Generative AI technology is revolutionizing the world of marketing, enabling teams to deliver highly personalized communications at scale and meet the growing expectations of customers. A recent survey by IBM and Momentive.ai revealed that 67% of CMOs plan to implement generative AI within the next 12 months, with 86% planning to do so within 24 months. This powerful tool has the potential to transform the end-to-end marketing process, from internal communications to customer-facing channels and product support.
While AI has already proven its value in various marketing functions, generative AI solutions offer even greater potential for personalization and employee performance improvement. However, the adoption of generative AI requires organizations to address challenges such as managing implementation complexity, building the right data set, and mitigating brand and intellectual property risks.
Building an effective generative AI marketing strategy begins with the foundation of sound data. Just like any AI implementation, generative AI relies on high-quality data to produce accurate results. Data curation plays a crucial role in ensuring that content development and audience targeting are optimized. It is essential to set guardrails and implement supervision to address bias, maintain consistency of brand voice, and ensure the accuracy of product and service information.
To create a successful data-driven generative AI marketing strategy, it is necessary to define use cases in advance and align with IT to ensure a secure data architecture that protects intellectual property and confidential data. Additionally, ongoing human supervision is vital to refine foundation models, align generative AI output with human intentions, and address ethical and legal concerns related to data use.
The top B2B use cases for generative AI, as cited by CMOs in the IBM survey, include content creation and editing, SEO, social media marketing, lead generation, and sales nurturing. However, leaders expressed concerns about data accuracy, privacy management, and the availability of skilled resources to build generative AI solutions. To address these concerns, adopting a practical approach that prioritizes building, testing, and learning about generative AI capabilities is crucial.
To support marketing organizations in implementing generative AI responsibly and effectively, IBM offers Watsonx, an enterprise-ready AI and data platform. Watsonx includes watsonx.ai, an enterprise studio for training and deploying generative AI models; watsonx.data, an open hybrid data store for scaling generative AI workloads; and watsonx.governance, a toolkit that ensures responsible and transparent AI workflows.
IBM Consulting™ and its team of over 20,000 AI experts globally empower marketing organizations to design and scale AI and automation solutions with confidence. Leveraging the expertise of the IBM watsonx technology and its open ecosystem of partners, IBM Consulting™ is committed to delivering AI models on any cloud, guided by ethics and trust.
By leveraging the right data sources and architecture, organizations can take the first steps towards harnessing the power of generative AI in their marketing strategies.
FAQ
What is generative AI?
Generative AI is a technology that enables the creation of creative and customized communications, allowing marketing teams to deliver highly personalized content at scale.
Why is data important in generative AI?
Data is essential in generative AI as high-quality data is crucial for producing accurate and reliable results. If the training data is biased or incomplete, the models may generate inaccurate content.
What are the challenges in adopting generative AI for marketing?
According to a survey by IBM and Momentive.ai, the primary challenges in adopting generative AI for marketing include managing the complexity of implementation, building the right data set, and mitigating brand and intellectual property risks.
How can organizations create an effective generative AI marketing strategy?
To create an effective generative AI marketing strategy, organizations should focus on sourcing and maintaining high-quality data, defining use cases in advance, aligning with IT to ensure a secure data architecture, and implementing ongoing human supervision to align generative AI output with human intentions.
What are the top B2B use cases for generative AI?
According to the IBM survey, the top B2B use cases for generative AI capabilities include content creation and editing, SEO, social media marketing, lead generation, and sales nurturing.
Summary
Generative AI is transforming the marketing landscape, enabling teams to deliver highly personalized communications at scale. However, adopting generative AI requires organizations to address challenges such as managing implementation complexity, building the right data set, and mitigating brand and intellectual property risks. By leveraging the right data sources and architecture, organizations can create effective generative AI marketing strategies that deliver personalized experiences while protecting their brand and ensuring data accuracy.
Source: IBM Research
More in this category ...
Ripple companions with SBI Group and HashKey DX for XRPL answers in Japan

April sees $25M in exploits and scams, marking historic low ― Certik

MSTR, COIN, RIOT and different crypto shares down as Bitcoin dips

EigenLayer publicizes token release and airdrop for the group

VeloxCon 2024: Innovation in knowledge control
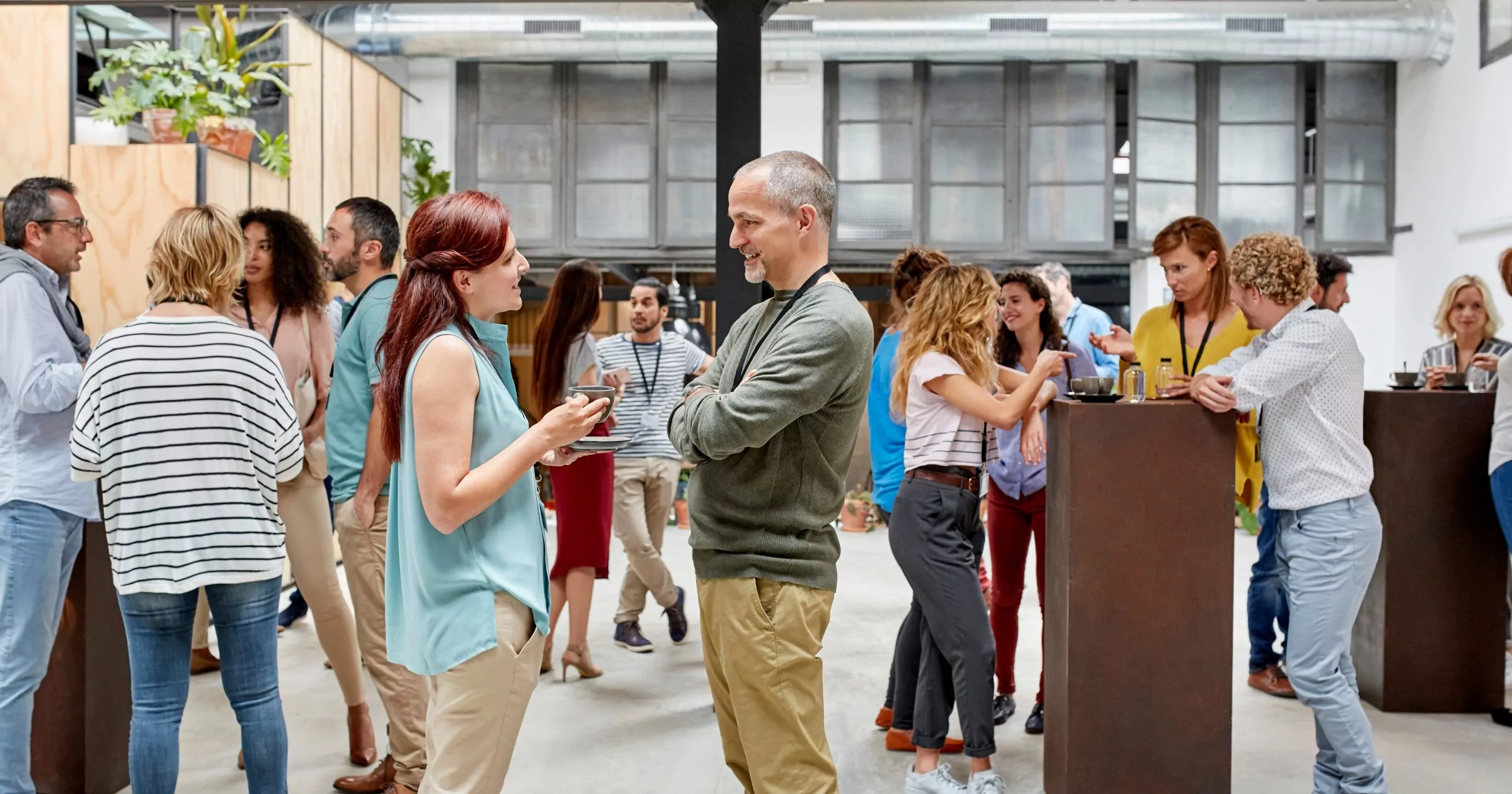
Successful Beta Service release of SOMESING, ‘My Hand-Carry Studio Karaoke App’

Dogwifhat (WIF) large pump on Bybit after record reasons marketplace frenzy

How fintech innovation is riding virtual transformation for communities around the globe

Wasabi Wallet developer bars U.S. customers amidst regulatory considerations

Analyst Foresees Peak In Late 2025
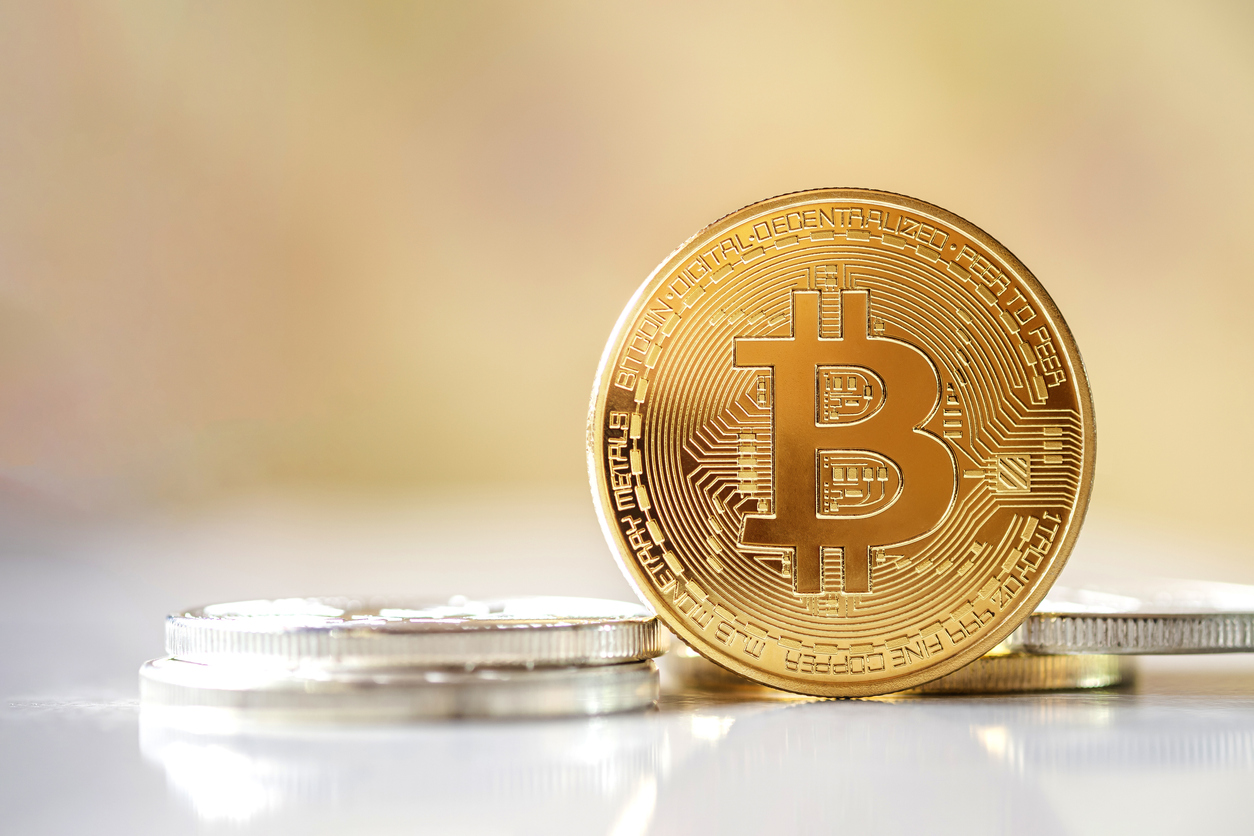
Solo Bitcoin miner wins the three.125 BTC lottery, fixing legitimate block
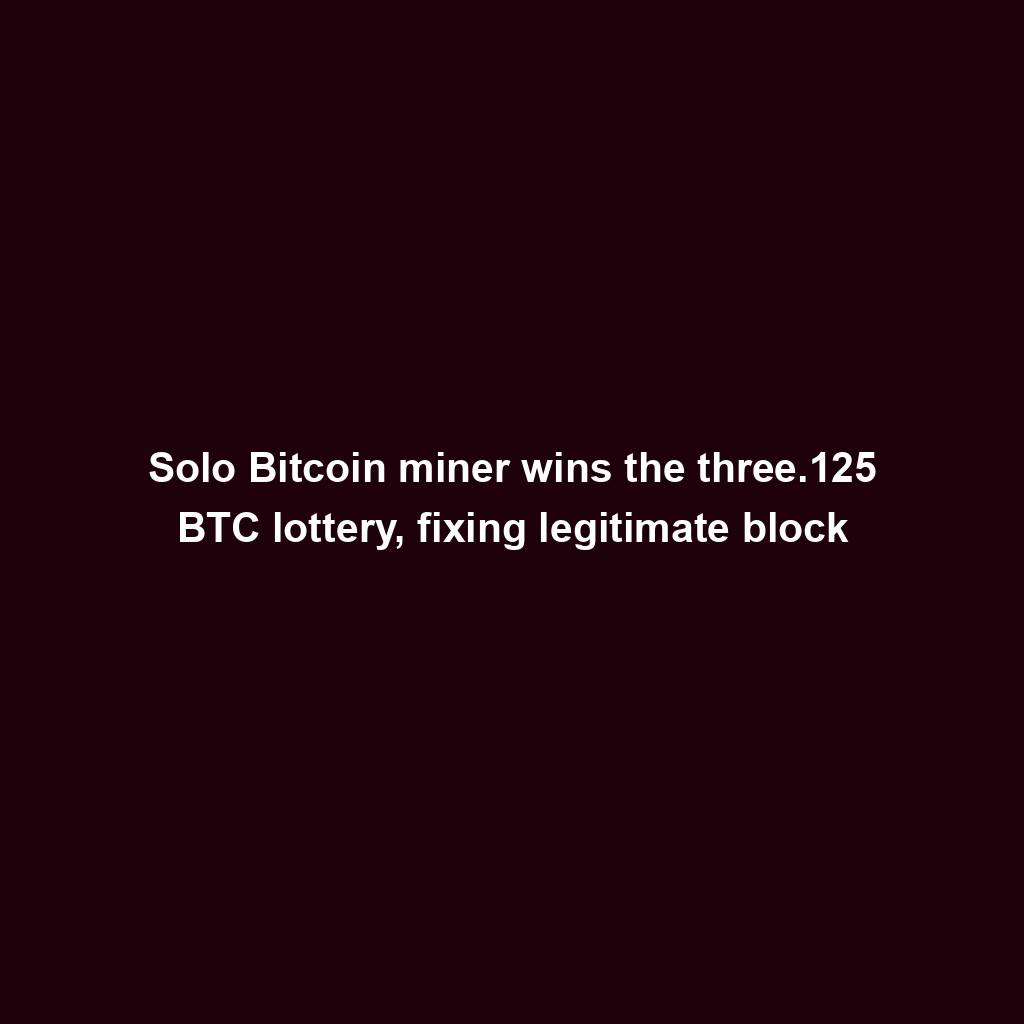
Ace Exchange Suspects Should Get 20-Year Prison Sentences: Prosecutors
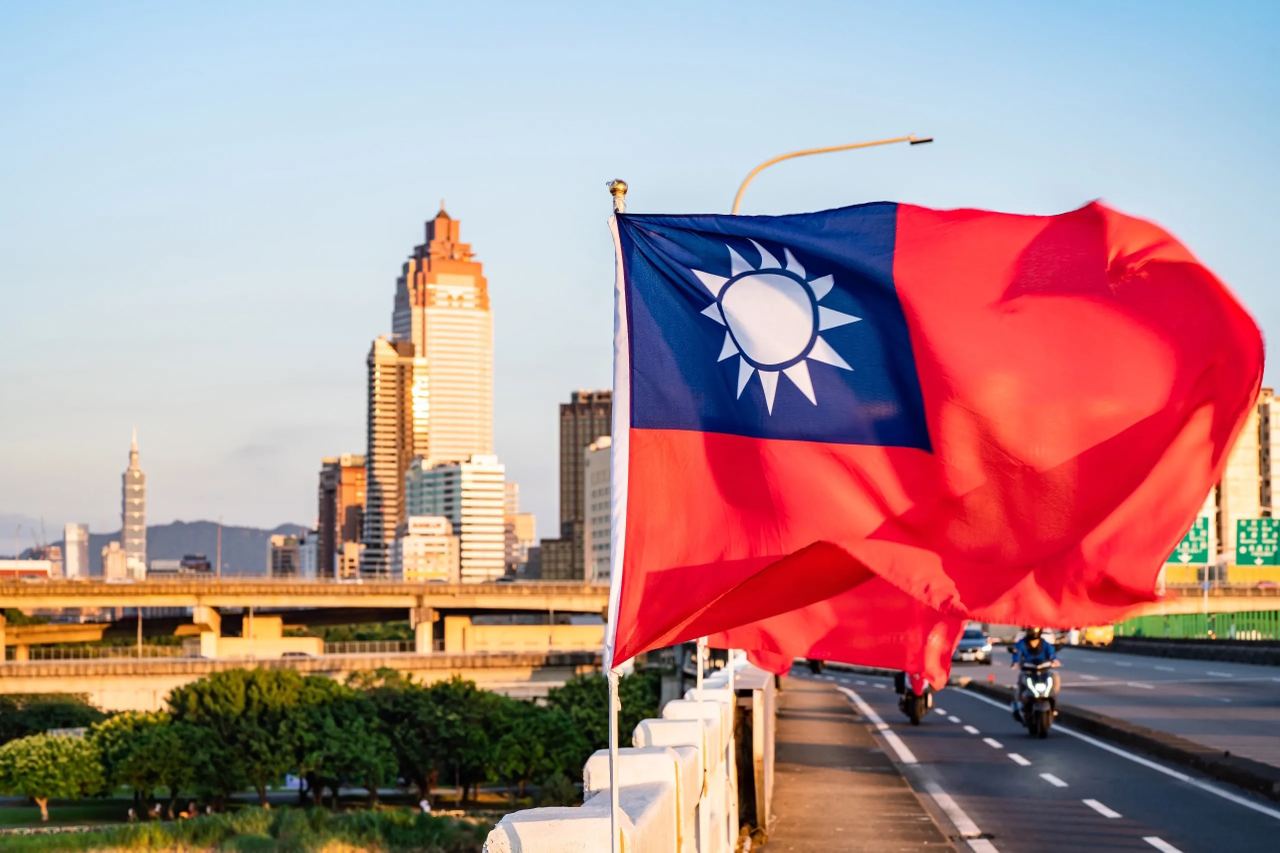
Google Cloud's Web3 portal release sparks debate in crypto trade
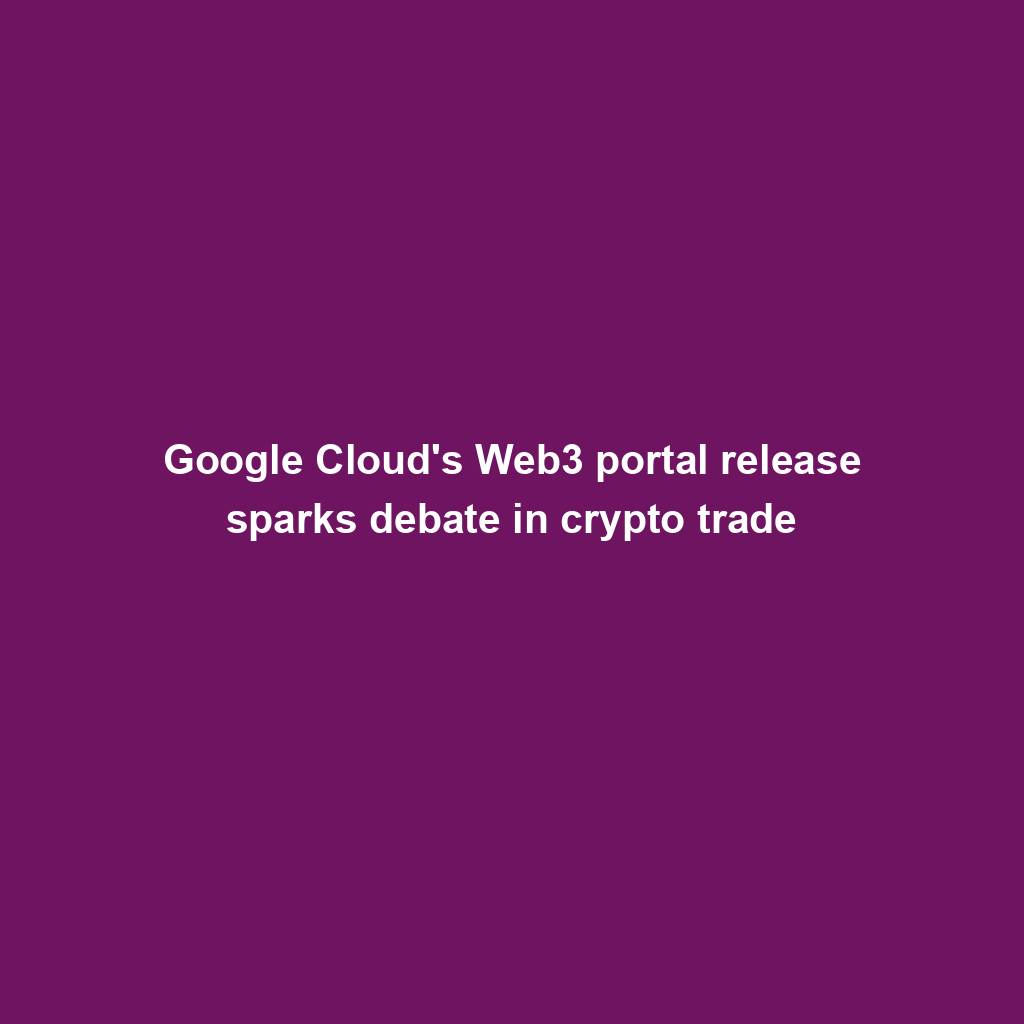
Bitcoin Primed For $77,000 Surge
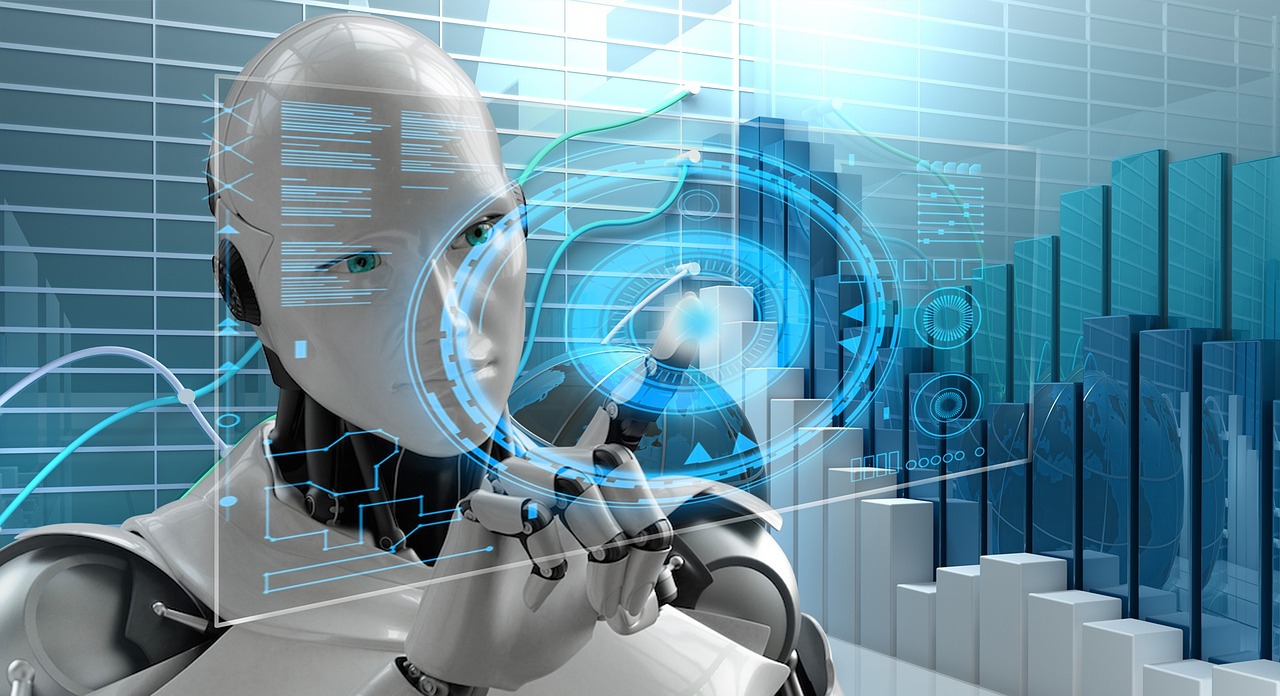
Bitbot’s twelfth presale level nears its finish after elevating $2.87 million
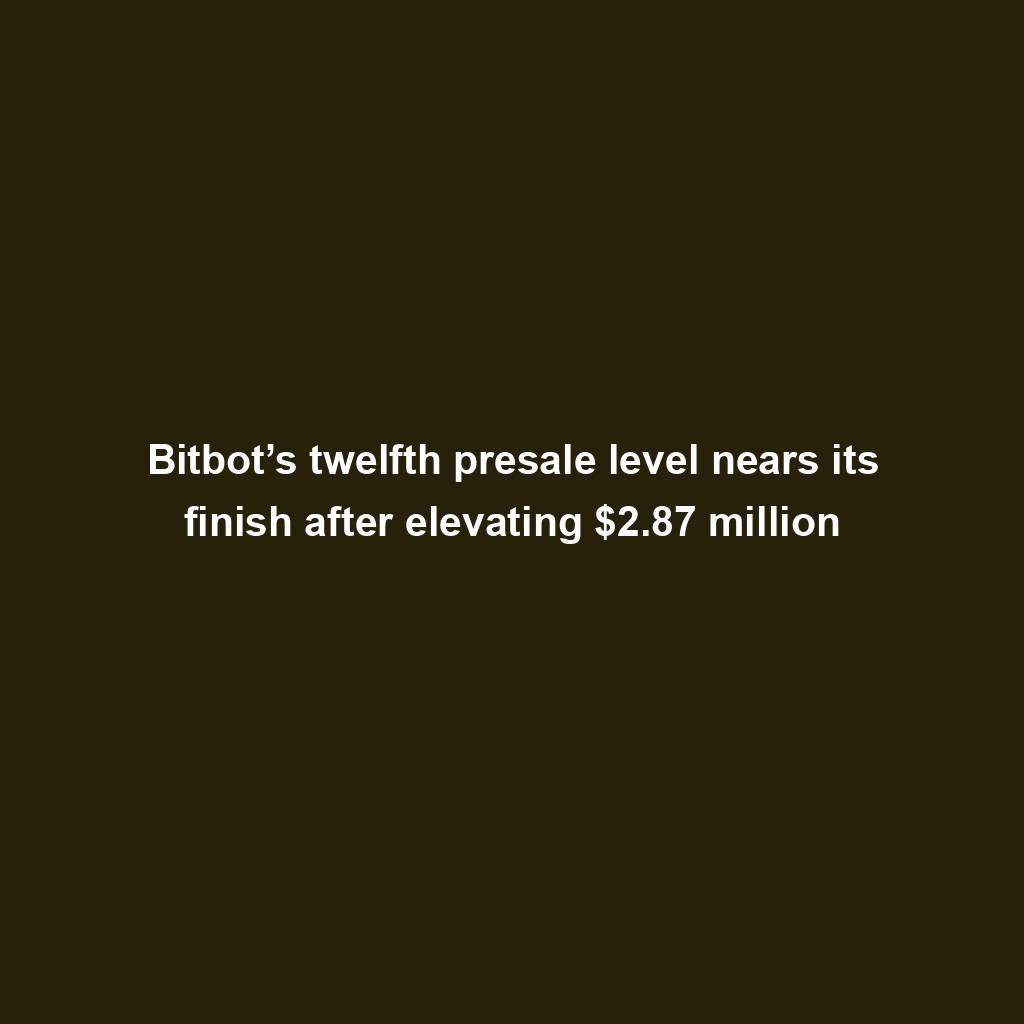
PANDA and MEW bullish momentum cool off: traders shift to new altcoin
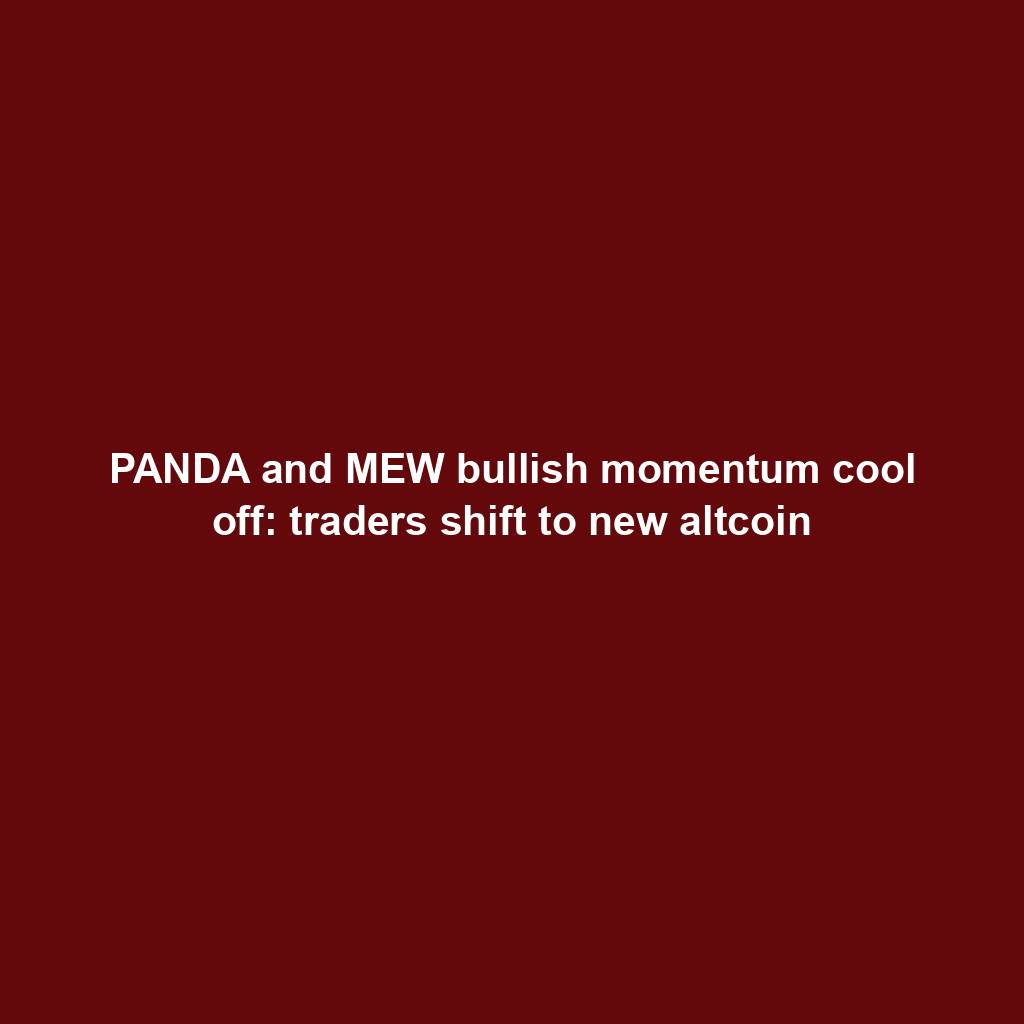
Commerce technique: Ecommerce is useless, lengthy are living ecommerce
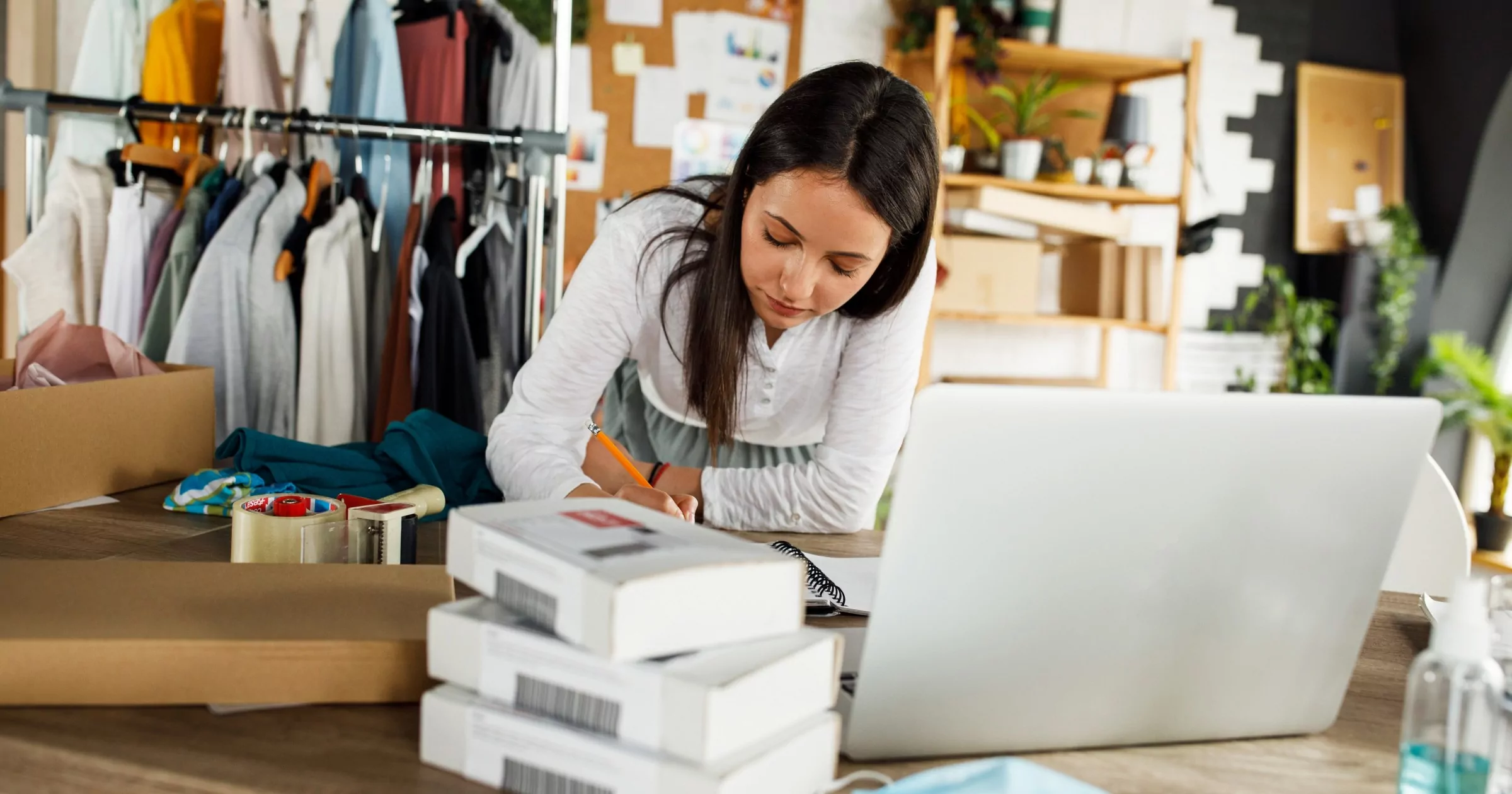
Republic First Bank closed by way of US regulators — crypto neighborhood reacts
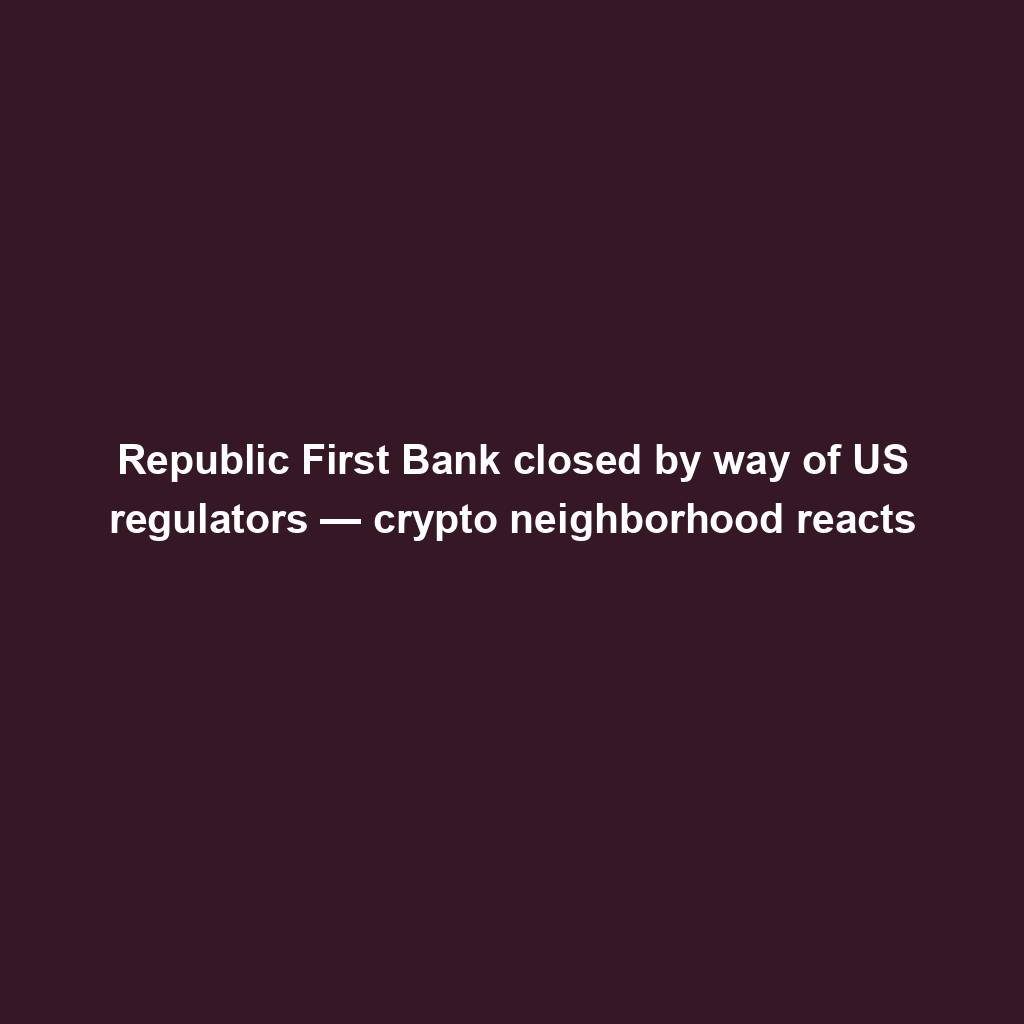
China’s former CBDC leader is beneath executive investigation
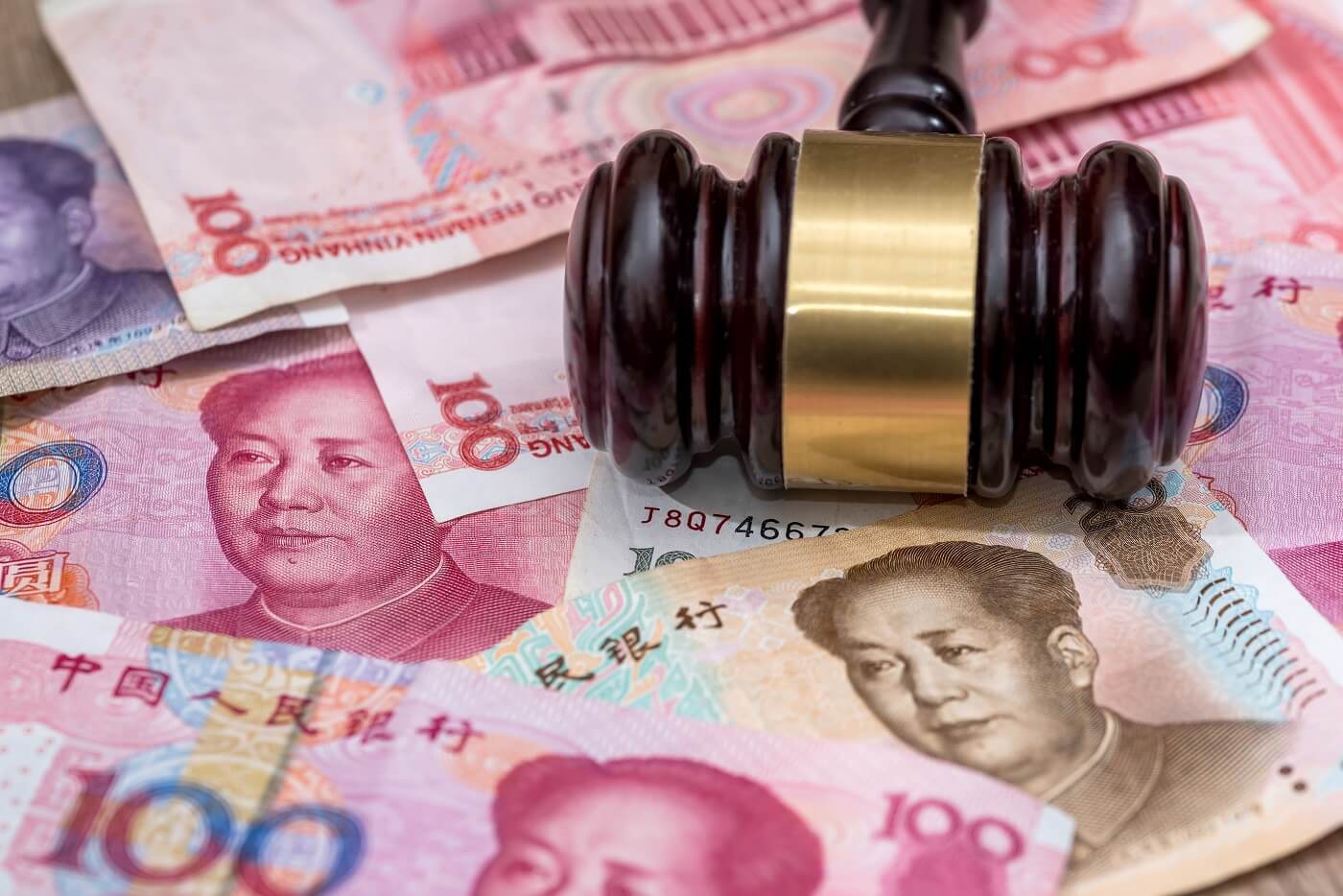
Bigger isn’t all the time higher: How hybrid Computational Intelligence development permits smaller language fashions
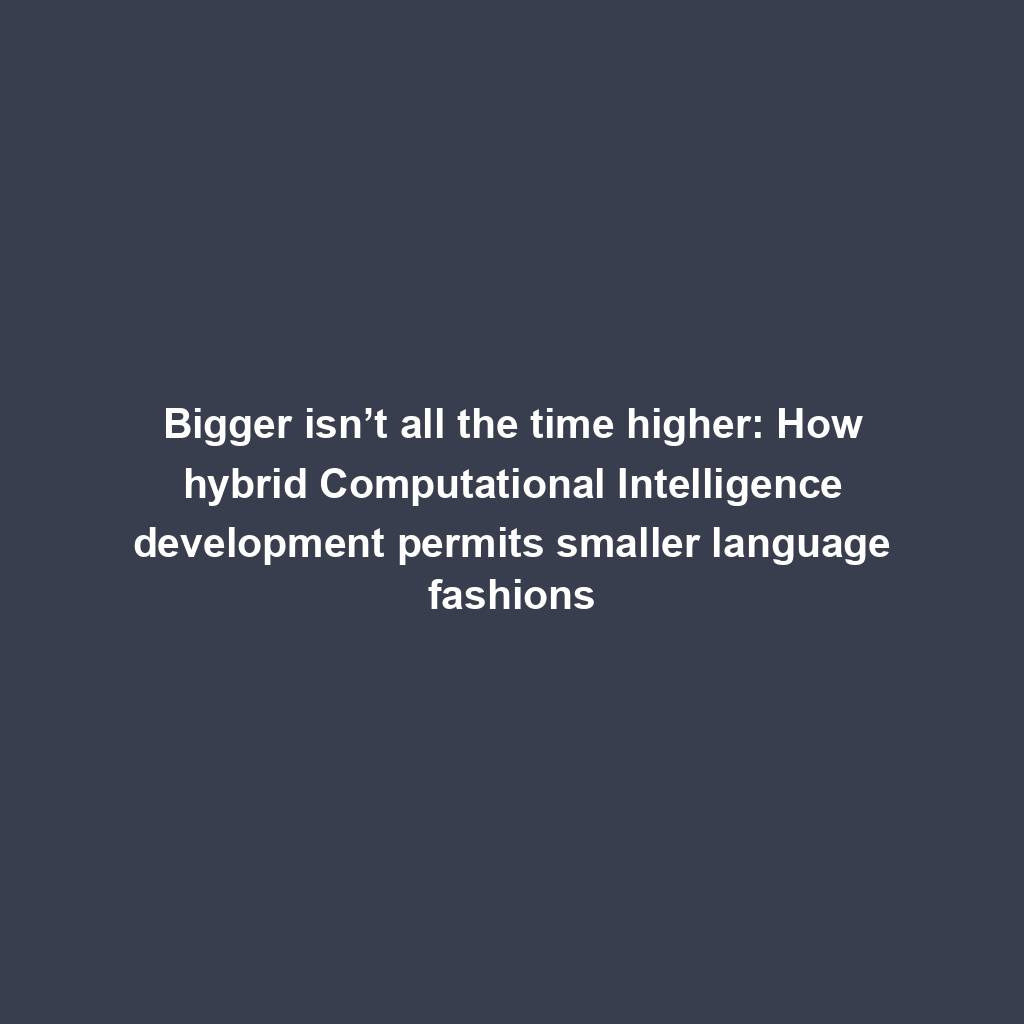
Pantera Capital buys extra Solana (SOL) from FTX
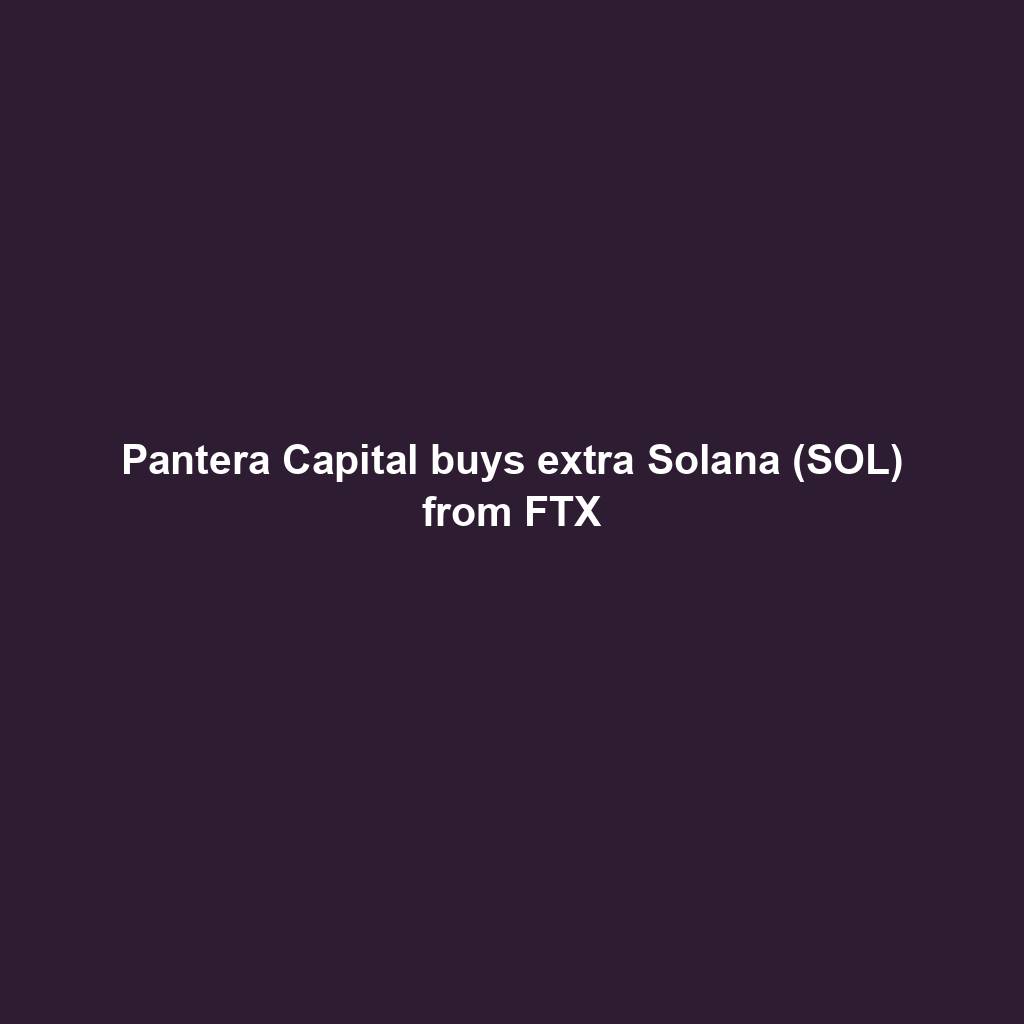
Successful Beta Service release of SOMESING, ‘My Hand-Carry Studio Karaoke App’

SEC sues Bitcoin miner Geosyn Mining for fraud; Bitbot presale nears $3M
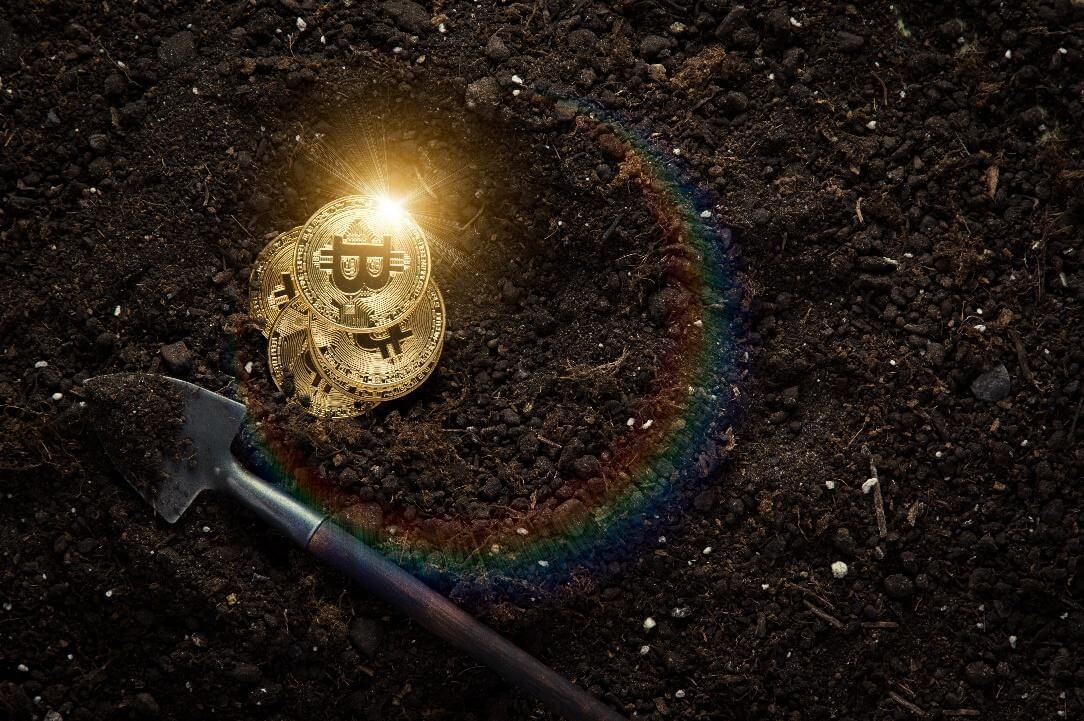
Business procedure reengineering (BPR) examples
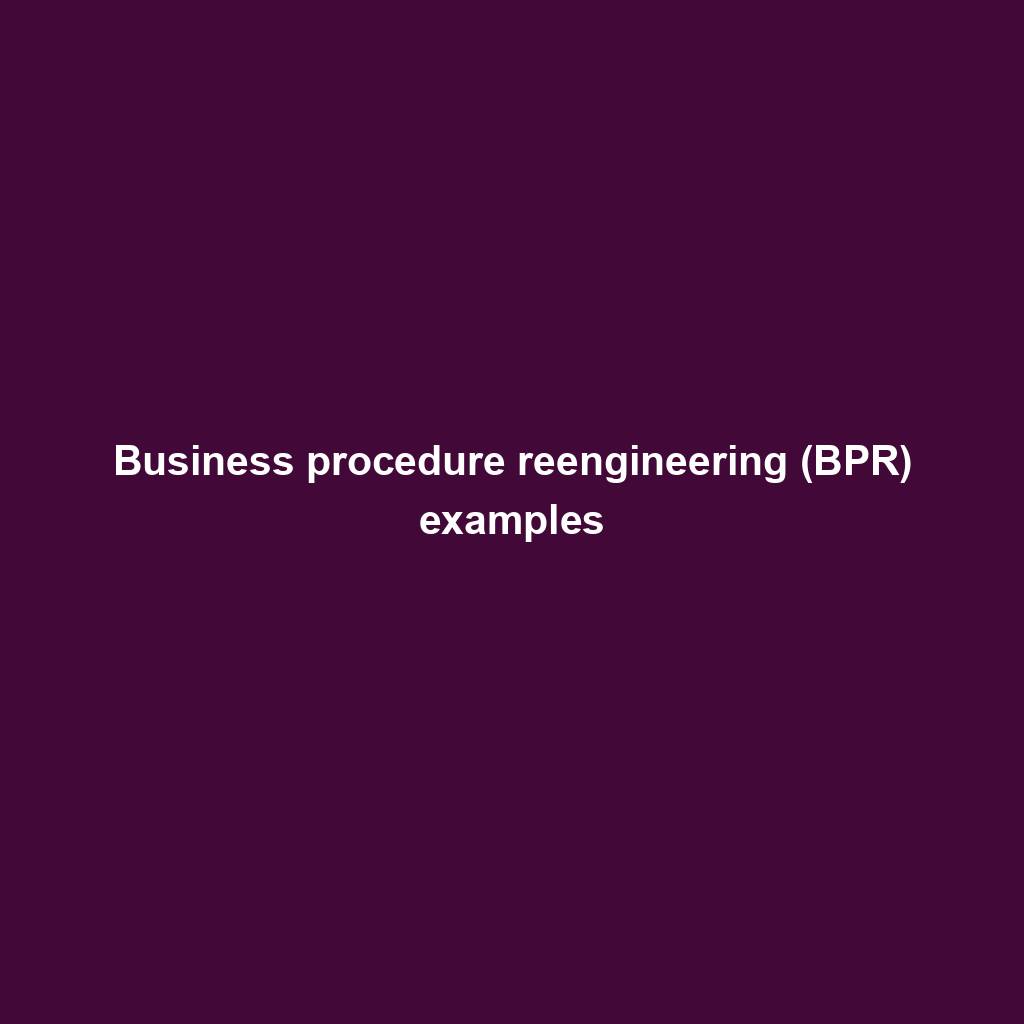
85% Of Altcoins In “Opportunity Zone,” Santiment Reveals
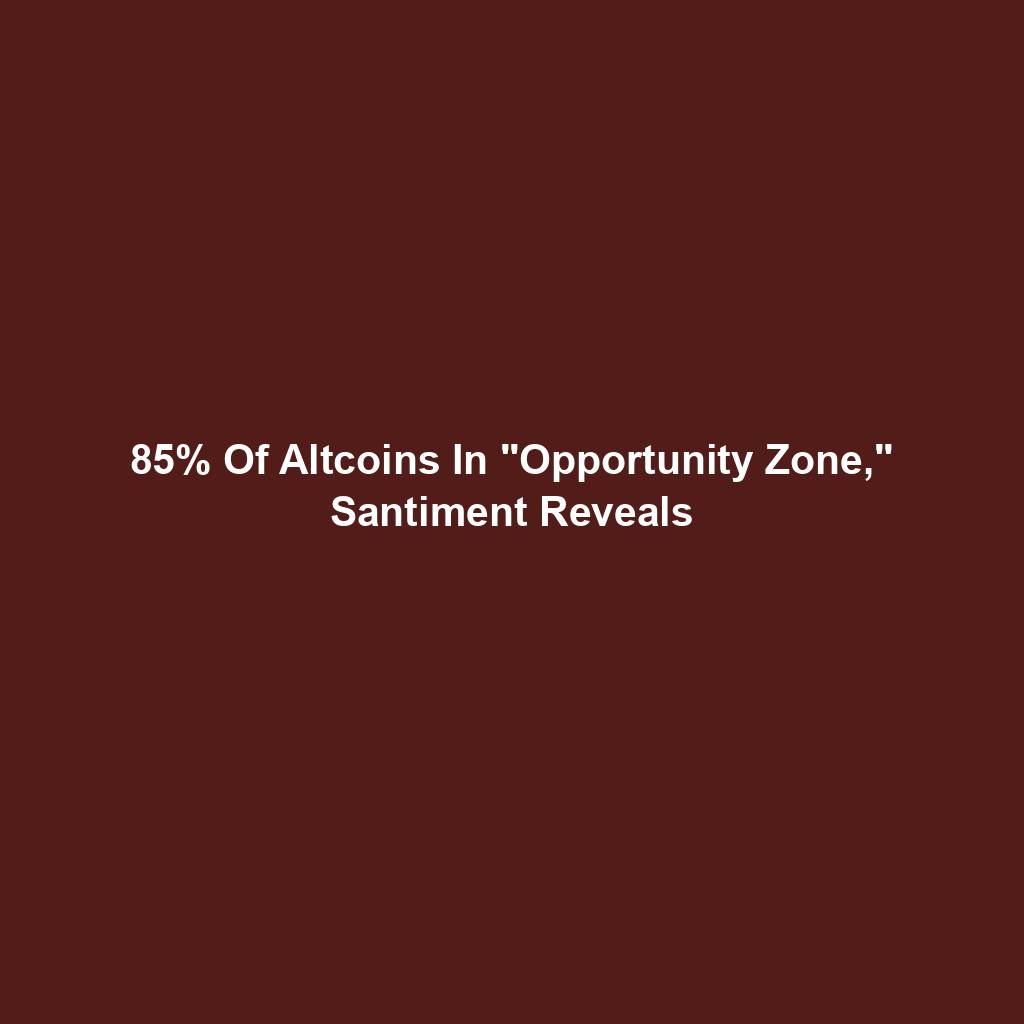
Sam Altman’s Worldcoin eyeing PayPal and OpenAI partnerships
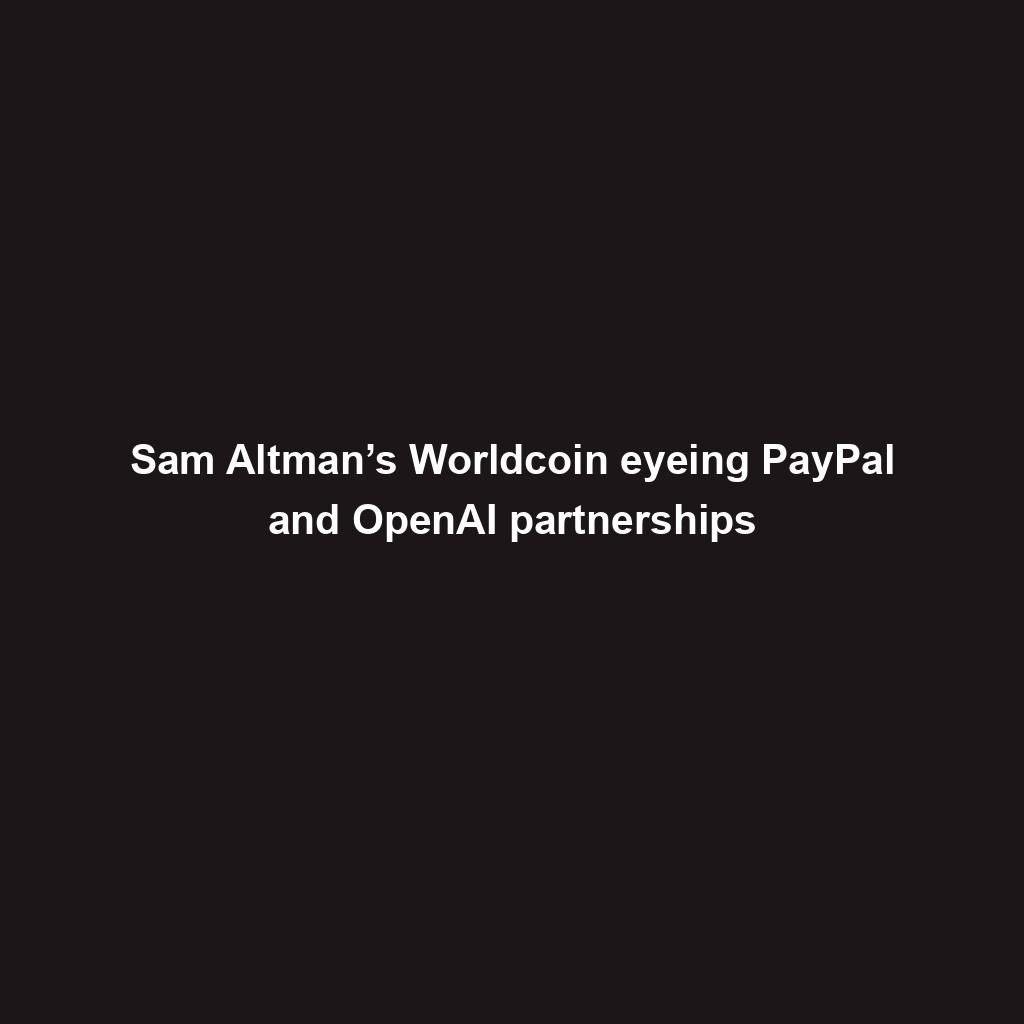
Artificial Intelligence transforms the IT strengthen enjoy
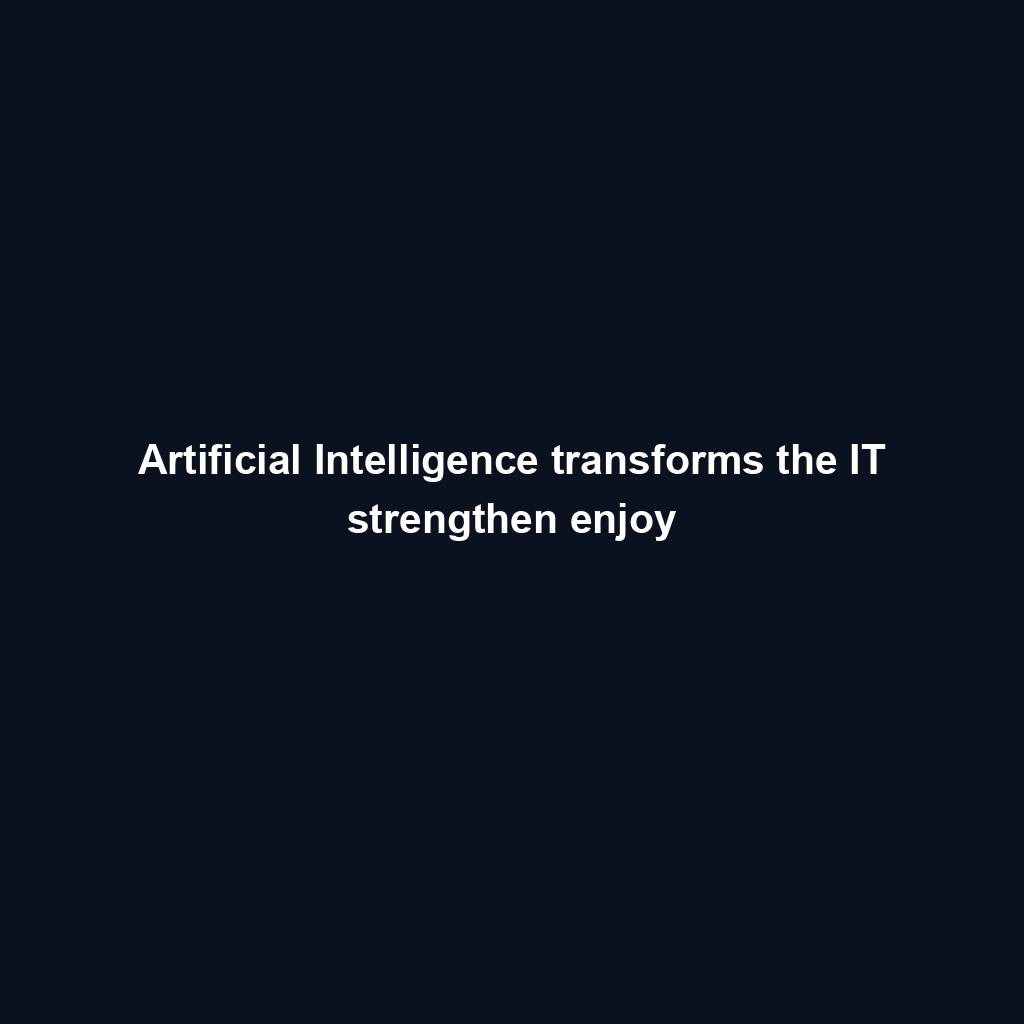
Franklin Templeton tokenizes $380M fund on Polygon and Stellar for P2P transfers
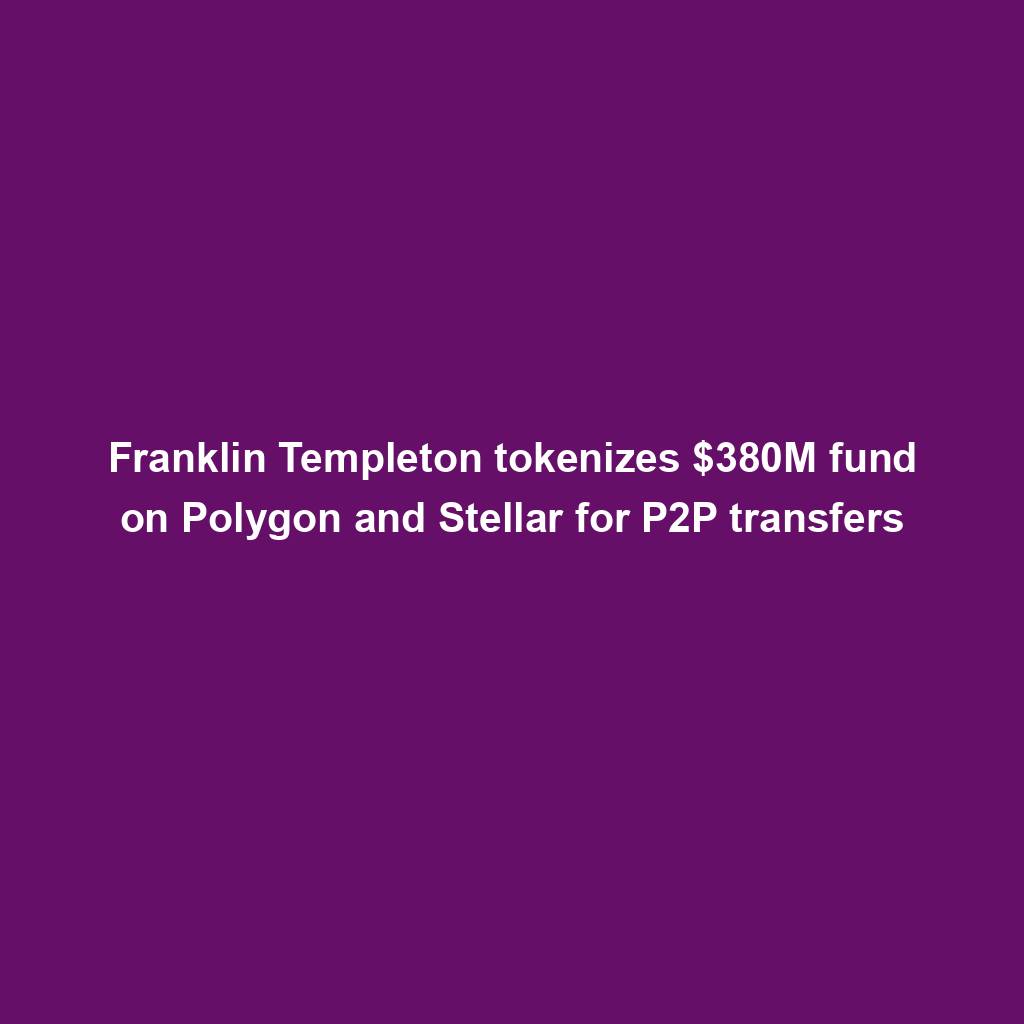
Meta’s letting Xbox, Lenovo, and Asus construct new Quest metaverse {hardware}
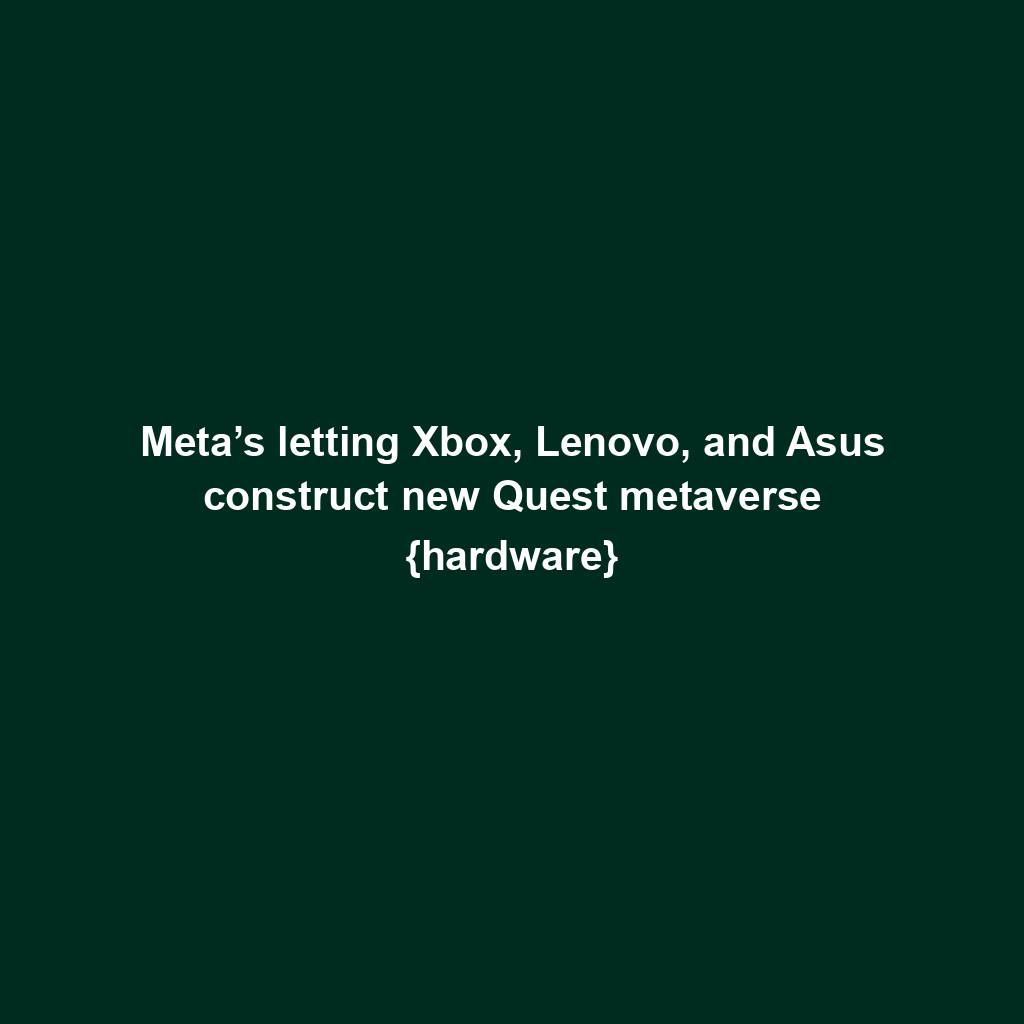
Shiba Inu (SHIB) unveils bold Shibarium plans as Kangamoon steals the display
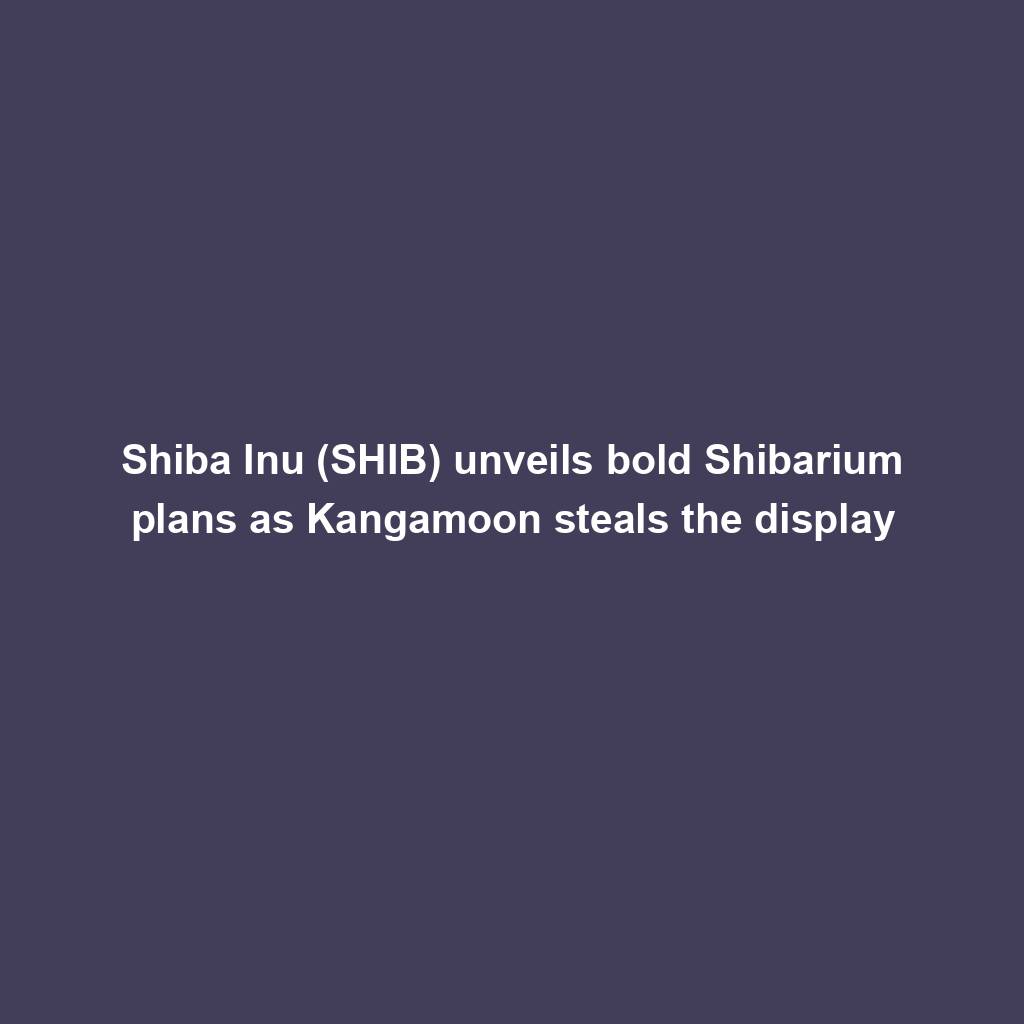