Efficiently managing clinical trials is essential for the pharmaceutical industry, but site selection and enrollment challenges can hinder success. However, the integration of artificial intelligence (AI) into the clinical trial process can provide valuable insights and optimize site performance. In this article, we explore three AI capabilities that can significantly improve clinical trial site selection and performance management.
Summary
Delivering drugs to market is a complex process with room for improvement, particularly in the area of clinical trials. Inefficiencies in clinical trial site selection and patient enrollment contribute to delays and increased costs for pharmaceutical companies. To address these challenges, integrating AI into the clinical trial process can provide valuable insights, accelerate recruitment times, reduce site footprint, and result in cost savings. This article highlights three AI capabilities that can optimize clinical trial site performance: AI-driven enrollment rate prediction, real-time monitoring and forecasting of site performance, and leveraging a Next Best Action (NBA) engine for mitigation plan execution.
Tackling complexities in clinical trial site selection
Constructing robust enrollment strategies and prioritizing clinical trial sites require data that can indicate site performance. However, the challenge lies in understanding which data is indicative of site performance and predicting the performance of sites that may not meet recruitment expectations. By utilizing AI algorithms, biopharma companies can analyze historical performance data and real-world data to accurately forecast enrollment rates, improve feasibility assessments, and optimize budget planning. Implementing AI technology in site selection can enhance accuracy and scalability, revolutionizing the clinical trial process.
Embracing AI for clinical trials
By embracing three AI-enabled capabilities, biopharma companies can optimize the clinical trial site selection process and develop core AI competencies. The first capability is AI-driven enrollment rate prediction, which allows for accurate forecasting of enrollment rates and helps in factoring non-performing sites into the enrollment strategy. The second capability is real-time monitoring and forecasting of site performance, which provides up-to-date insights and enables proactive decision-making and course corrections. The third capability is leveraging a Next Best Action (NBA) engine for mitigation plan execution, which recommends effective interventions to optimize site performance in real-time. These AI capabilities empower companies to make informed decisions, improve trial success rates, and save valuable resources.
Shattering the status quo
Clinical trials are crucial for the pharmaceutical industry, but delays can hinder progress. To address trial management challenges, understanding the process and people involved is vital. Biopharma companies should adopt a long-term AI strategy that includes building AI capabilities within the trial process to enable enrollment forecasting, real-time site monitoring, and data-driven recommendations. This approach can generate significant savings and boost confidence in AI investments. Additionally, IBM Consulting and Pfizer are collaborating to explore the integration of quantum computing with classical machine learning to accurately predict clinical trial sites at risk of recruitment failure. Quantum computing offers a transformative technology to solve complex problems in the industry.
FAQs
1. How can AI improve clinical trial site selection?
AI can enhance clinical trial site selection by analyzing historical performance data and real-world data to accurately forecast enrollment rates and optimize budget planning. By leveraging AI algorithms, biopharma companies can identify non-performing sites, factor in site performance into the enrollment strategy, and improve feasibility assessments.
2. What are the benefits of real-time monitoring and forecasting of site performance?
Real-time monitoring and forecasting of site performance provide up-to-date insights on enrollment progress, facilitate early detection of performance issues, and enable proactive decision-making and course corrections. It empowers teams to enhance enrollment forecasting, detect potential issues, and allocate resources effectively to maximize the success of the clinical trial.
3. How can a Next Best Action (NBA) engine optimize site performance?
A Next Best Action (NBA) engine utilizes AI algorithms to analyze real-time site performance data, predict future events, and recommend the most effective mitigation actions or interventions in real-time. By exploring different scenarios and evaluating trade-offs, the NBA engine helps stakeholders make informed decisions and optimize site performance during the trial.
4. How can the integration of quantum computing improve clinical trial prediction?
In collaboration with IBM Consulting, Pfizer is exploring the integration of quantum computing with classical machine learning to accurately predict clinical trial sites at risk of recruitment failure. Quantum computing, a rapidly emerging technology, utilizes the principles of quantum mechanics to solve complex problems too difficult for classical computers. By combining quantum computing and machine learning, clinical trial predictions can be enhanced, leading to more efficient and successful trials.
More in this category ...
Ripple companions with SBI Group and HashKey DX for XRPL answers in Japan

April sees $25M in exploits and scams, marking historic low ― Certik

MSTR, COIN, RIOT and different crypto shares down as Bitcoin dips

EigenLayer publicizes token release and airdrop for the group

VeloxCon 2024: Innovation in knowledge control
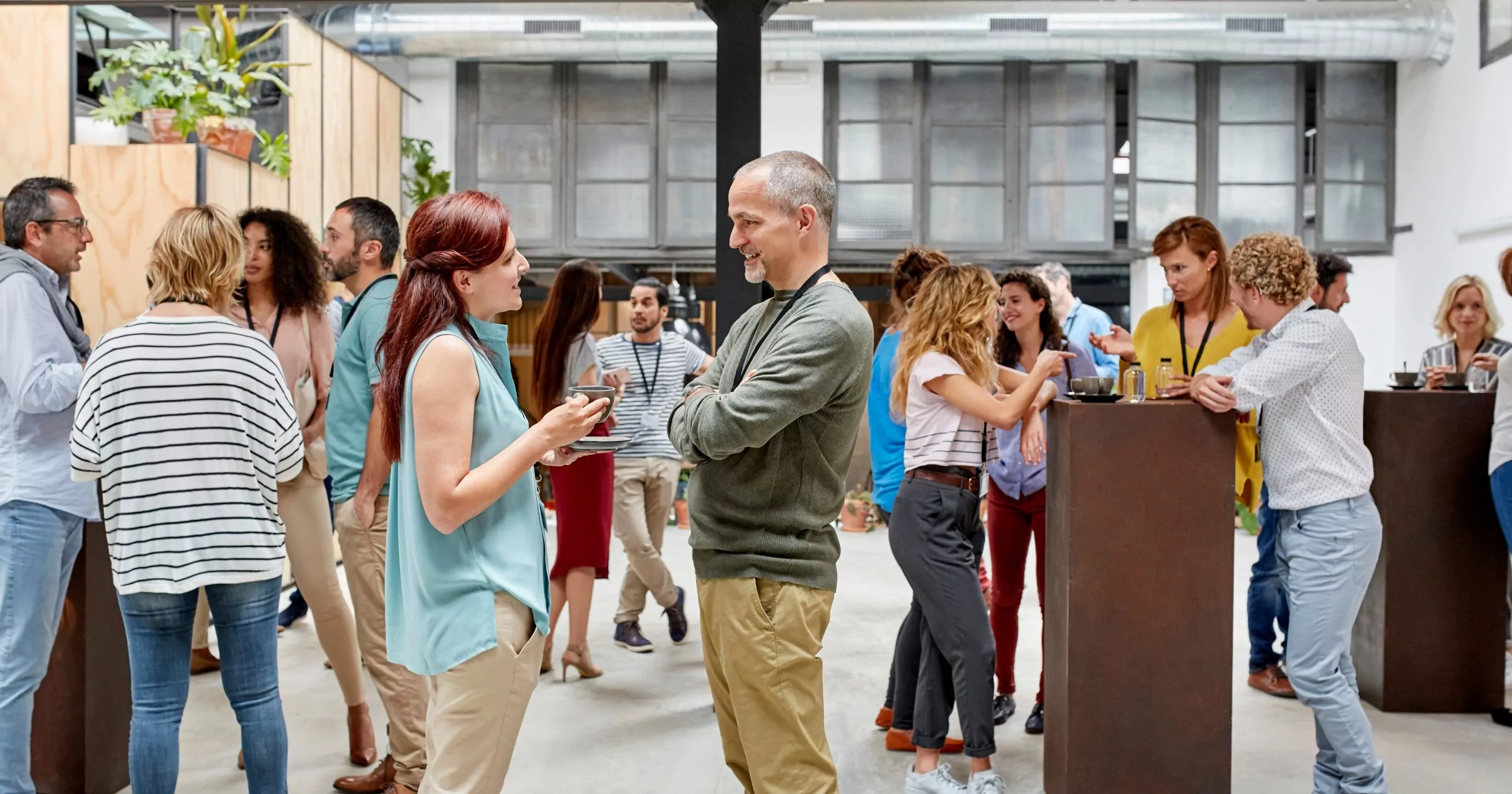
Successful Beta Service release of SOMESING, ‘My Hand-Carry Studio Karaoke App’

Dogwifhat (WIF) large pump on Bybit after record reasons marketplace frenzy

How fintech innovation is riding virtual transformation for communities around the globe

Wasabi Wallet developer bars U.S. customers amidst regulatory considerations

Analyst Foresees Peak In Late 2025
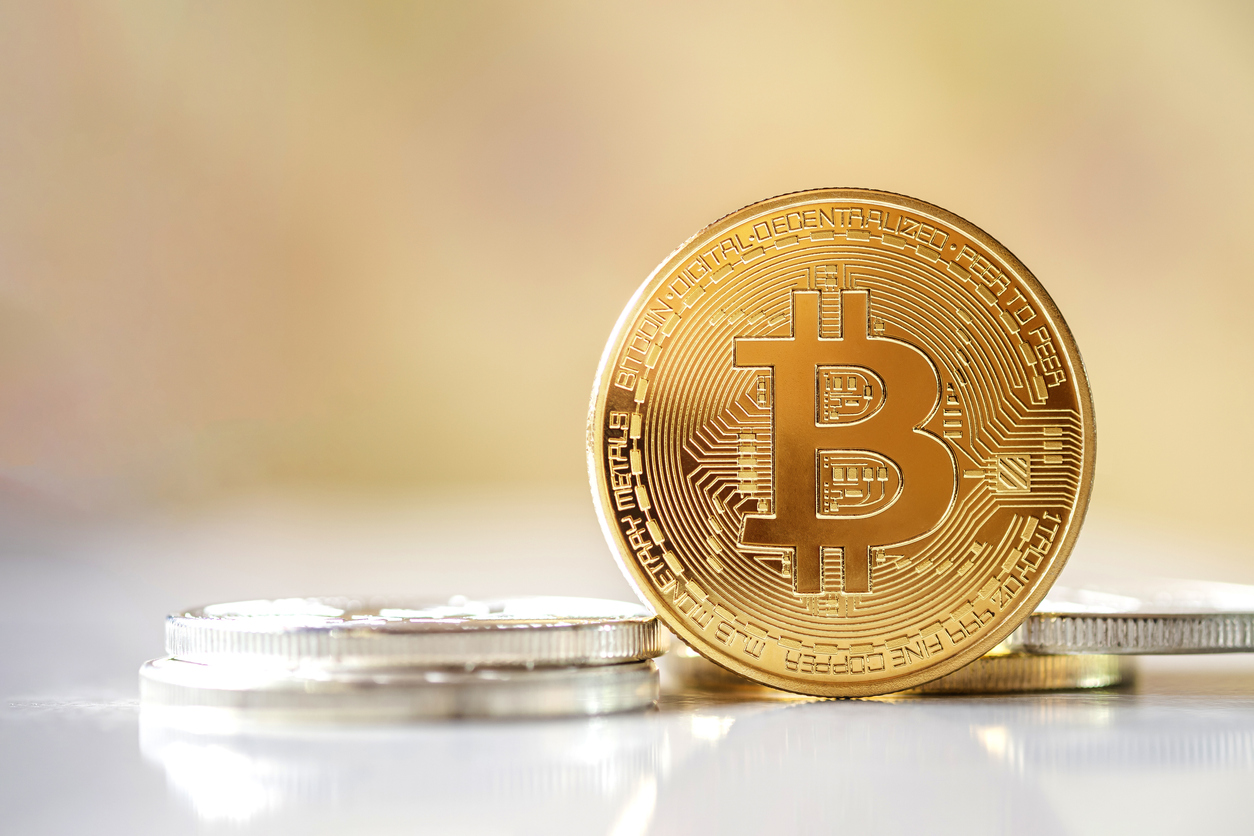
Solo Bitcoin miner wins the three.125 BTC lottery, fixing legitimate block
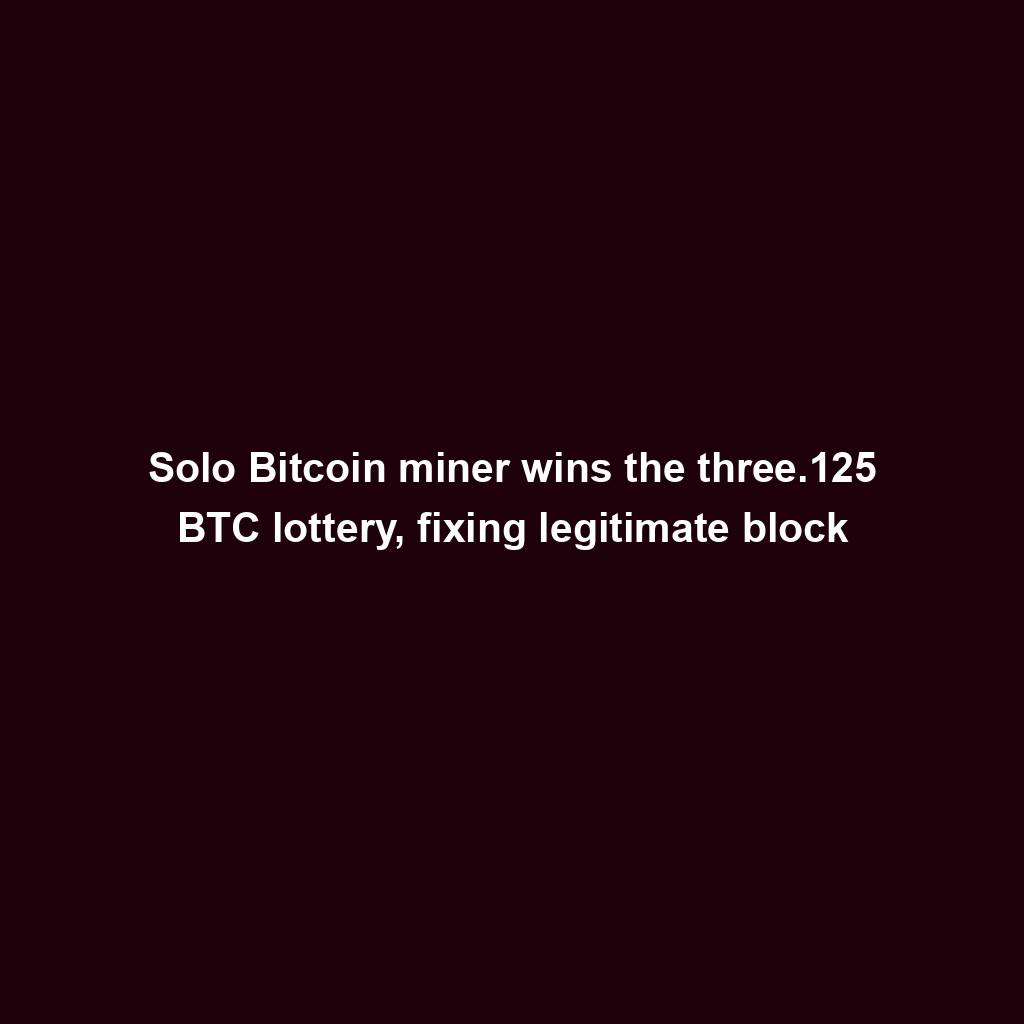
Ace Exchange Suspects Should Get 20-Year Prison Sentences: Prosecutors
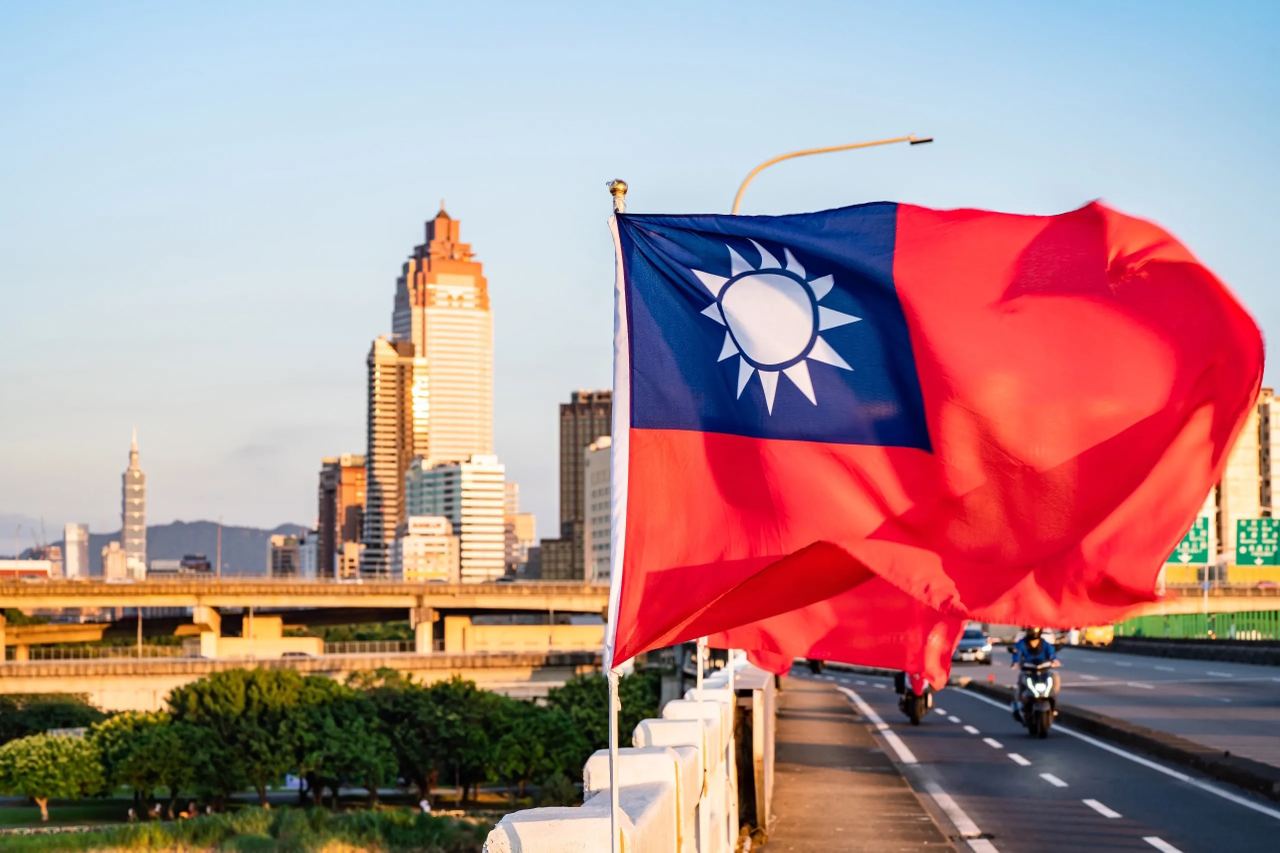
Google Cloud's Web3 portal release sparks debate in crypto trade
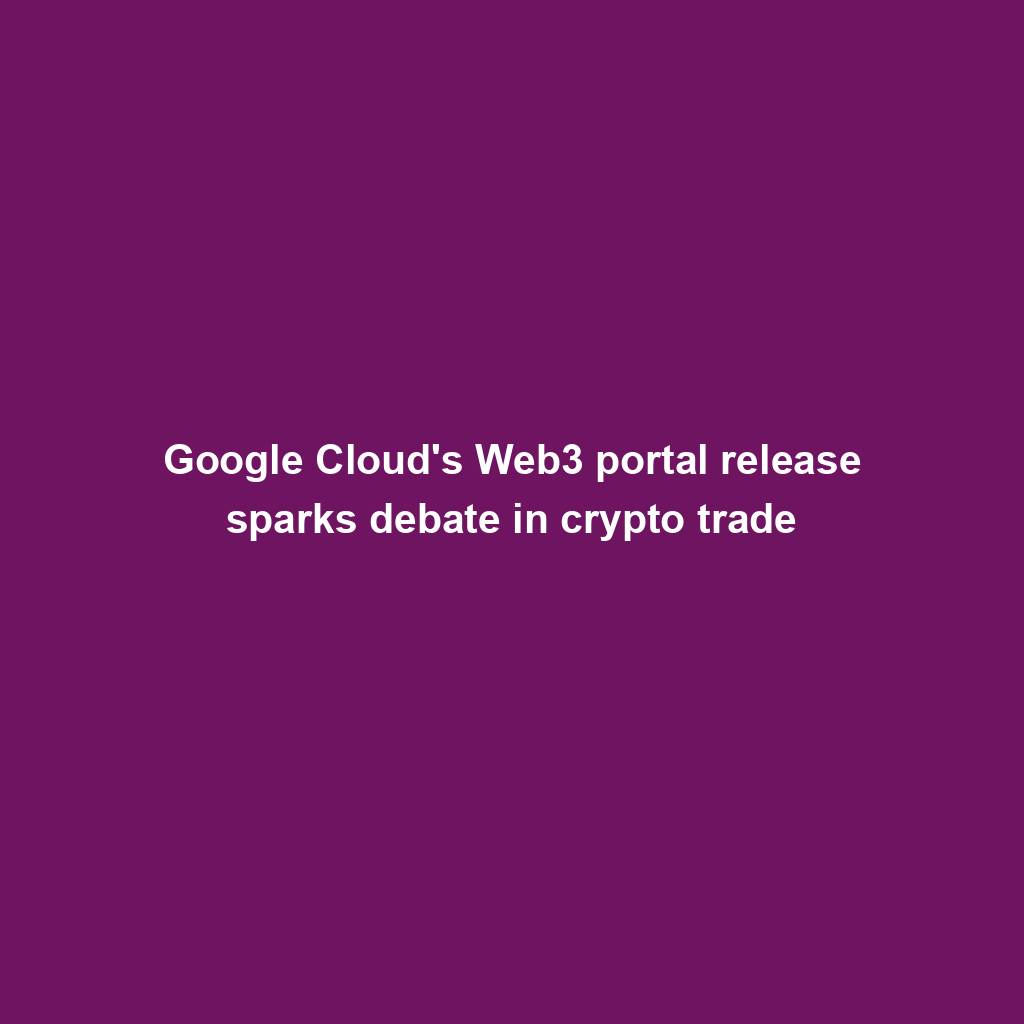
Bitcoin Primed For $77,000 Surge
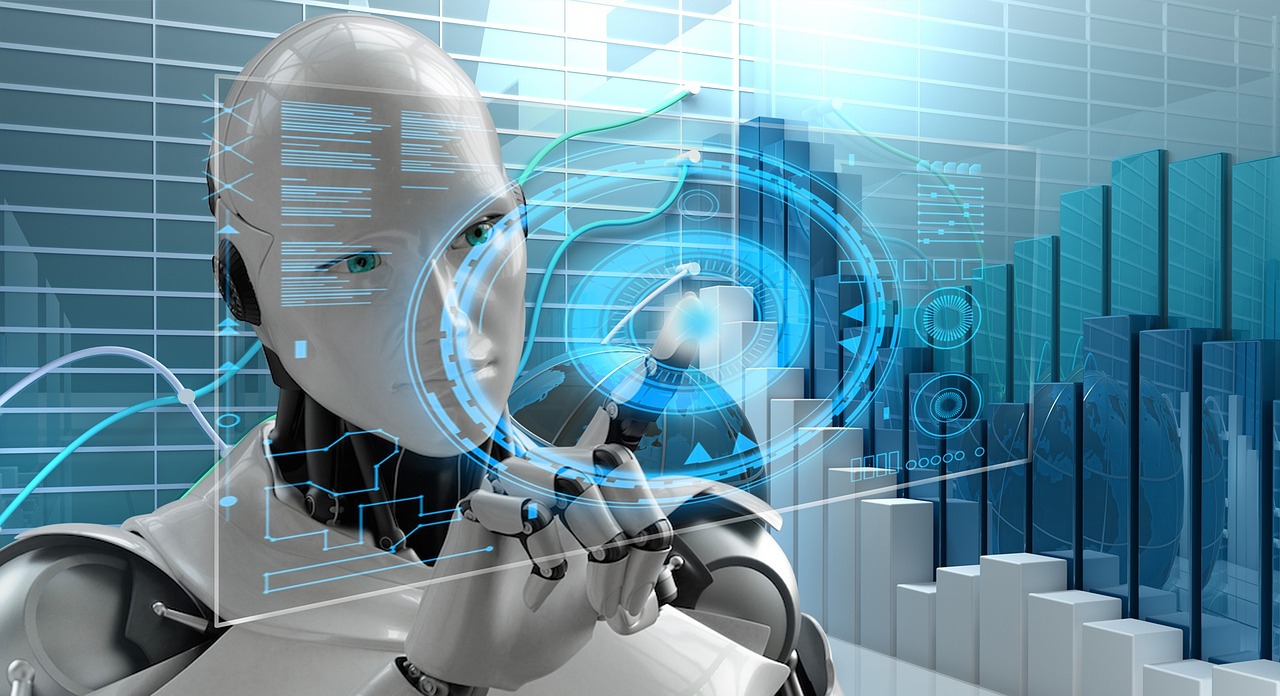
Bitbot’s twelfth presale level nears its finish after elevating $2.87 million
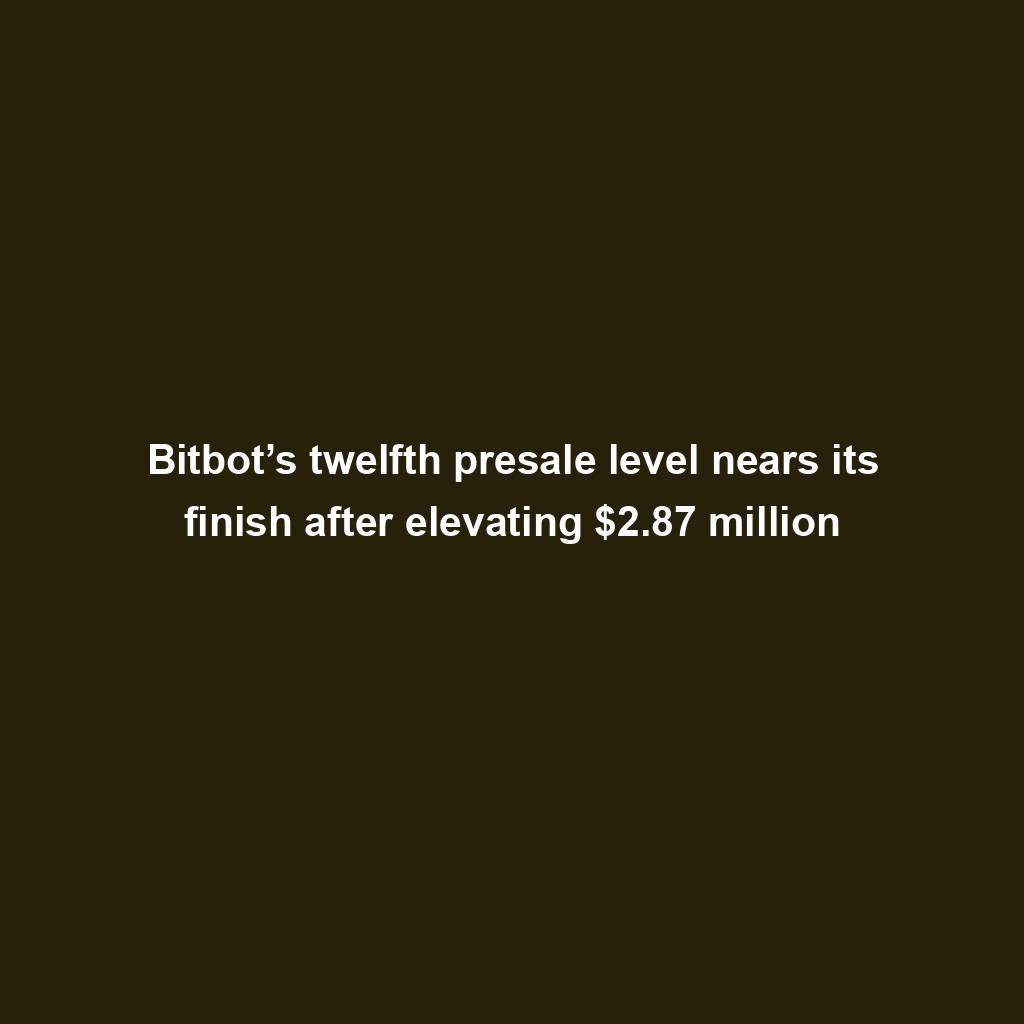
PANDA and MEW bullish momentum cool off: traders shift to new altcoin
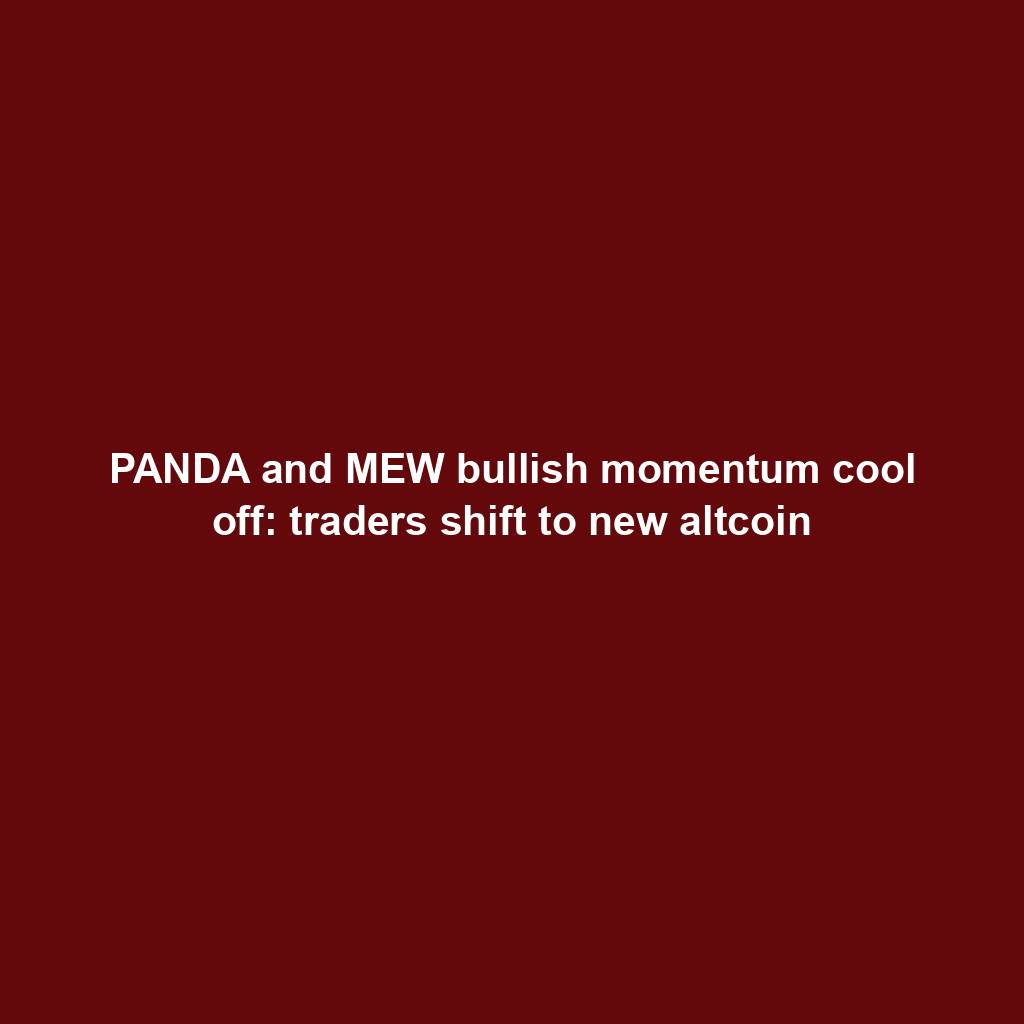
Commerce technique: Ecommerce is useless, lengthy are living ecommerce
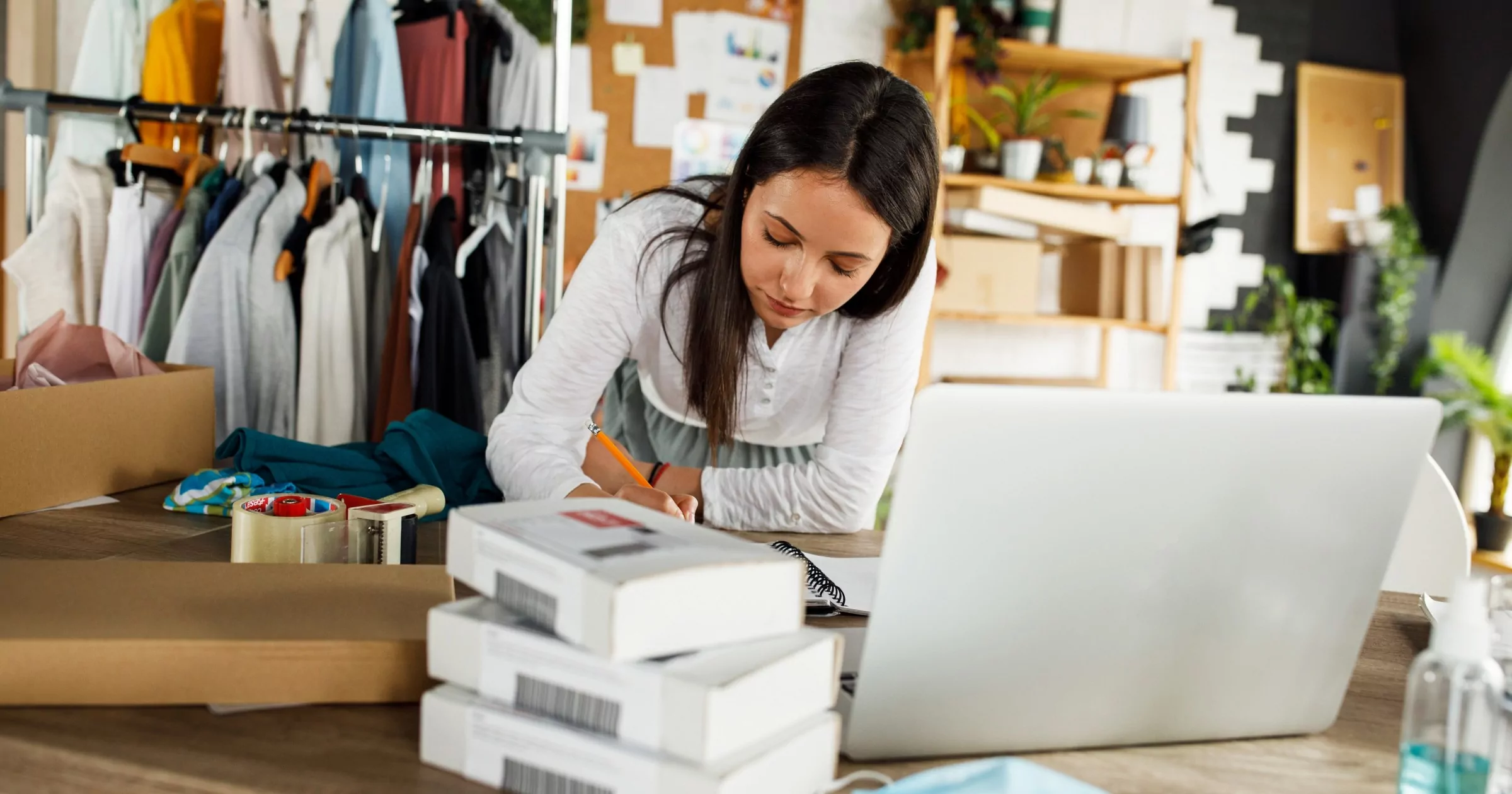
Republic First Bank closed by way of US regulators — crypto neighborhood reacts
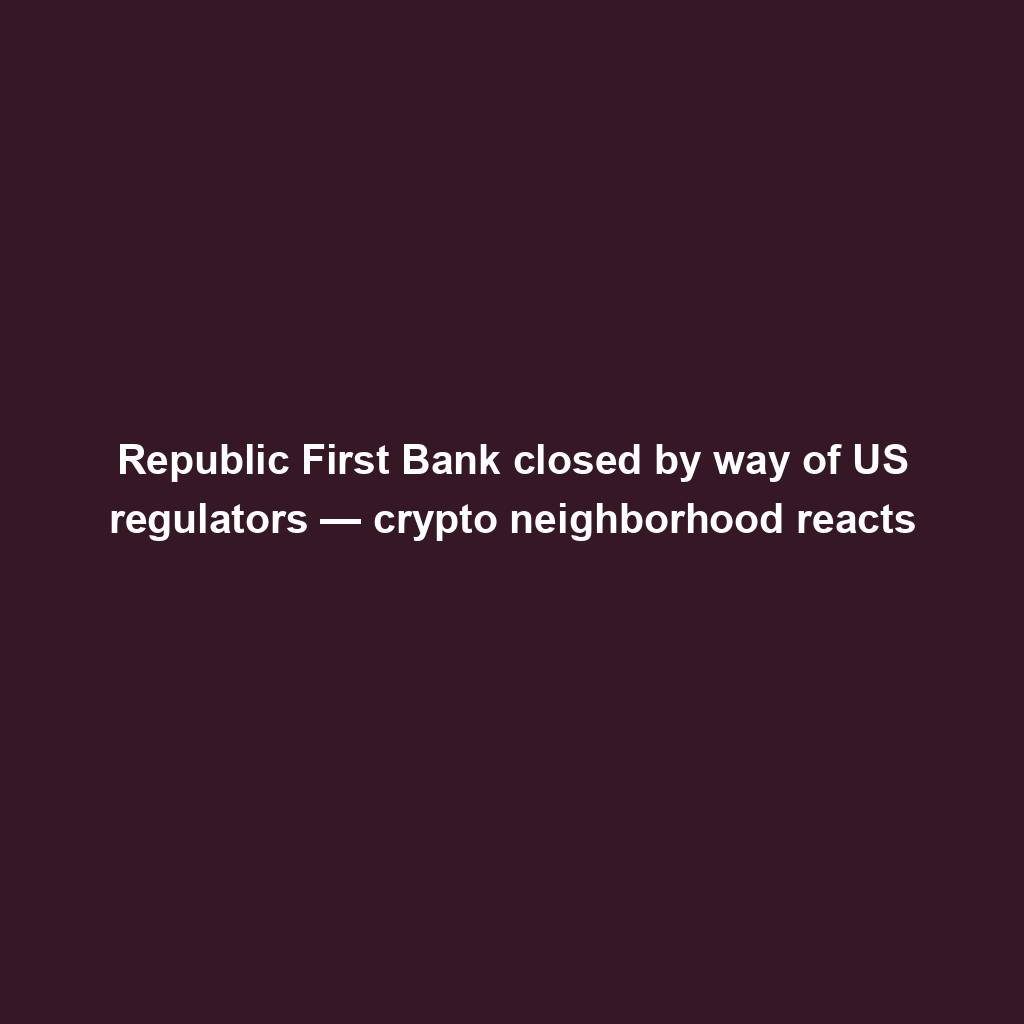
China’s former CBDC leader is beneath executive investigation
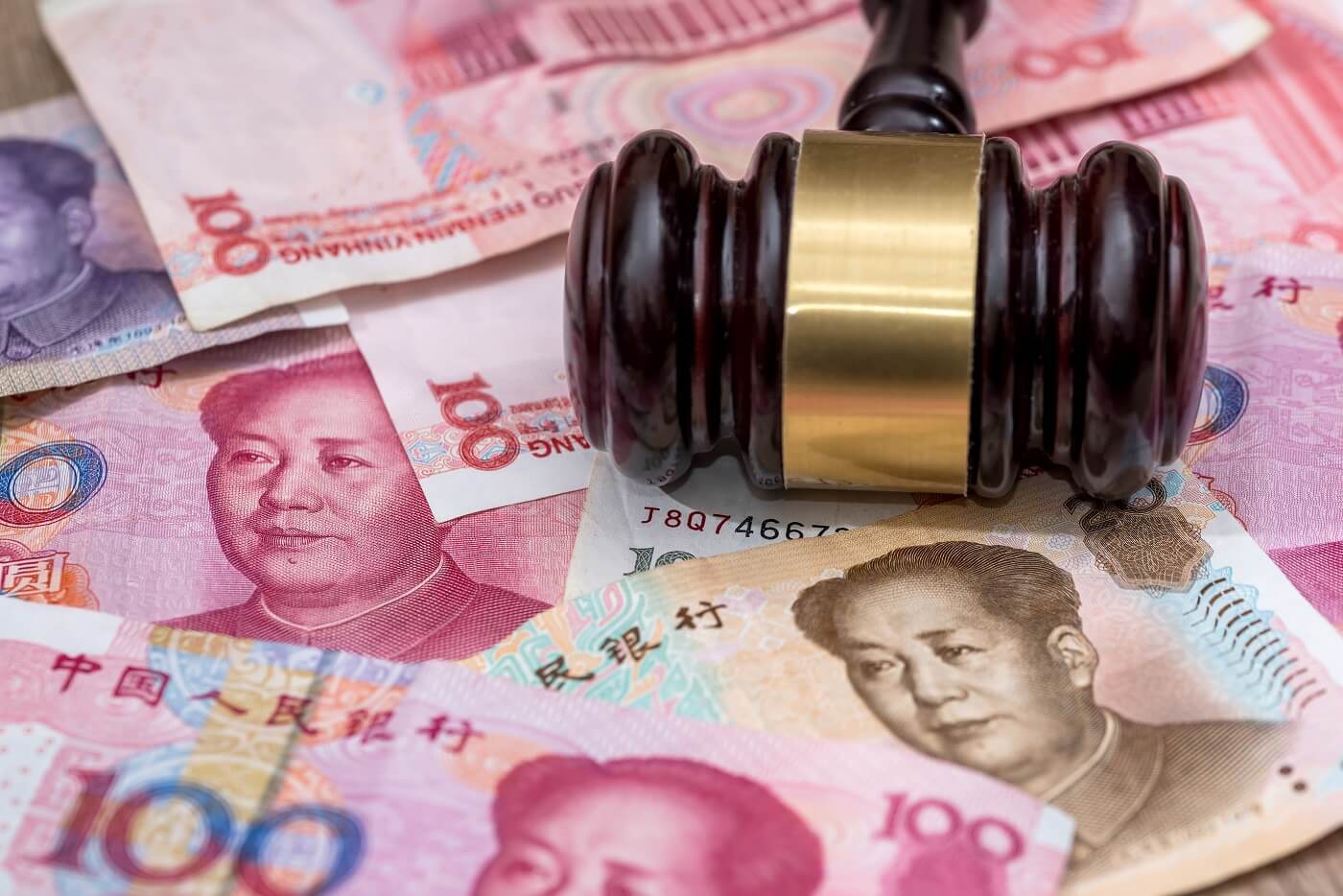
Bigger isn’t all the time higher: How hybrid Computational Intelligence development permits smaller language fashions
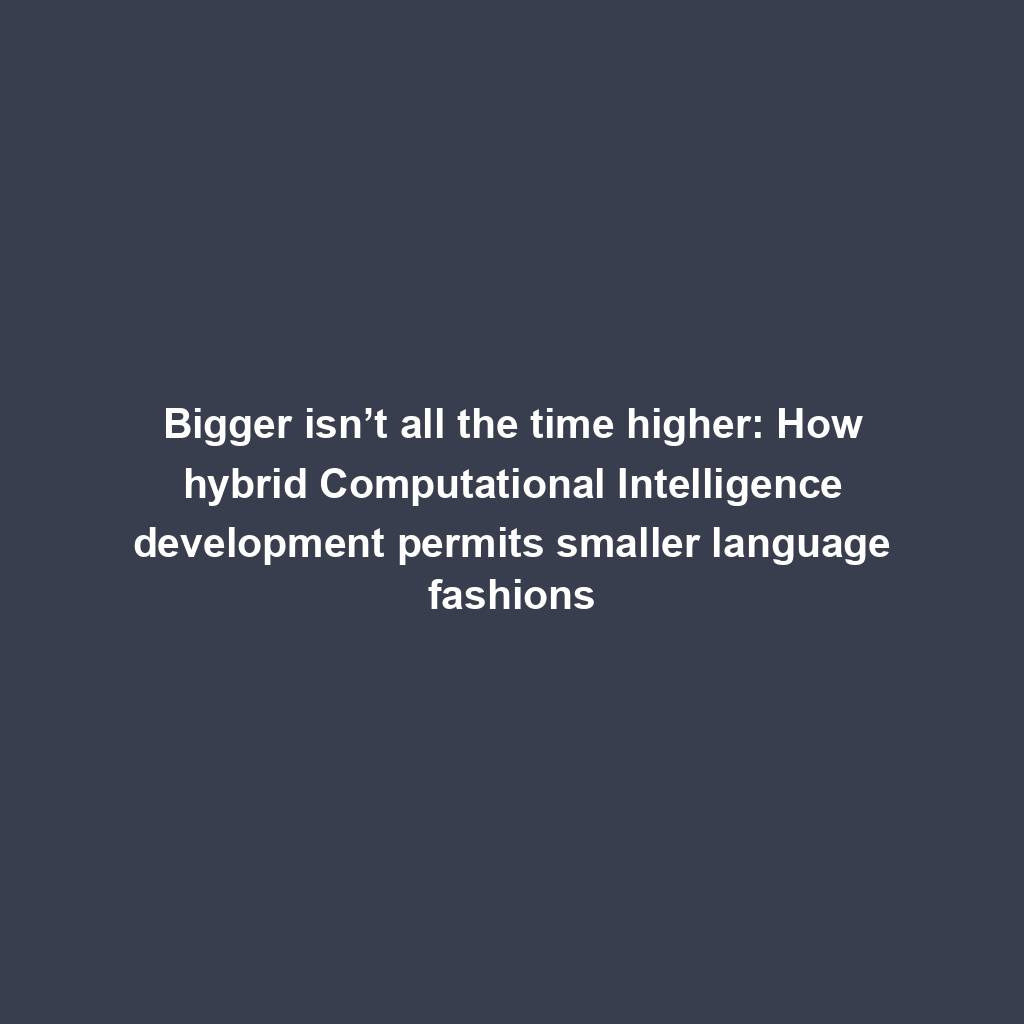
Pantera Capital buys extra Solana (SOL) from FTX
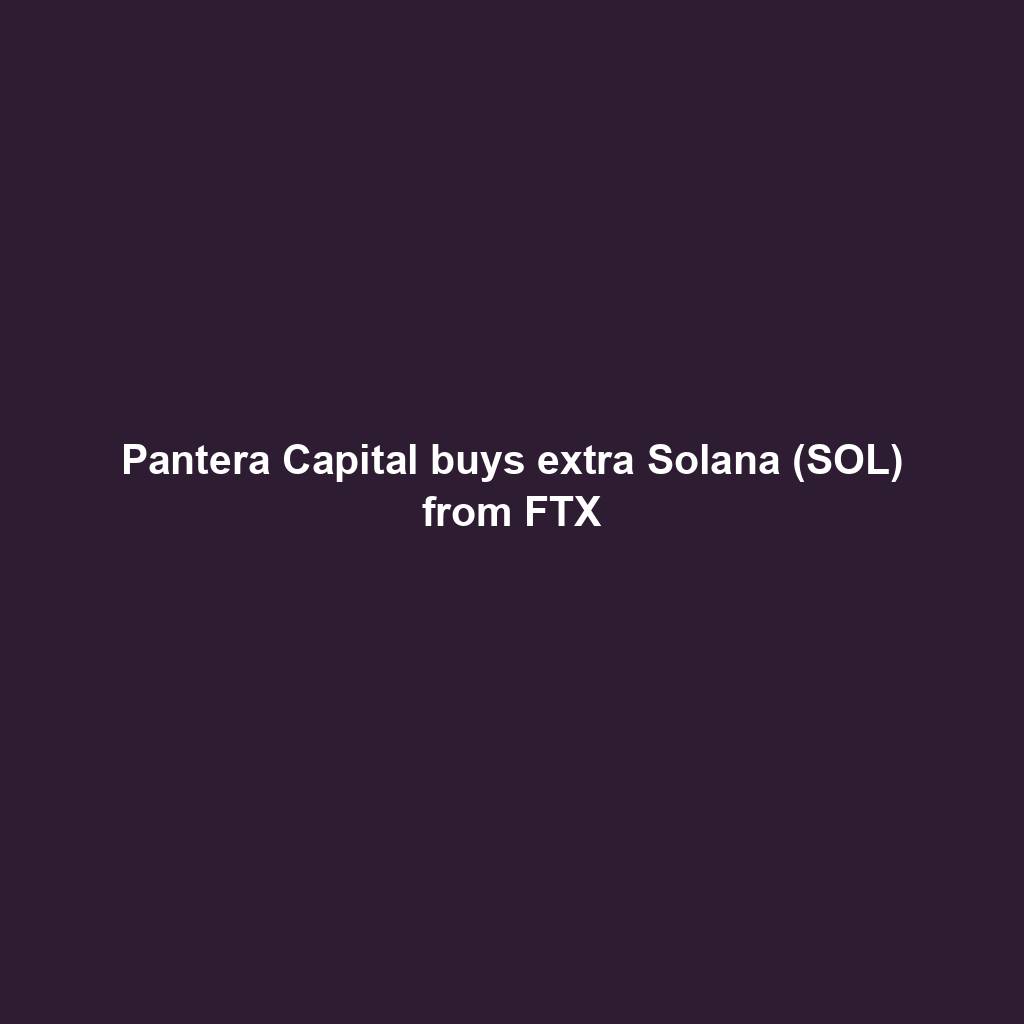
Successful Beta Service release of SOMESING, ‘My Hand-Carry Studio Karaoke App’

SEC sues Bitcoin miner Geosyn Mining for fraud; Bitbot presale nears $3M
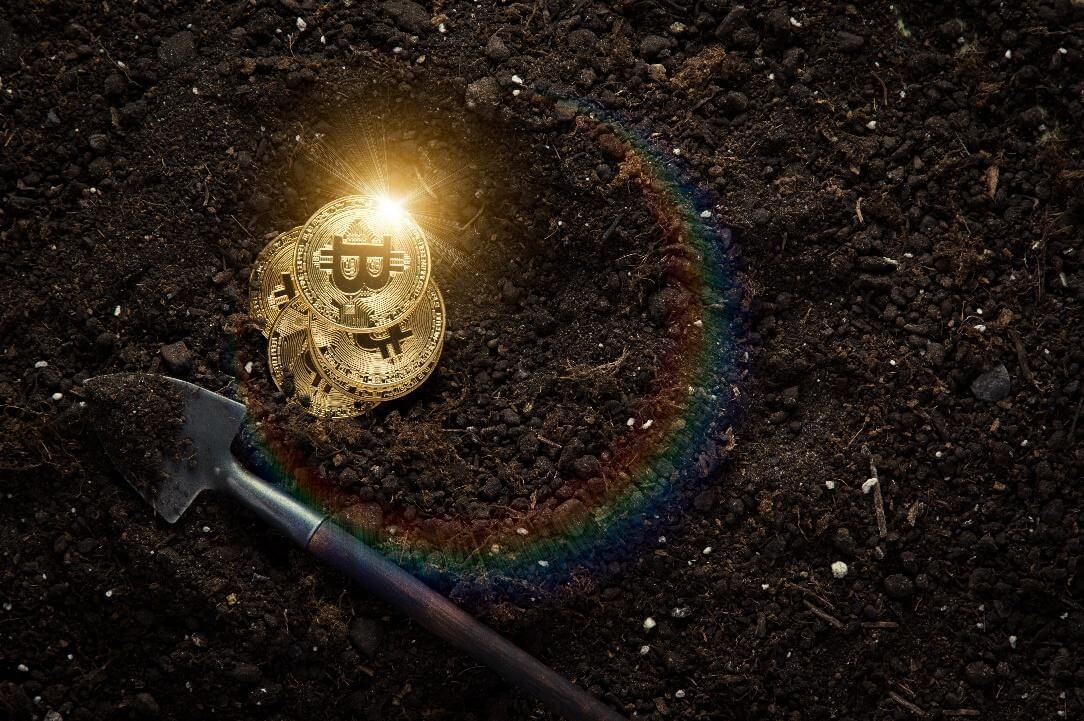
Business procedure reengineering (BPR) examples
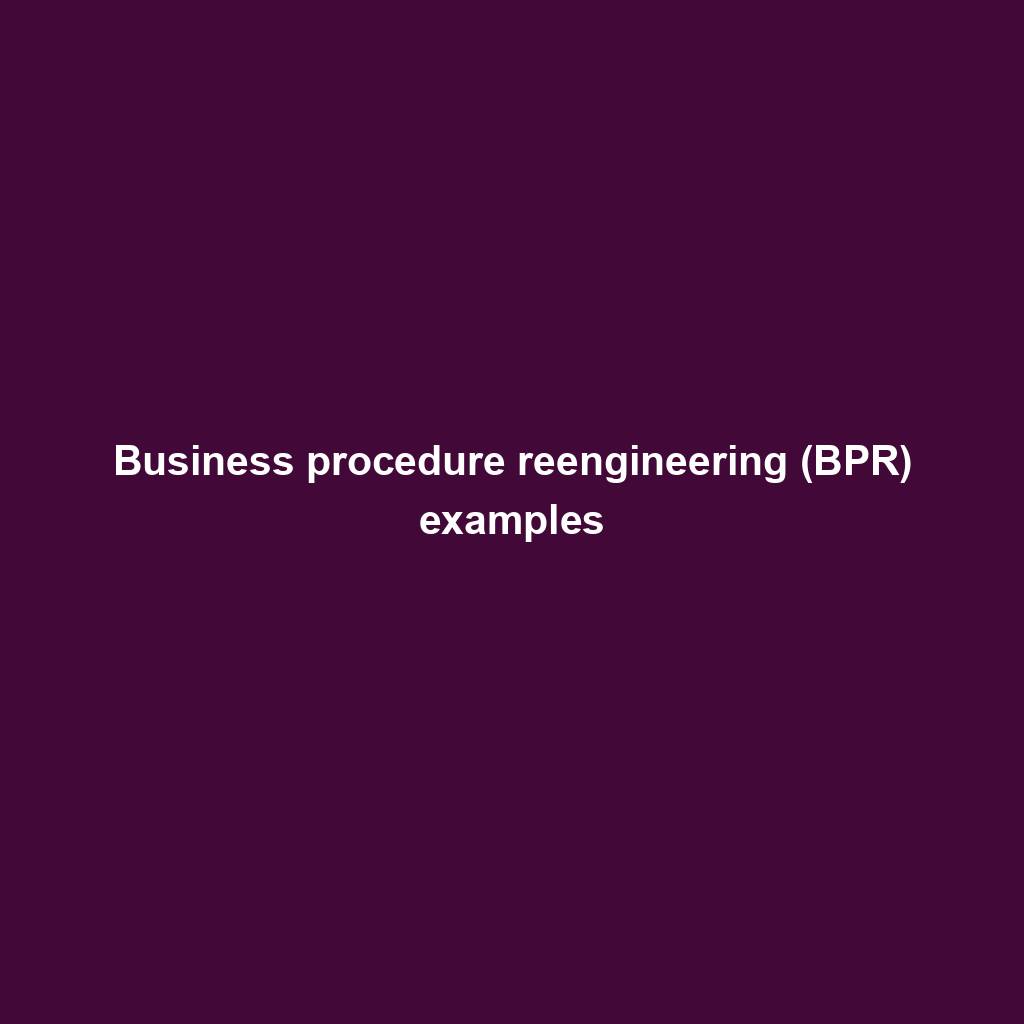
85% Of Altcoins In “Opportunity Zone,” Santiment Reveals
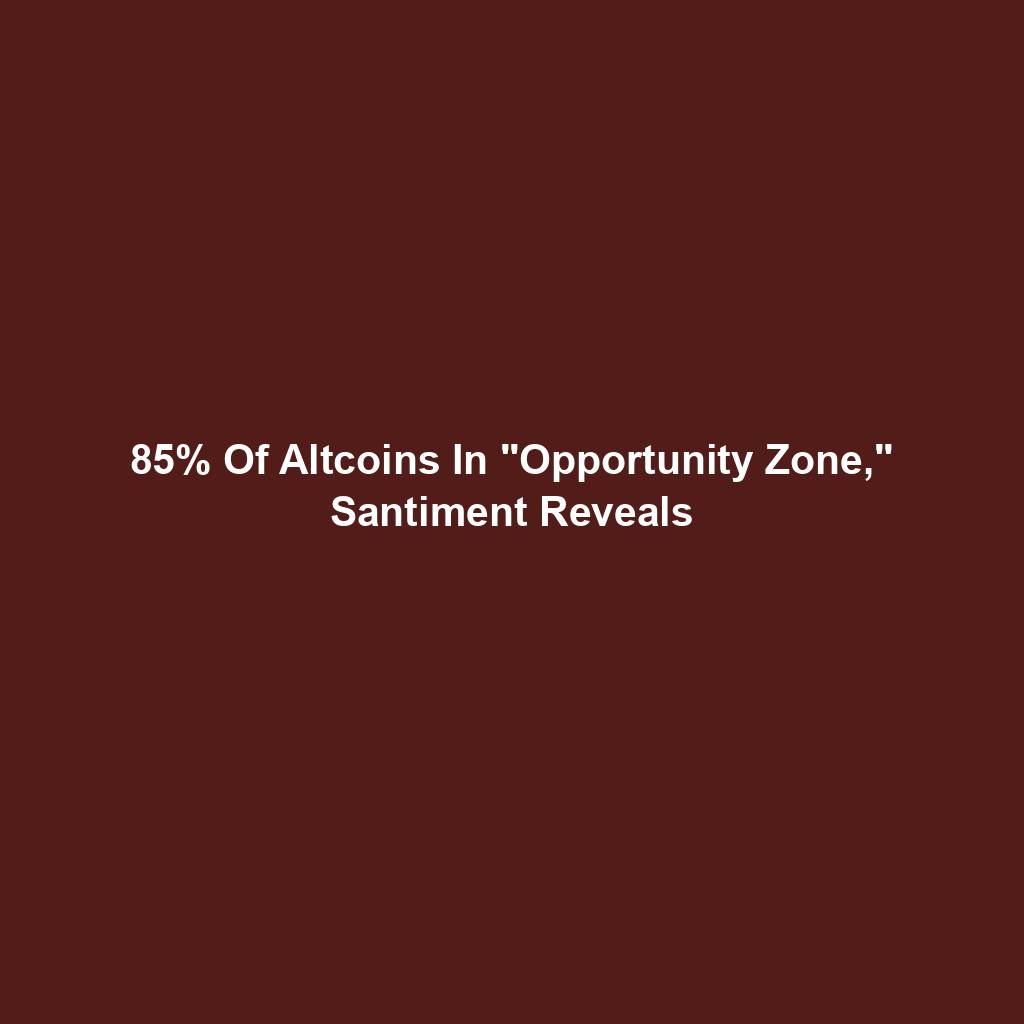
Sam Altman’s Worldcoin eyeing PayPal and OpenAI partnerships
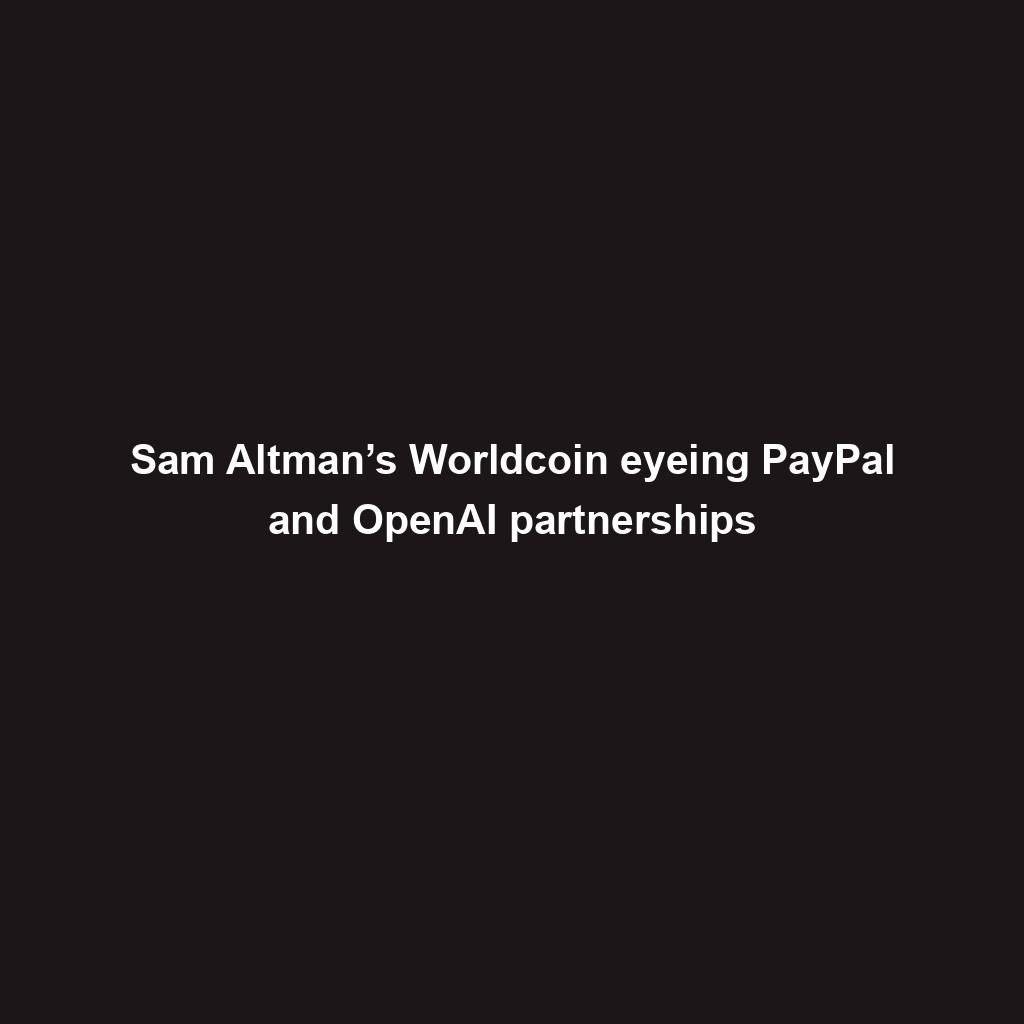
Artificial Intelligence transforms the IT strengthen enjoy
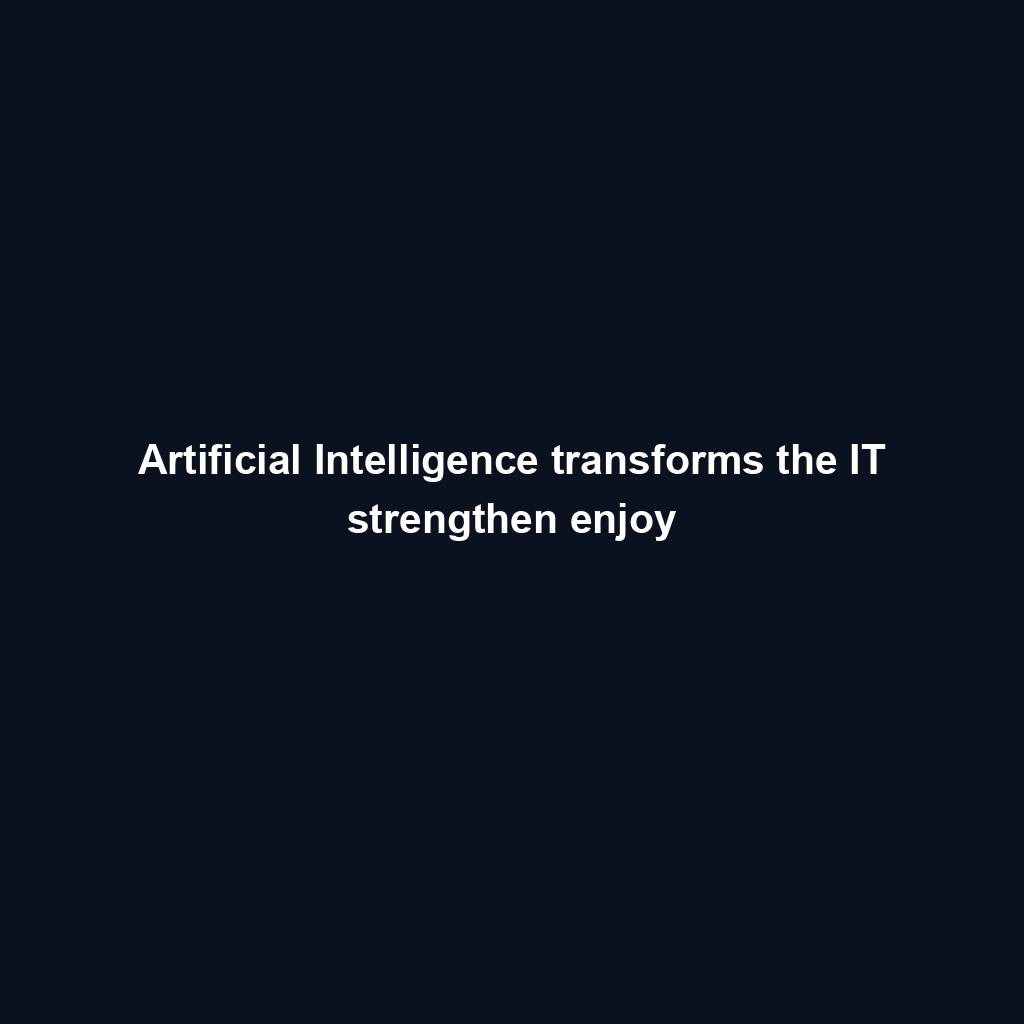
Franklin Templeton tokenizes $380M fund on Polygon and Stellar for P2P transfers
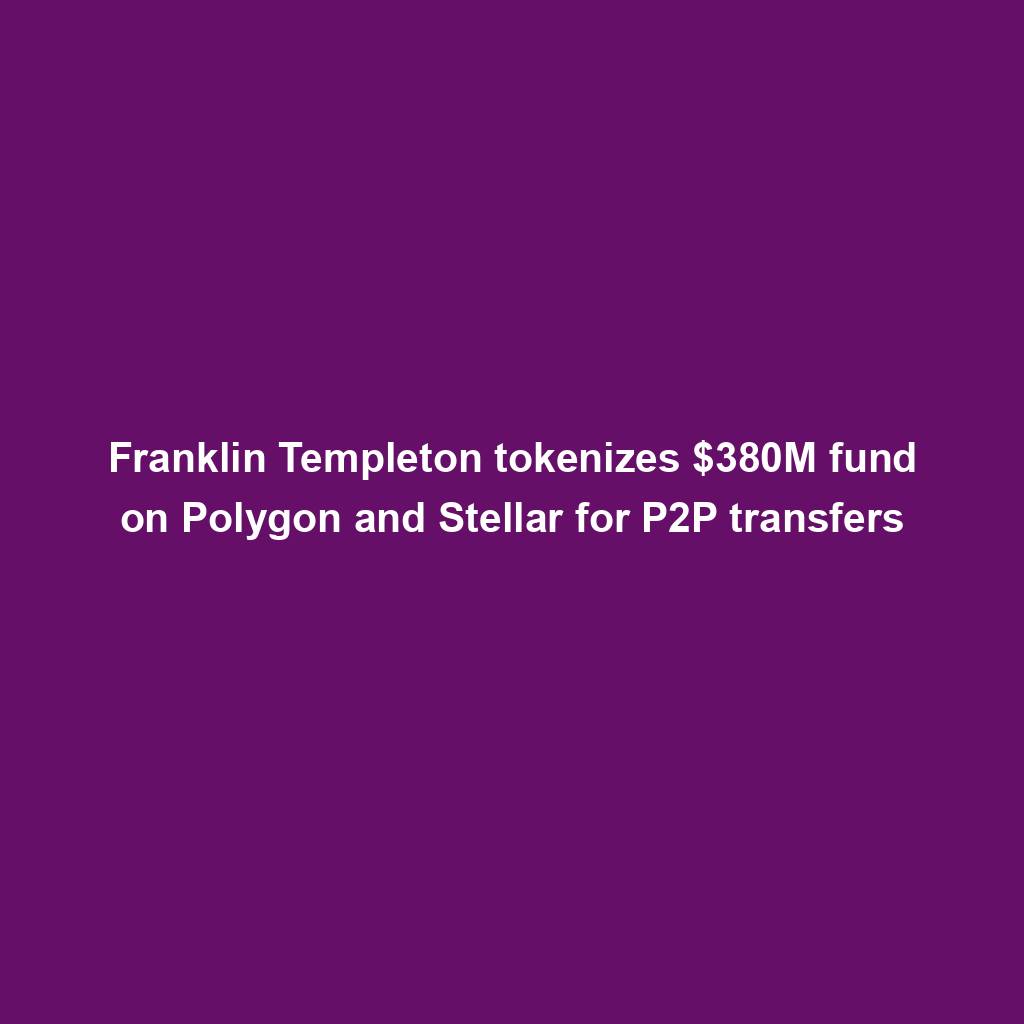
Meta’s letting Xbox, Lenovo, and Asus construct new Quest metaverse {hardware}
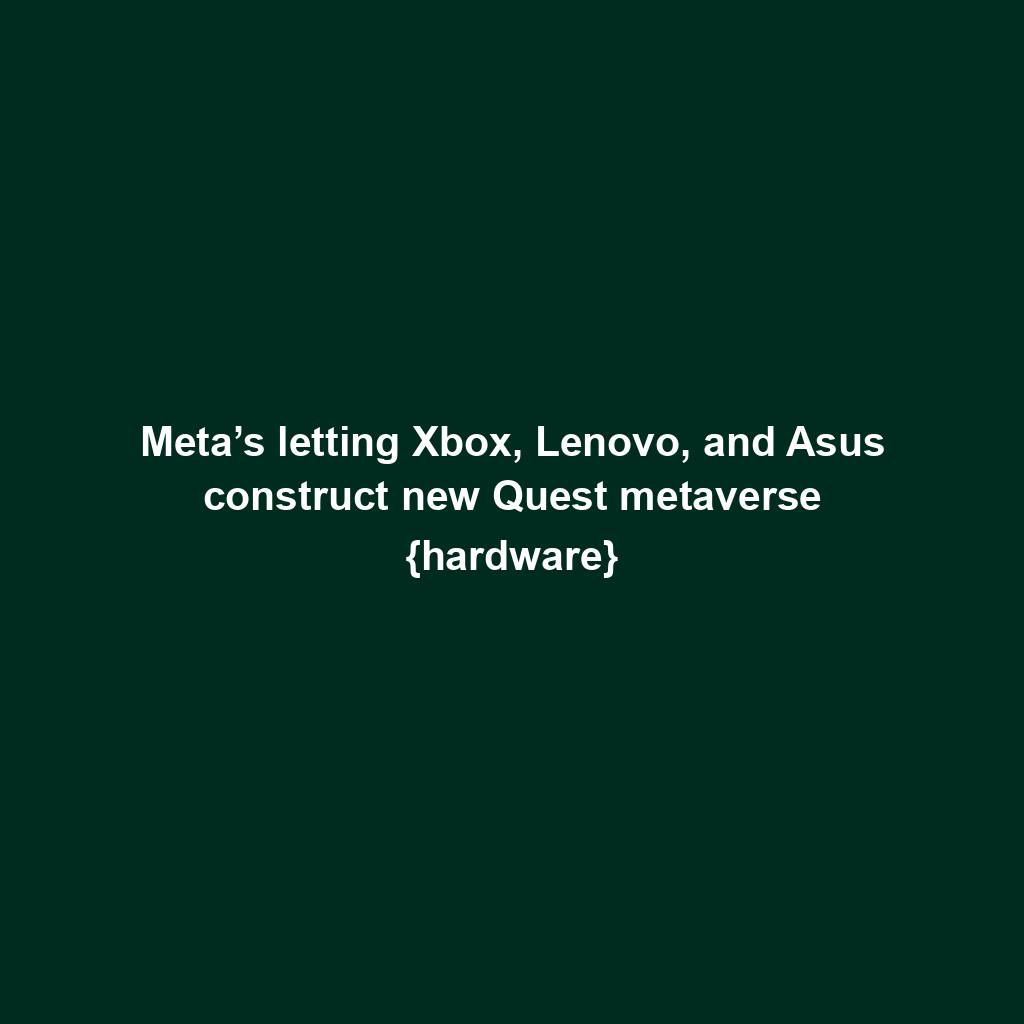
Shiba Inu (SHIB) unveils bold Shibarium plans as Kangamoon steals the display
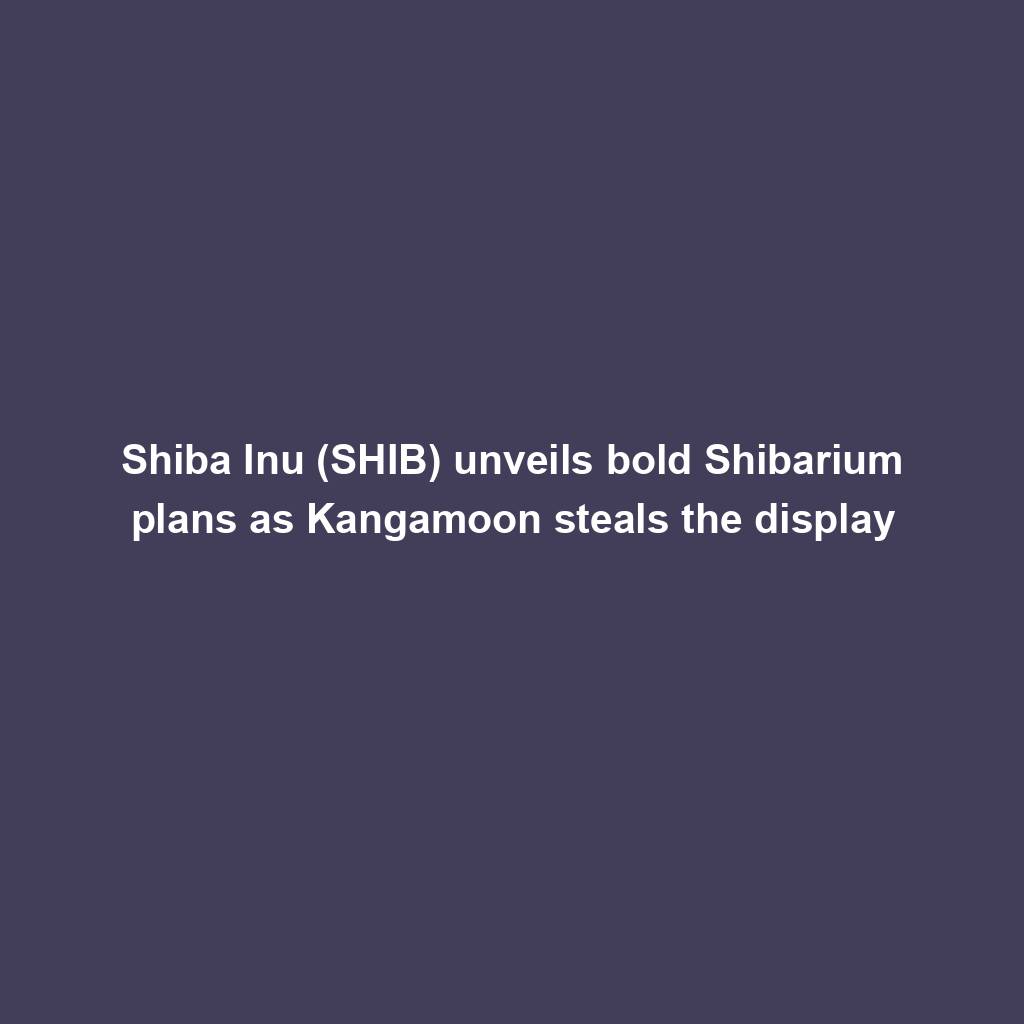