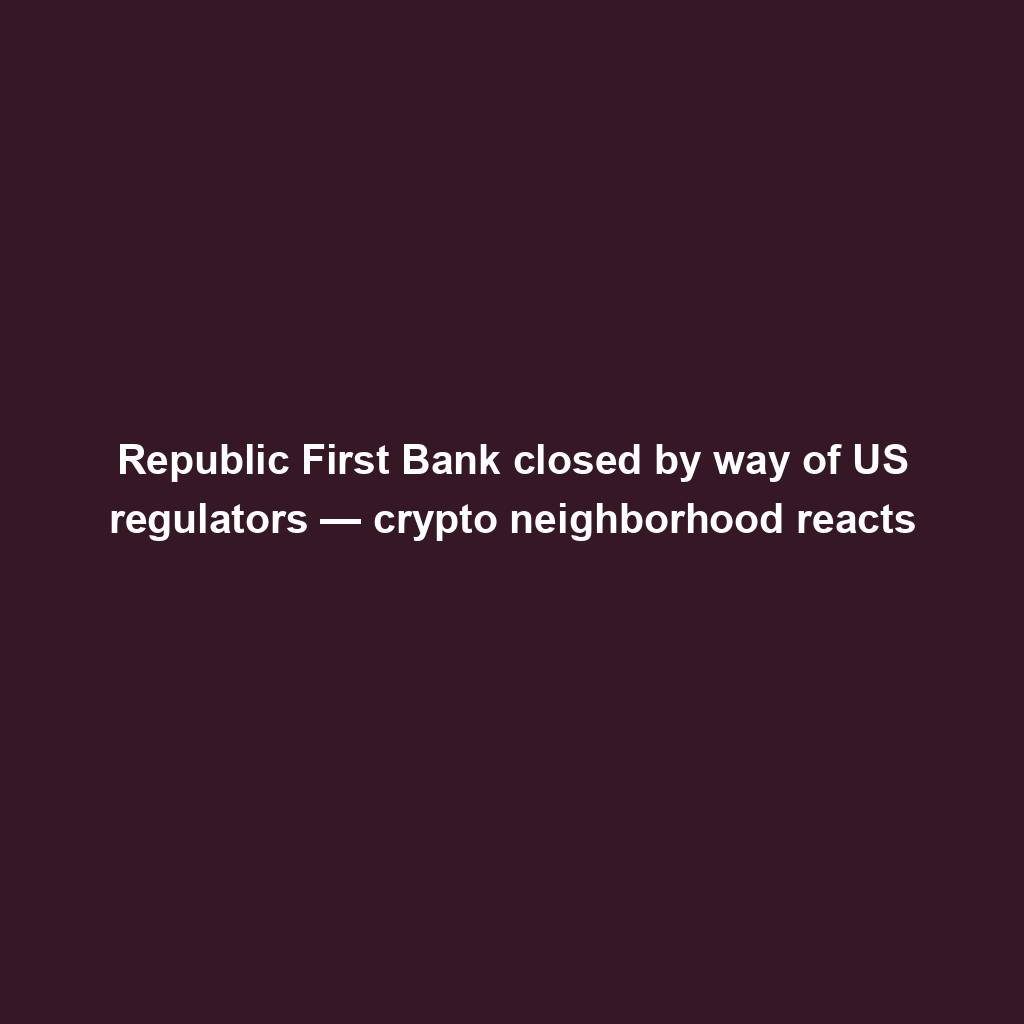
China’s former CBDC leader is beneath executive investigation
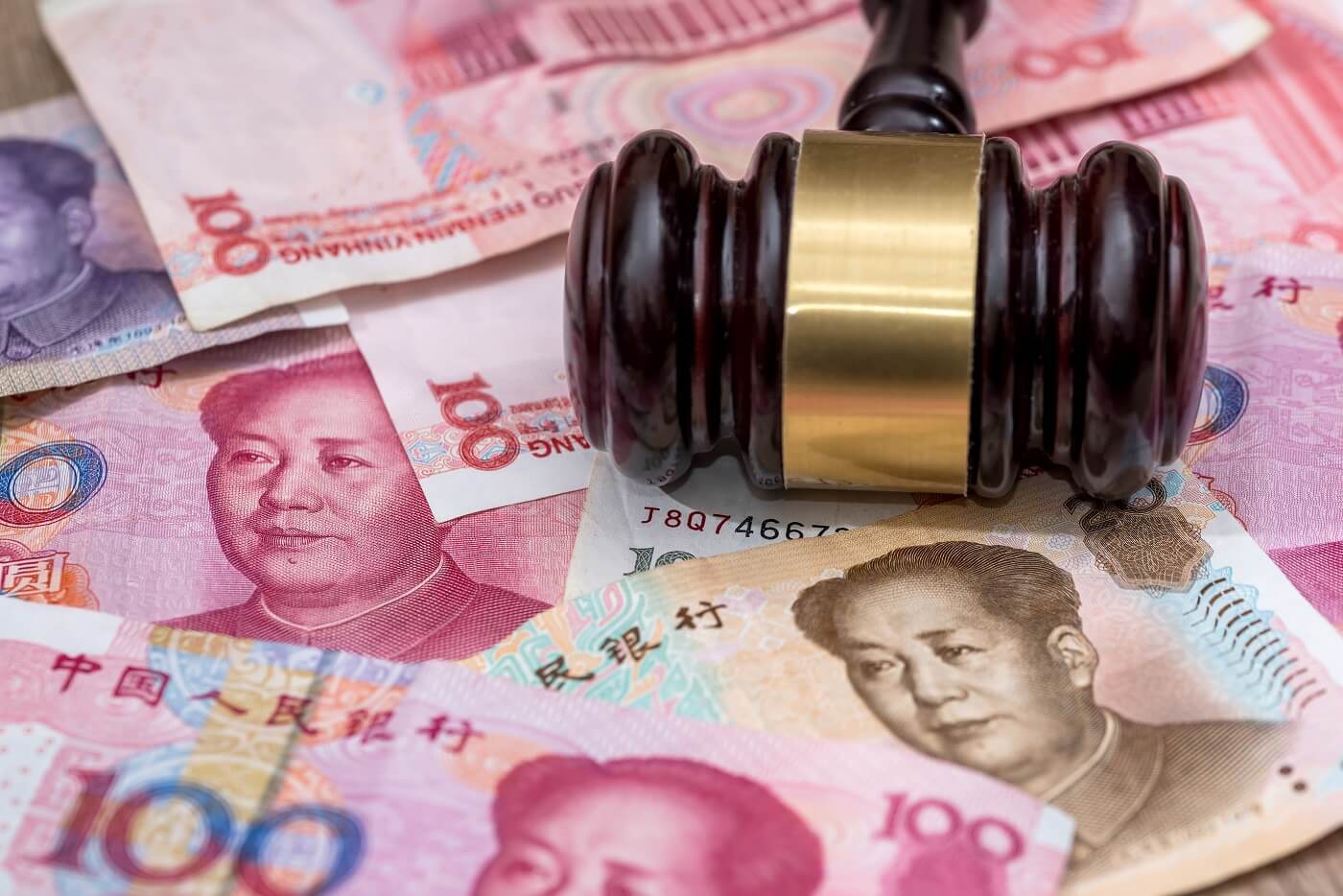
Bigger isn’t all the time higher: How hybrid Computational Intelligence development permits smaller language fashions
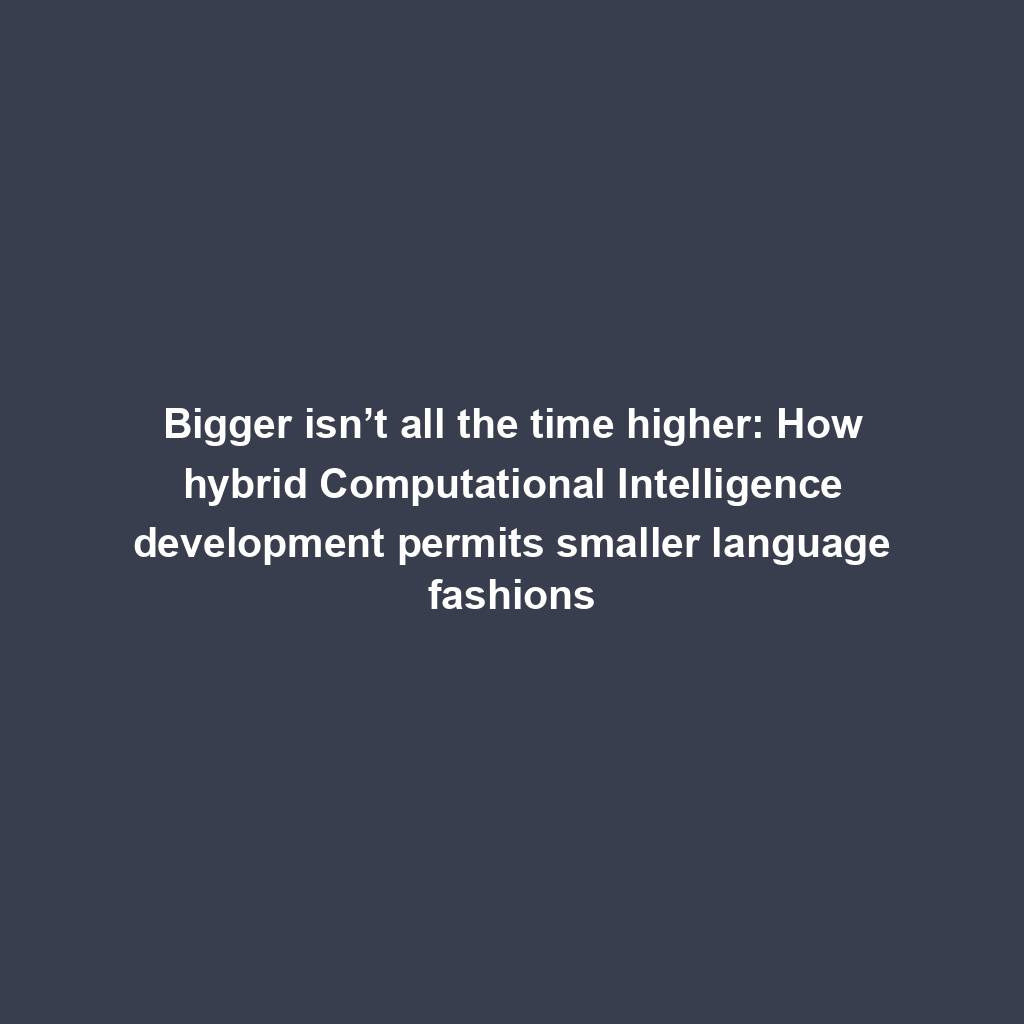
Pantera Capital buys extra Solana (SOL) from FTX
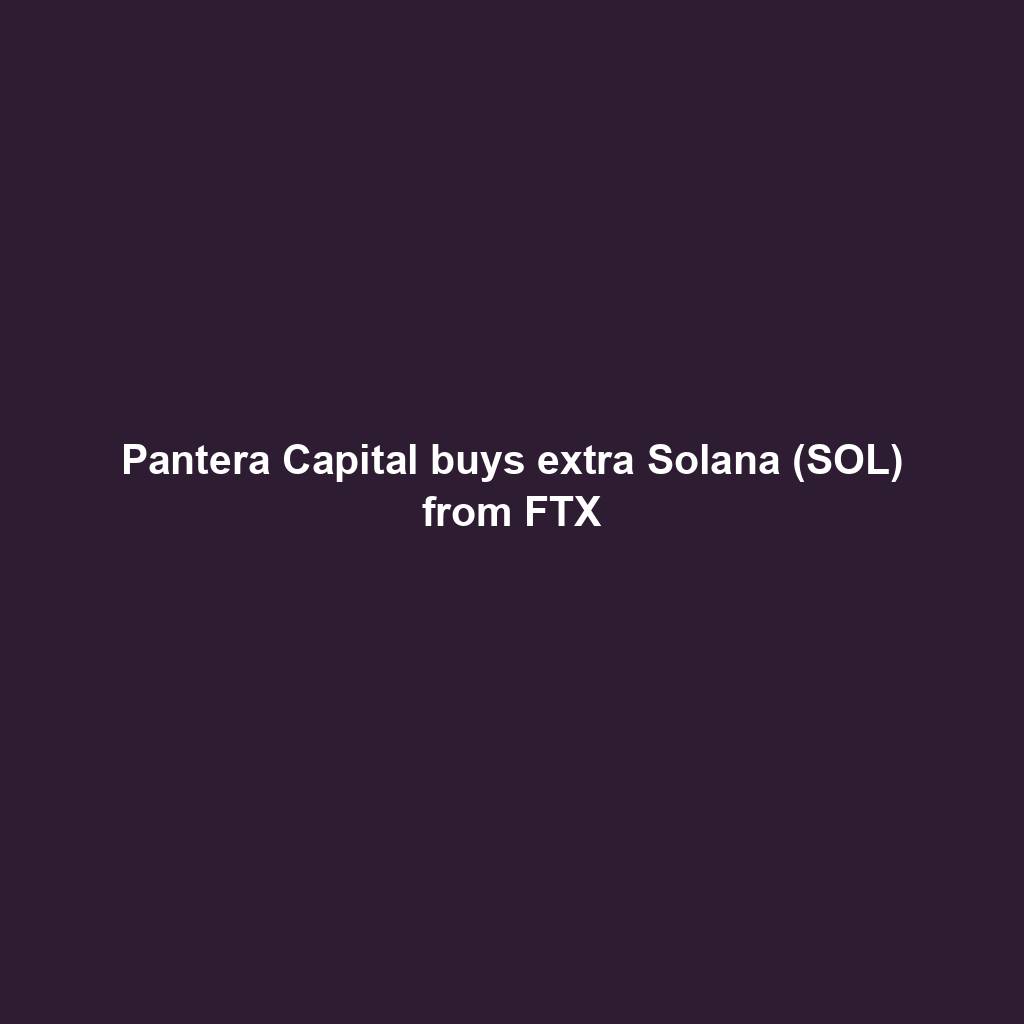
Successful Beta Service release of SOMESING, ‘My Hand-Carry Studio Karaoke App’

SEC sues Bitcoin miner Geosyn Mining for fraud; Bitbot presale nears $3M
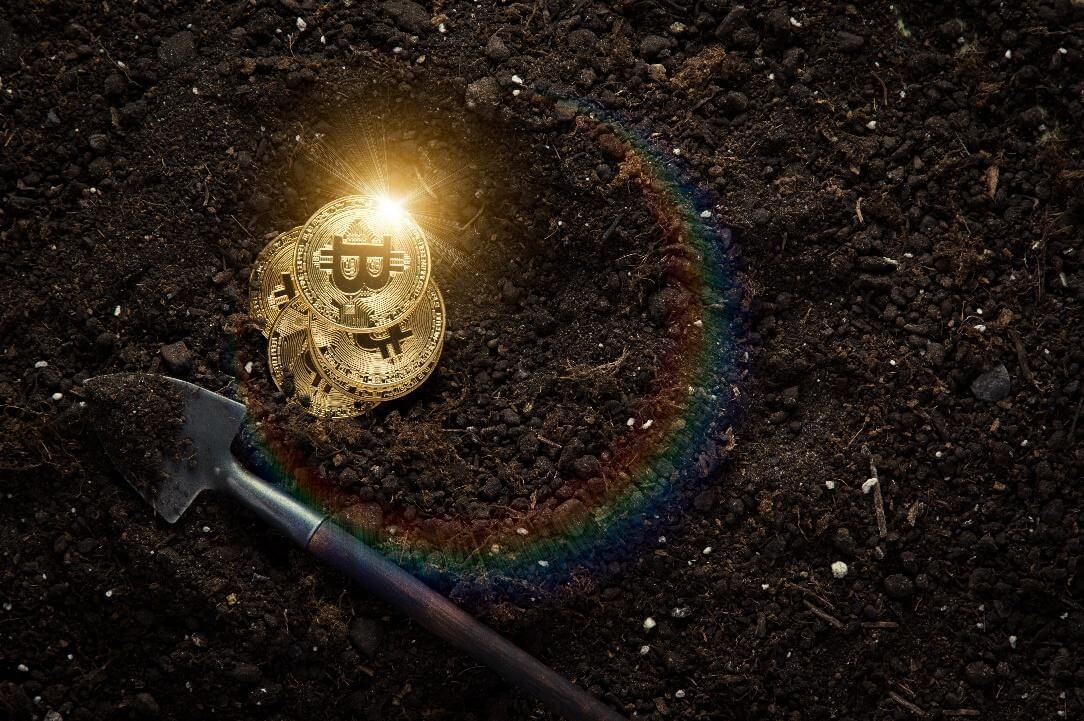
Business procedure reengineering (BPR) examples
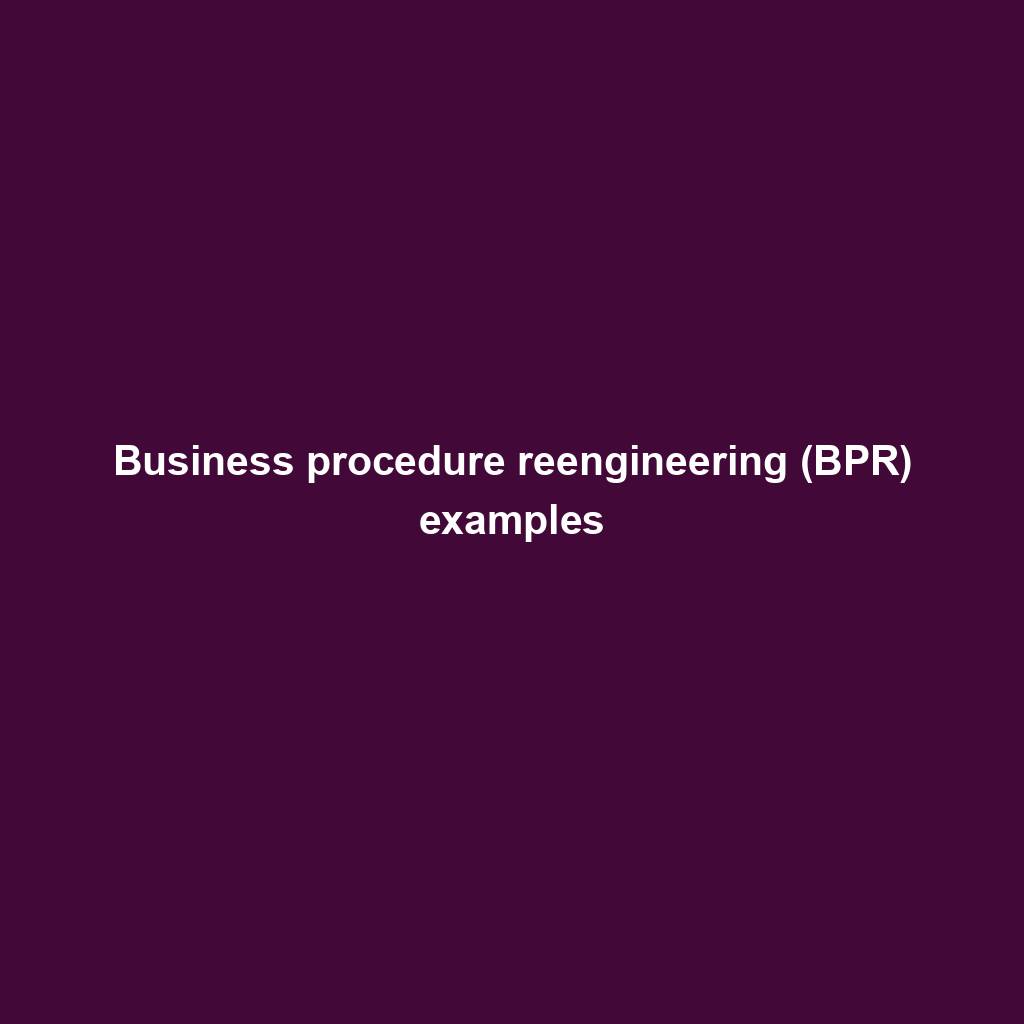
85% Of Altcoins In “Opportunity Zone,” Santiment Reveals
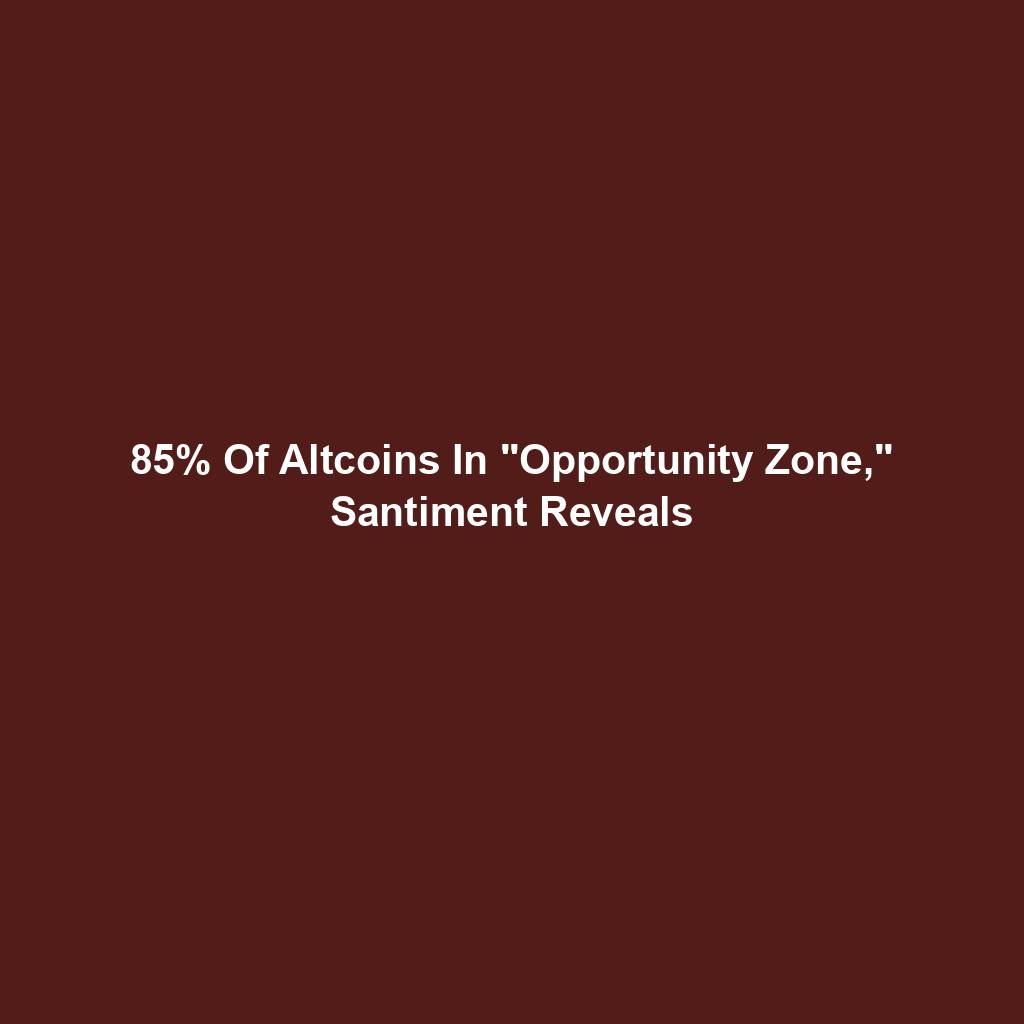
Sam Altman’s Worldcoin eyeing PayPal and OpenAI partnerships
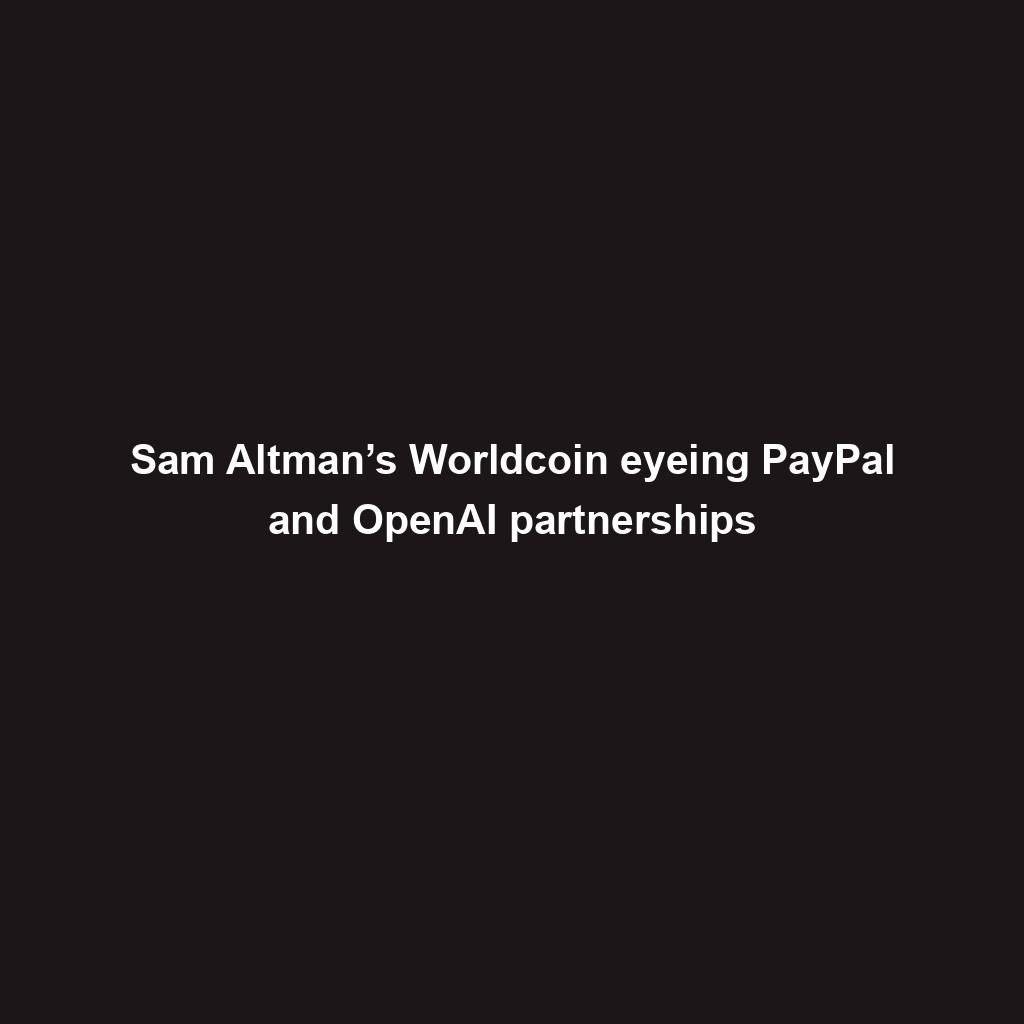
Artificial Intelligence transforms the IT strengthen enjoy
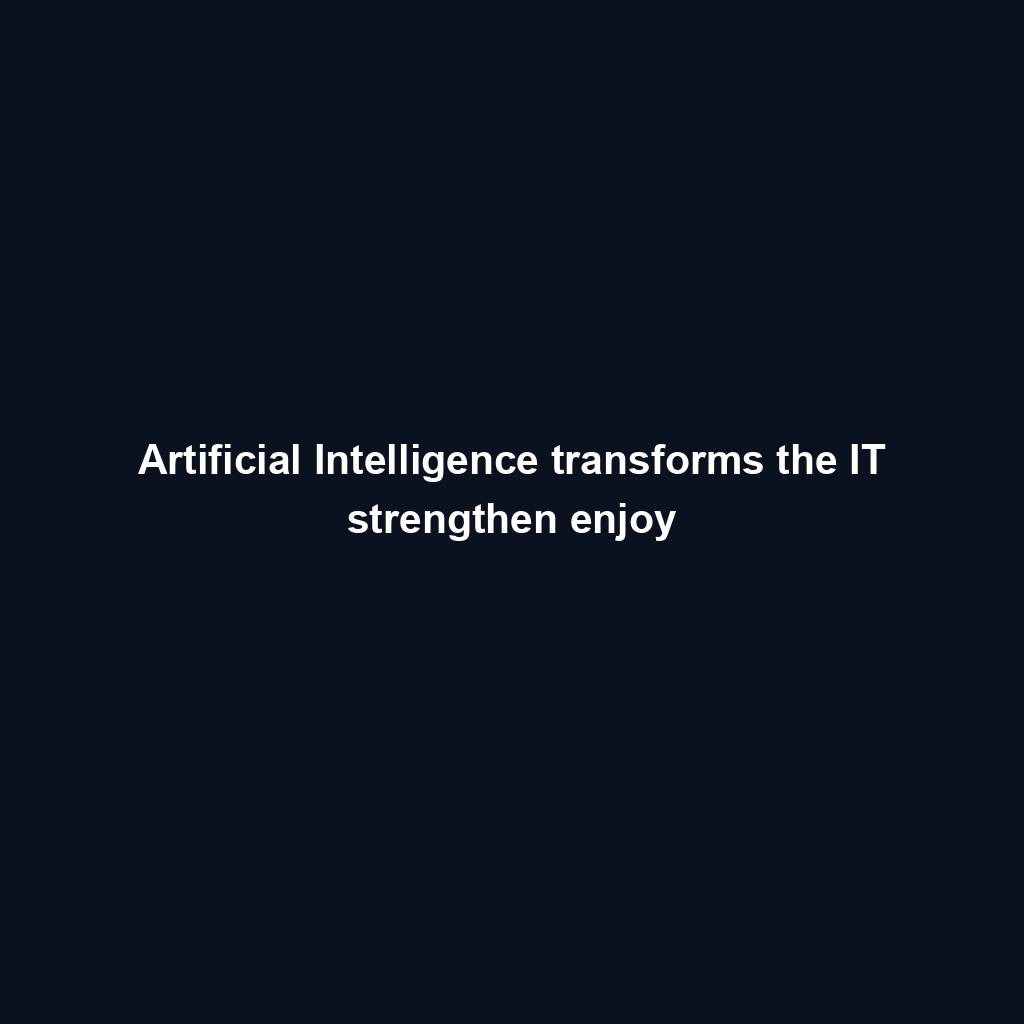
Franklin Templeton tokenizes $380M fund on Polygon and Stellar for P2P transfers
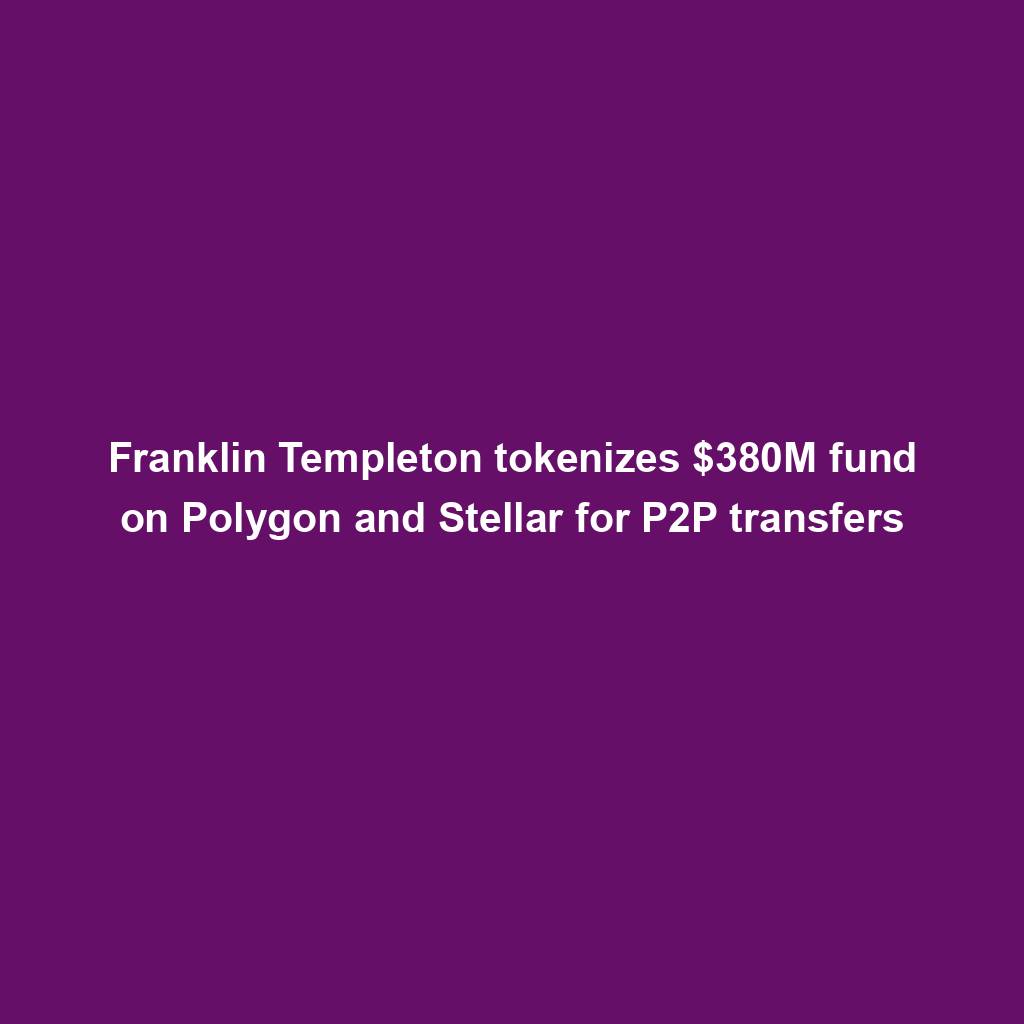
Meta’s letting Xbox, Lenovo, and Asus construct new Quest metaverse {hardware}
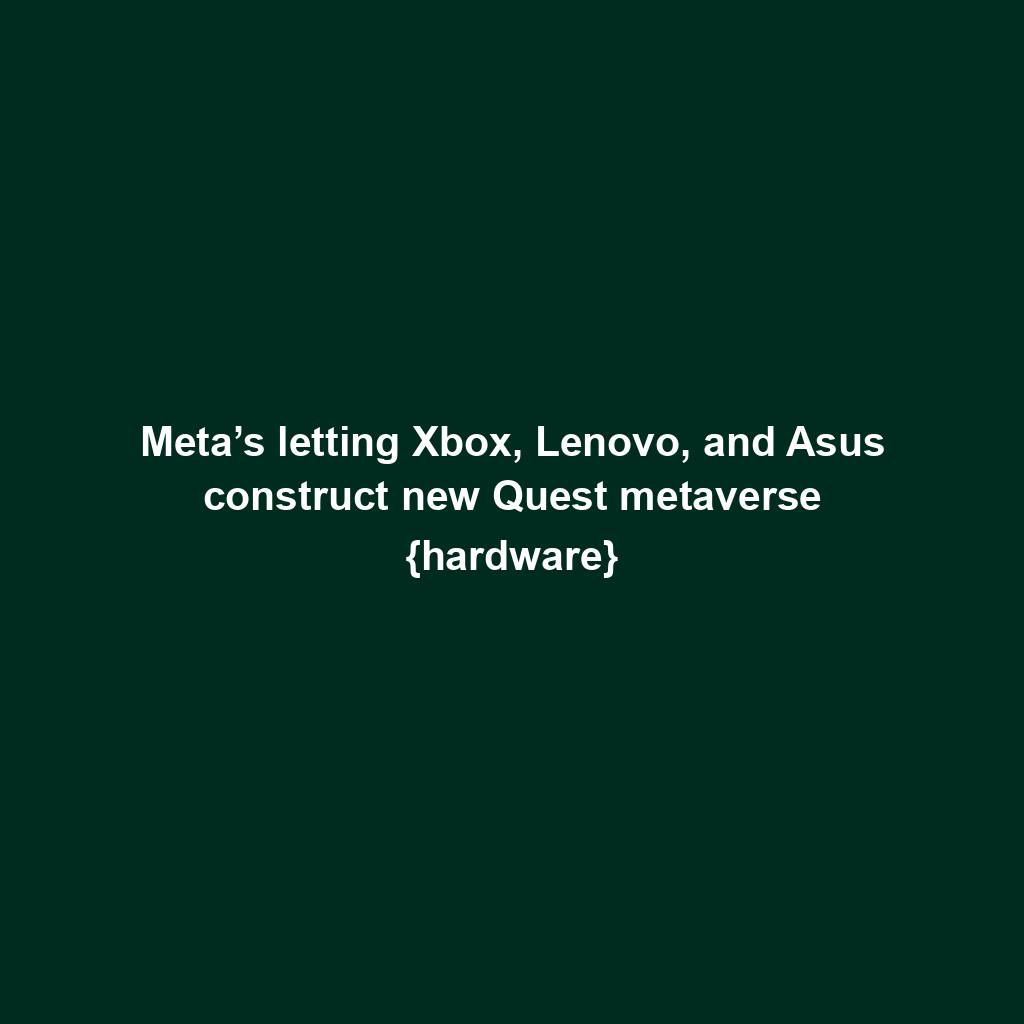
Shiba Inu (SHIB) unveils bold Shibarium plans as Kangamoon steals the display
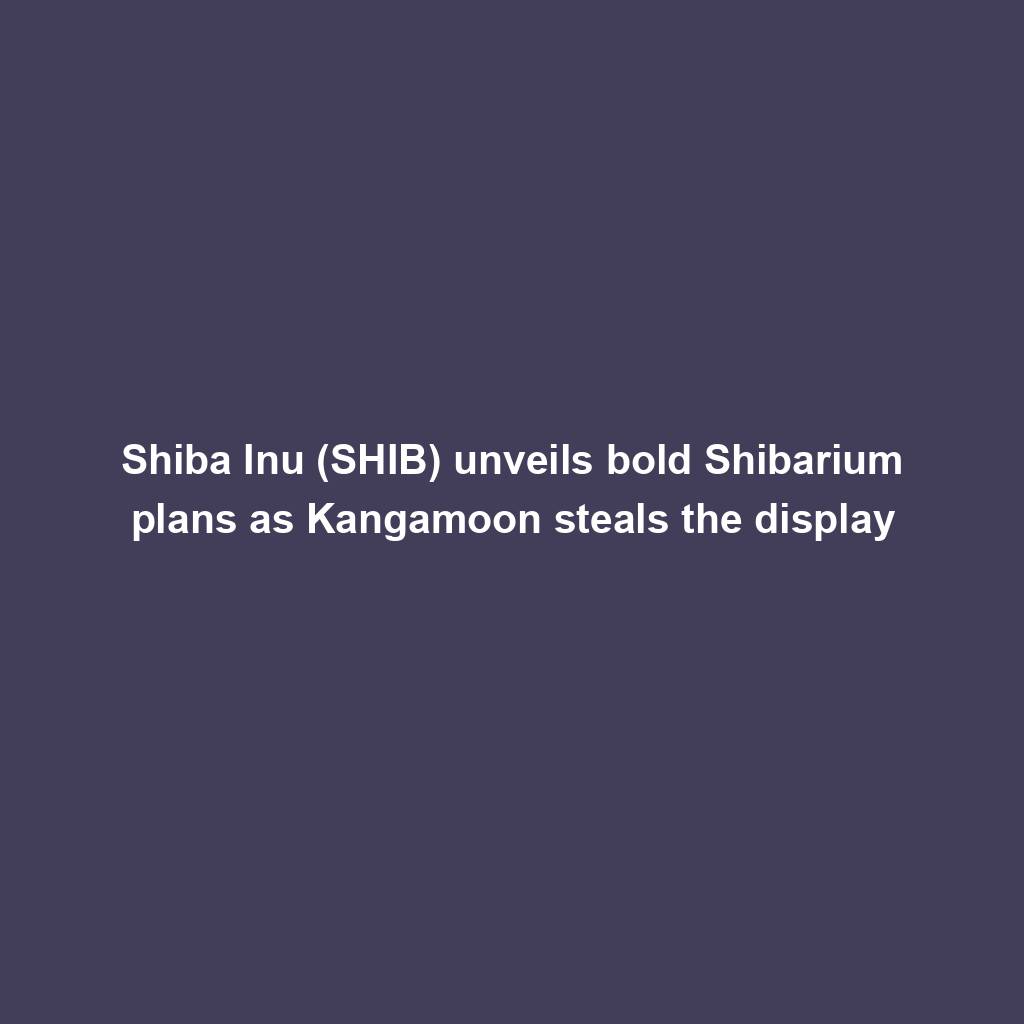